Correlation clustering with stochastic labellings
SIMBAD(2013)
摘要
Correlation clustering is the problem of finding a crisp partition of the vertices of a correlation graph in such a way as to minimize the disagreements in the cluster assignments. In this paper, we discuss a relaxation to the original problem setting which allows probabilistic assignments of vertices to labels. By so doing, overlapping clusters can be captured. We also show that a known optimization heuristic can be applied to the problem formulation, but with the automatic selection of the number of classes. Additionally, we propose a simple way of building an ensemble of agreement functions sampled from a reproducing kernel Hilbert space, which allows to apply correlation clustering without the empirical estimation of pairwise correlation values.
更多查看译文
关键词
pairwise correlation value,correlation graph,cluster assignment,automatic selection,correlation clustering,stochastic labellings,crisp partition,problem formulation,original problem setting,empirical estimation,agreement function
AI 理解论文
溯源树
样例
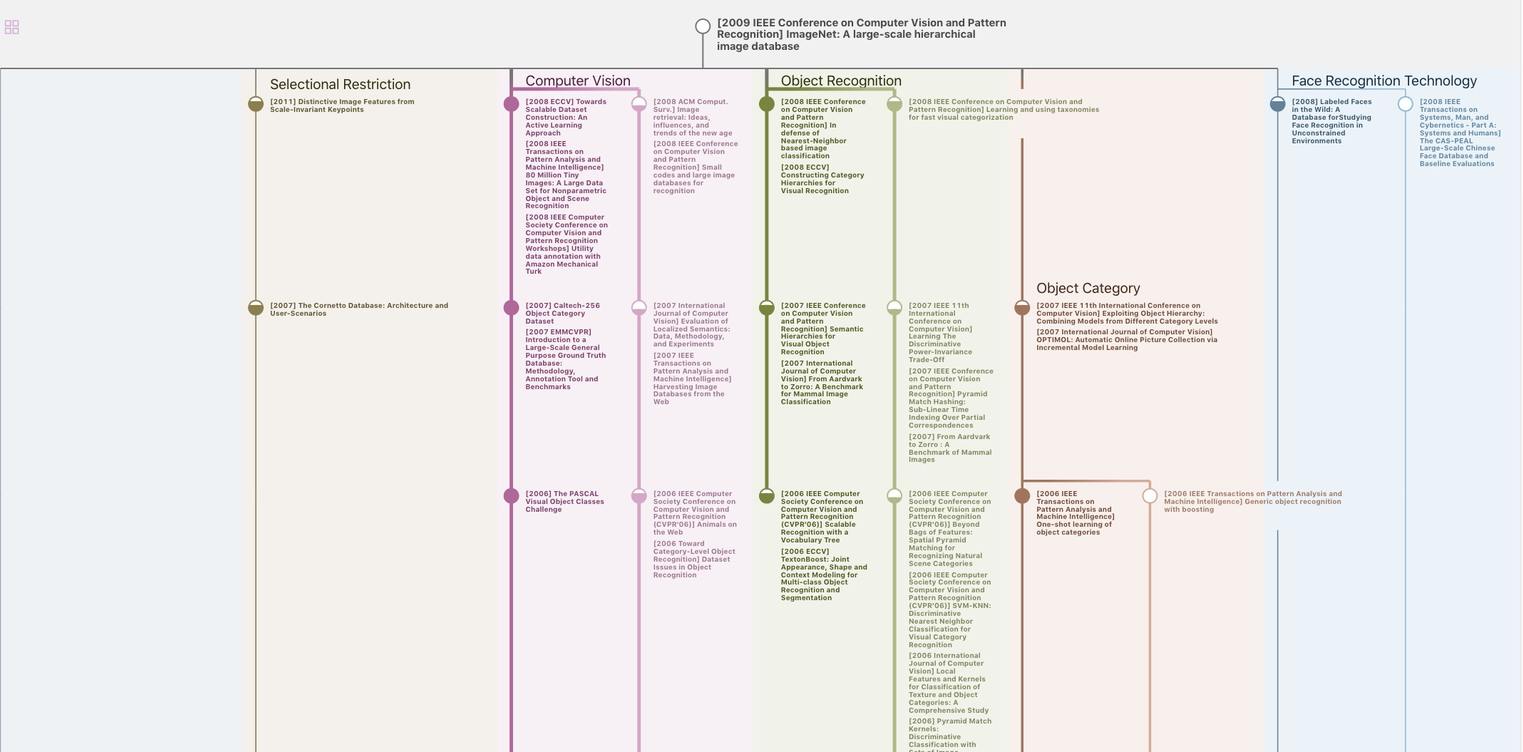
生成溯源树,研究论文发展脉络
Chat Paper
正在生成论文摘要