Towards Payment-Bound Analysis in Cloud Systems with Task-Prediction Errors
Cloud Computing(2013)
摘要
In modern cloud systems, how to optimize user service level based on virtual resources customized on demand is a critical issue. In this paper, we comprehensively analyze the payment bound under a cloud model with virtual machines (VMs), by taking into account that task's workload may be predicted with errors. The analysis is based on an optimized resource allocation algorithm with polynomial time complexity. We theoretically derive the upper bound of task payment based on a particular margin of workload prediction-error. We also extend the payment-minimization algorithm to adapt to the dynamic changes of host availability over time, and perform the evaluation by a real-cluster environment with 56 VMs deployed. Experiments confirm the correctness of our theoretical inference, and show that our payment-minimization solution can keep 95% of user payments below 1.15 times as large as the theoretical values of the ideal payment with hypothetically accurate information. The ratio for the rest user payments can be limited to about 1.5 at the worst case.
更多查看译文
关键词
payment-minimization algorithm,ideal payment,task workload,optimized resource allocation algorithm,real-cluster environment,user service level,user service level optimization,cloud model,analysis of optimization,cloud systems,virtual machines,user payment,resource allocation,task-prediction errors,rest user payment,computational complexity,payment bound,virtual resources,workload prediction-error,modern cloud system,vm,financial management,cloud computing,payment-bound analysis,payment-minimization solution,towards payment-bound analysis,convex optimization,polynomial time complexity,task payment
AI 理解论文
溯源树
样例
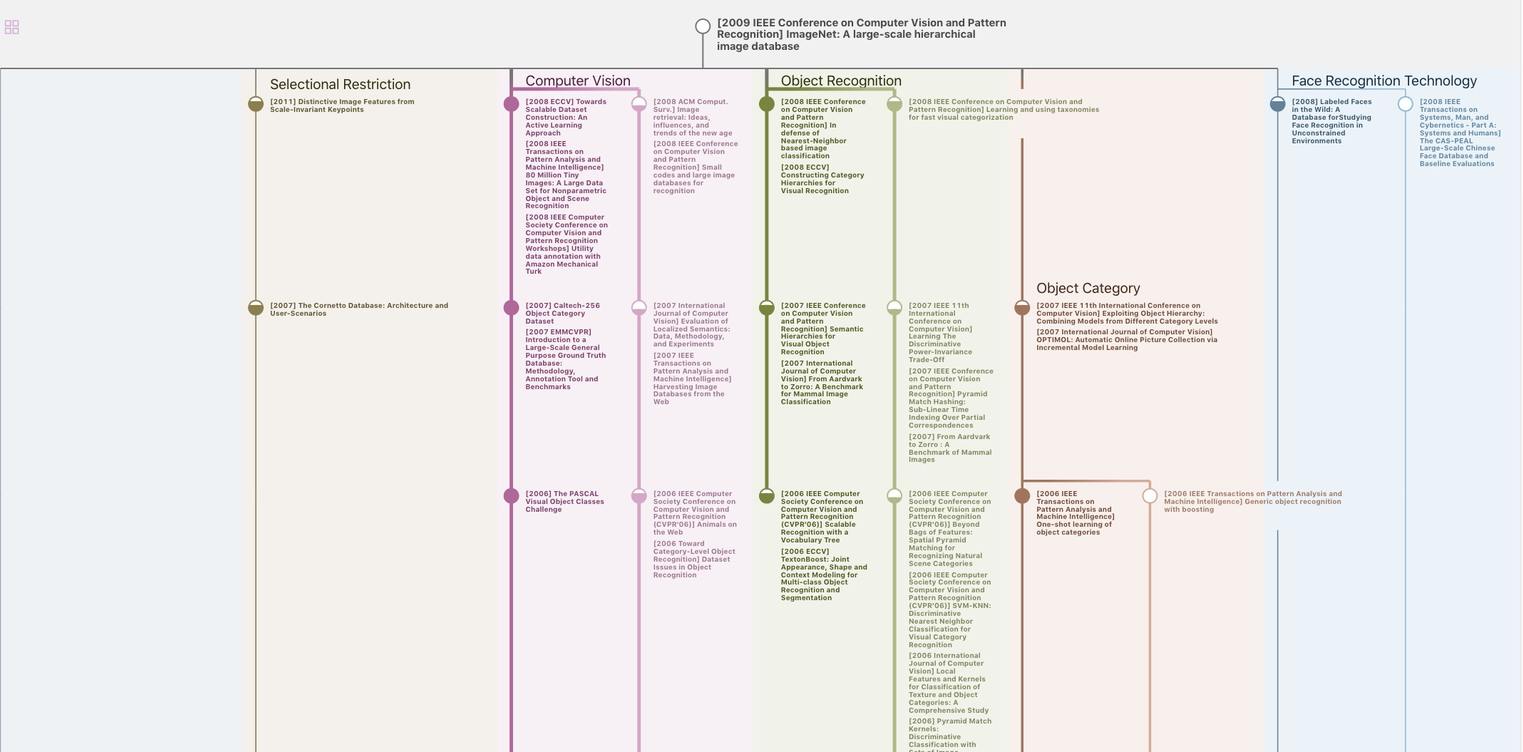
生成溯源树,研究论文发展脉络
Chat Paper
正在生成论文摘要