An ontology-based similarity measure for biomedical data-application to radiology reports.
Journal of Biomedical Informatics(2013)
摘要
Determining similarity between two individual concepts or two sets of concepts extracted from a free text document is important for various aspects of biomedicine, for instance, to find prior clinical reports for a patient that are relevant to the current clinical context. Using simple concept matching techniques, such as lexicon based comparisons, is typically not sufficient to determine an accurate measure of similarity.In this study, we tested an enhancement to the standard document vector cosine similarity model in which ontological parent-child (is-a) relationships are exploited. For a given concept, we define a semantic vector consisting of all parent concepts and their corresponding weights as determined by the shortest distance between the concept and parent after accounting for all possible paths. Similarity between the two concepts is then determined by taking the cosine angle between the two corresponding vectors. To test the improvement over the non-semantic document vector cosine similarity model, we measured the similarity between groups of reports arising from similar clinical contexts, including anatomy and imaging procedure. We further applied the similarity metrics within a k-nearest-neighbor (k-NN) algorithm to classify reports based on their anatomical and procedure based groups. 2150 production CT radiology reports (952 abdomen reports and 1128 neuro reports) were used in testing with SNOMED CT, restricted to Body structure, Clinical finding and Procedure branches, as the reference ontology.The semantic algorithm preferentially increased the intra-class similarity over the inter-class similarity, with a 0.07 and 0.08 mean increase in the neuro-neuro and abdomen-abdomen pairs versus a 0.04 mean increase in the neuro-abdomen pairs. Using leave-one-out cross-validation in which each document was iteratively used as a test sample while excluding it from the training data, the k-NN based classification accuracy was shown in all cases to be consistently higher with the semantics based measure compared with the non-semantic case. Moreover, the accuracy remained steady even as k value was increased - for the two anatomy related classes accuracy for k=41 was 93.1% with semantics compared to 86.7% without semantics. Similarly, for the eight imaging procedures related classes, accuracy (for k=41) with semantics was 63.8% compared to 60.2% without semantics. At the same k, accuracy improved significantly to 82.8% and 77.4% respectively when procedures were logically grouped together into four classes (such as ignoring contrast information in the imaging procedure description). Similar results were seen at other k-values.The addition of semantic context into the document vector space model improves the ability of the cosine similarity to differentiate between radiology reports of different anatomical and image procedure-based classes. This effect can be leveraged for document classification tasks, which suggests its potential applicability for biomedical information retrieval.
更多查看译文
关键词
document classification task,mean increase,intra-class similarity,radiology information systems,document vector space model,radiology informatics,natural language processing,biomedical data,semantics,semantic similarity,systematized nomenclature of medicine,document similarity comparison,ontology-based similarity measure,free text document,cosine similarity,similarity model,inter-class similarity,similarity metrics,imaging procedure
AI 理解论文
溯源树
样例
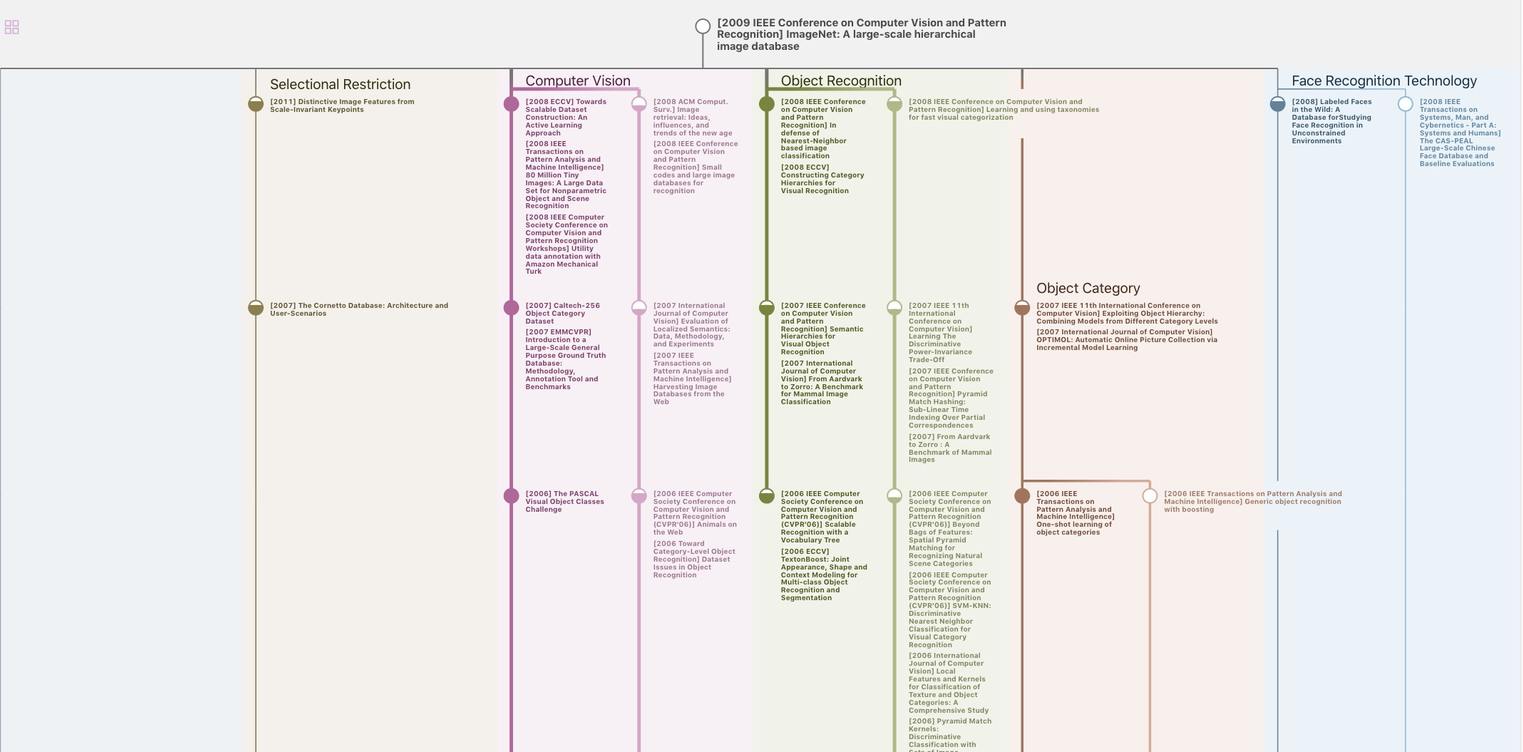
生成溯源树,研究论文发展脉络
Chat Paper
正在生成论文摘要