Spatial interpolation using MLP–RBFN hybrid networks
INTERNATIONAL JOURNAL OF GEOGRAPHICAL INFORMATION SCIENCE(2013)
摘要
It is easy for a multi-layered perception MLP to fit a stratified spatial interpolation pattern whose form is close to open surface; while it is easy for a radial basis function network RBFN to fit a pocket radial spatial interpolation pattern whose form is close to closed surface. However, in the real world, the spatial interpolation pattern may consist of stratified and pocket patterns. Neither MLP nor RBFN can fit the pattern easily. To combine their advantages to fit the complex hybrid spatial interpolation patterns, in this article we propose a novel neural network, MLP–RBFN hybrid network MRHN, whose hidden layer contains sigmoid and Gaussian units at the same time. Although there are two kinds of processing units in MRHN, in this study we used the principle of minimizing the error sum of squares to derive the supervised learning rules for all the network parameters. This research took rainfall distribution in Taiwan as a case study. The results show that 1 the prediction error of the testing dataset outside the training dataset demonstrated that MRHN was the most accurate among the three networks, RBFN was the next best, and MLP was the worst; 2 the MLP model seriously underestimated the values of high observed rainfall; 3 over-learning may be a serious shortcoming of using RBFN in spatial interpolation applications; 4 MRHN may have better generalization learning capacity than RBFN in spatial interpolation applications.
更多查看译文
关键词
stratified spatial interpolation pattern,network parameter,rbfn hybrid network,multi-layered perception mlp,spatial interpolation application,mlp model,spatial interpolation pattern,pocket radial spatial interpolation,complex hybrid spatial interpolation,novel neural network,radial basis function network,rainfall,interpolation,spatial analysis,spatial interpolation,artificial neural network
AI 理解论文
溯源树
样例
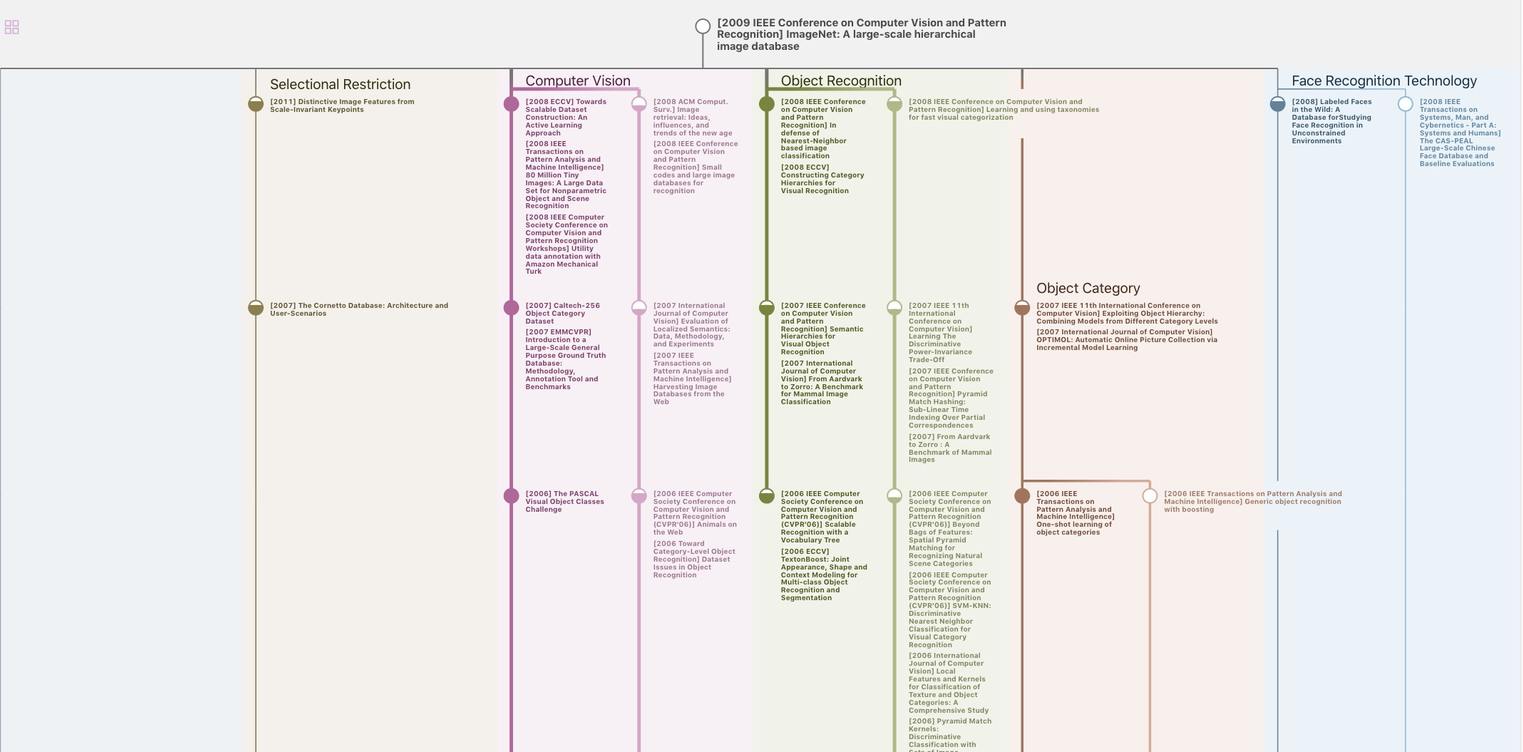
生成溯源树,研究论文发展脉络
Chat Paper
正在生成论文摘要