Constraints as Features
CVPR '13 Proceedings of the 2013 IEEE Conference on Computer Vision and Pattern Recognition(2013)
摘要
In this paper, we introduce a new approach to constrained clustering which treats the constraints as features. Our method augments the original feature space with additional dimensions, each of which derived from a given Cannot-link constraints. The specified Cannot-link pair gets extreme coordinates values, and the rest of the points get coordinate values that express their spatial influence from the specified constrained pair. After augmenting all the new features, a standard unconstrained clustering algorithm can be performed, like k-means or spectral clustering. We demonstrate the efficacy of our method for active semi-supervised learning applied to image segmentation and compare it to alternative methods. We also evaluate the performance of our method on the four most commonly evaluated datasets from the UCI machine learning repository.
更多查看译文
关键词
image segmentation,learning (artificial intelligence),pattern clustering,Cannot-link constraints,Cannot-link pair,UCI machine learning repository,active semisupervised learning,constrained clustering,extreme coordinate values,feature space,image segmentation,k-means clustering,spectral clustering,standard unconstrained clustering algorithm,clustering,image segmentation,machine learning,semi-supervised
AI 理解论文
溯源树
样例
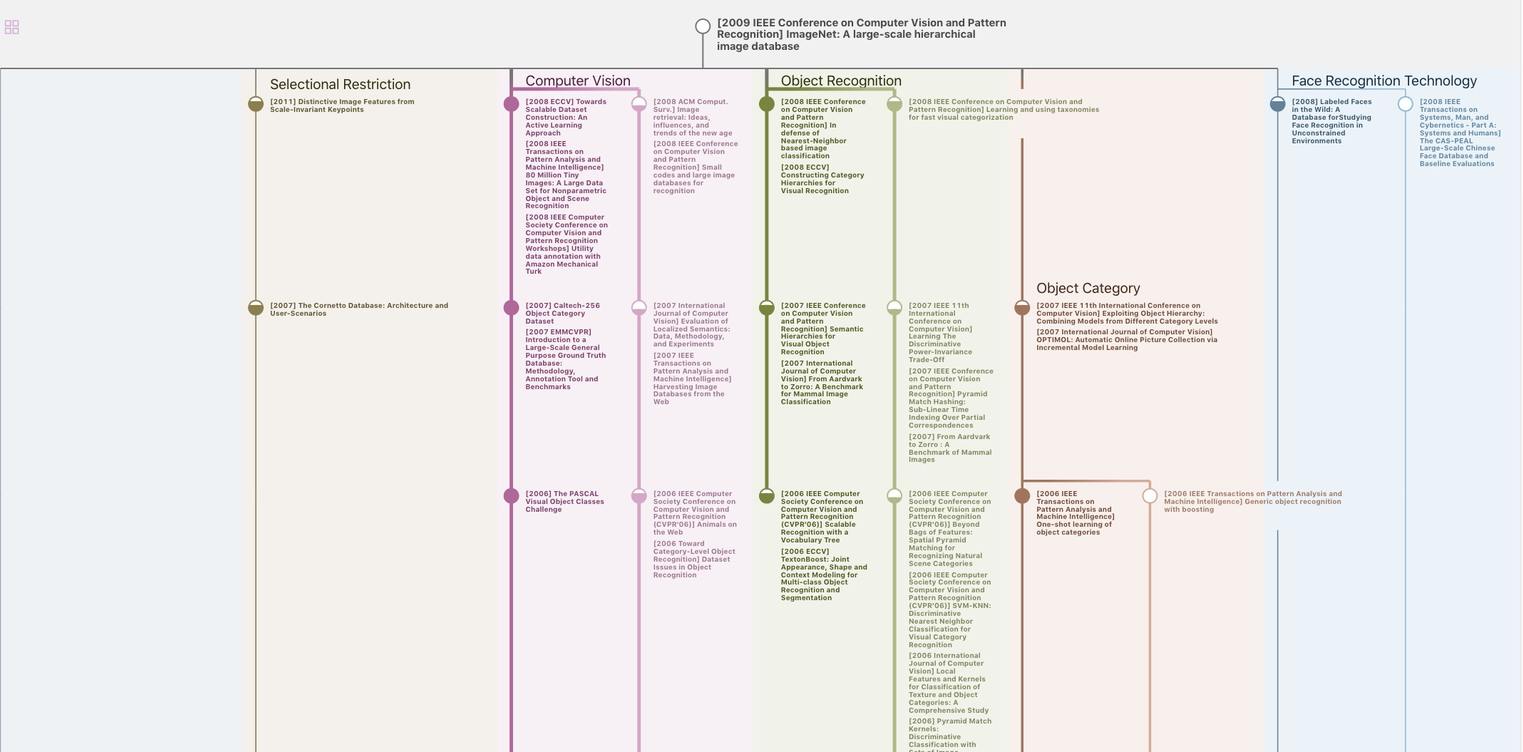
生成溯源树,研究论文发展脉络
Chat Paper
正在生成论文摘要