Spatial Inference Machines
Computer Vision and Pattern Recognition(2013)
摘要
This paper addresses the problem of semantic segmentation of 3D point clouds. We extend the inference machines framework of Ross et al. by adding spatial factors that model mid-range and long-range dependencies inherent in the data. The new model is able to account for semantic spatial context. During training, our method automatically isolates and retains factors modelling spatial dependencies between variables that are relevant for achieving higher prediction accuracy. We evaluate the proposed method by using it to predict 17-category semantic segmentations on sets of stitched Kinect scans. Experimental results show that the spatial dependencies learned by our method significantly improve the accuracy of segmentation. They also show that our method outperforms the existing segmentation technique of Koppula et al.
更多查看译文
关键词
17-category semantic segmentation,higher prediction accuracy,existing segmentation technique,spatial dependency,semantic segmentation,spatial factor,new model,spatial inference machines,semantic spatial context,image segmentation,predictive models,learning artificial intelligence,computer vision,semantics,graphical models,computational modeling,spatial reasoning
AI 理解论文
溯源树
样例
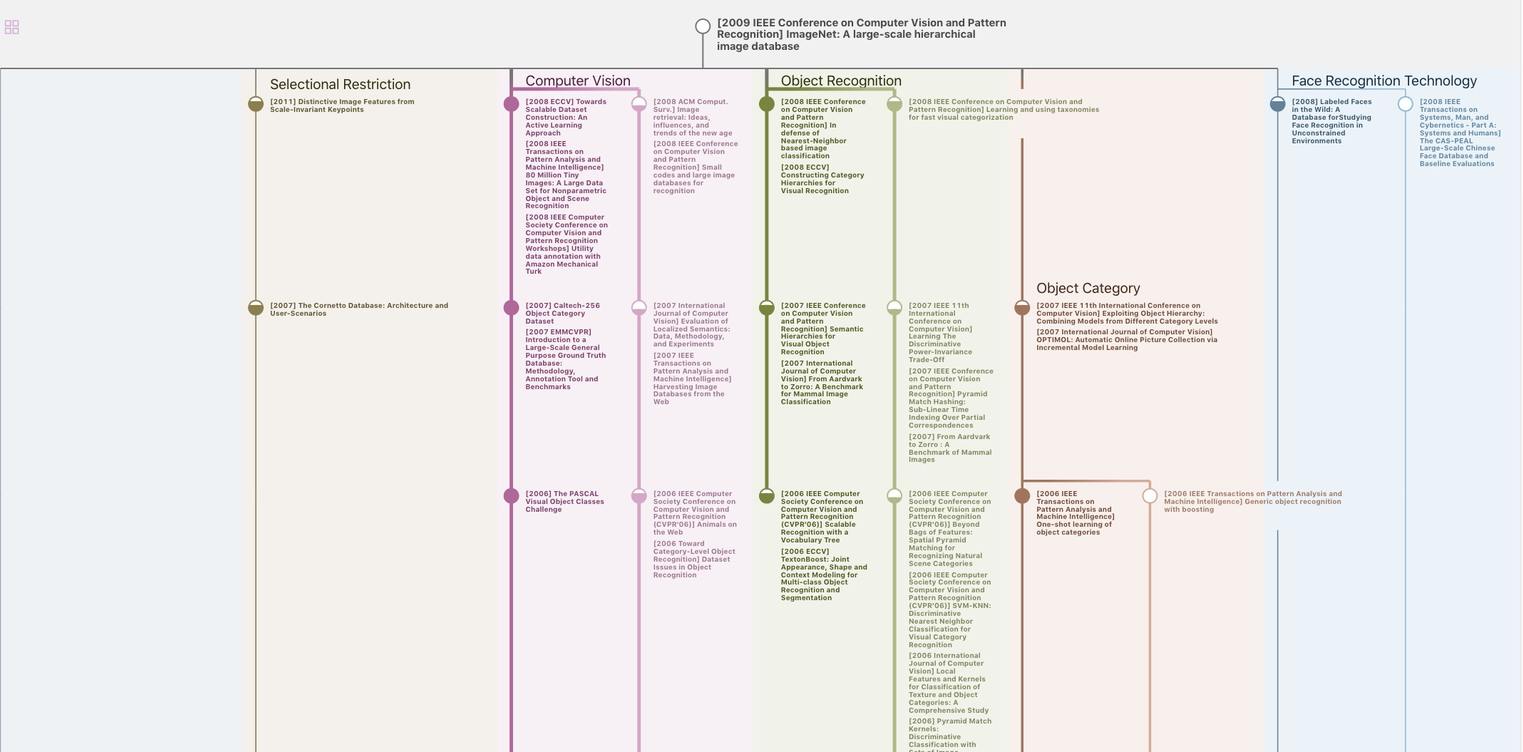
生成溯源树,研究论文发展脉络
Chat Paper
正在生成论文摘要