Online behavior change detection in computer games
Expert Systems with Applications(2013)
摘要
Player Modelling has been receiving much attention from the game community in the recent years. The ability to build accurate models of player behavior can be useful in many aspects of a game. One important aspect is the tracking of a player's behavior along time, informing every time a change is perceived. This way, the game Artificial Intelligence can adapt itself to better respond to this new behavior. In order to build models of player behavior, researchers frequently resort to Machine Learning techniques. Such methods work on previously recorded game metrics representing player's interactions with the game environment. However, if the player changes styles over time, the constructed models get out of date. In order to address this drawback, this work proposes the use of and incremental learning technique to track a player's behavior during his/her interaction with the game environment. Our approach attempts to automatically detect the moments in time when the player changes behavior. We apply a change detection technique from the area of Data Stream Mining that is based on incremental clustering and novelty detection. We also propose three modifications to the original technique, in order to formalize change detection, improve detection rate and reduce detection delay. Simulations were performed considering data produced by the Unreal Tournament game, showing the applicability of the method to online tracking of a player's behavior and informing whenever behavior changes occur.
更多查看译文
关键词
computer game,game artificial intelligence,unreal tournament game,game community,player changes style,player changes behavior,behavior change,game environment,player behavior,online behavior change detection,game metrics,new behavior,data stream mining
AI 理解论文
溯源树
样例
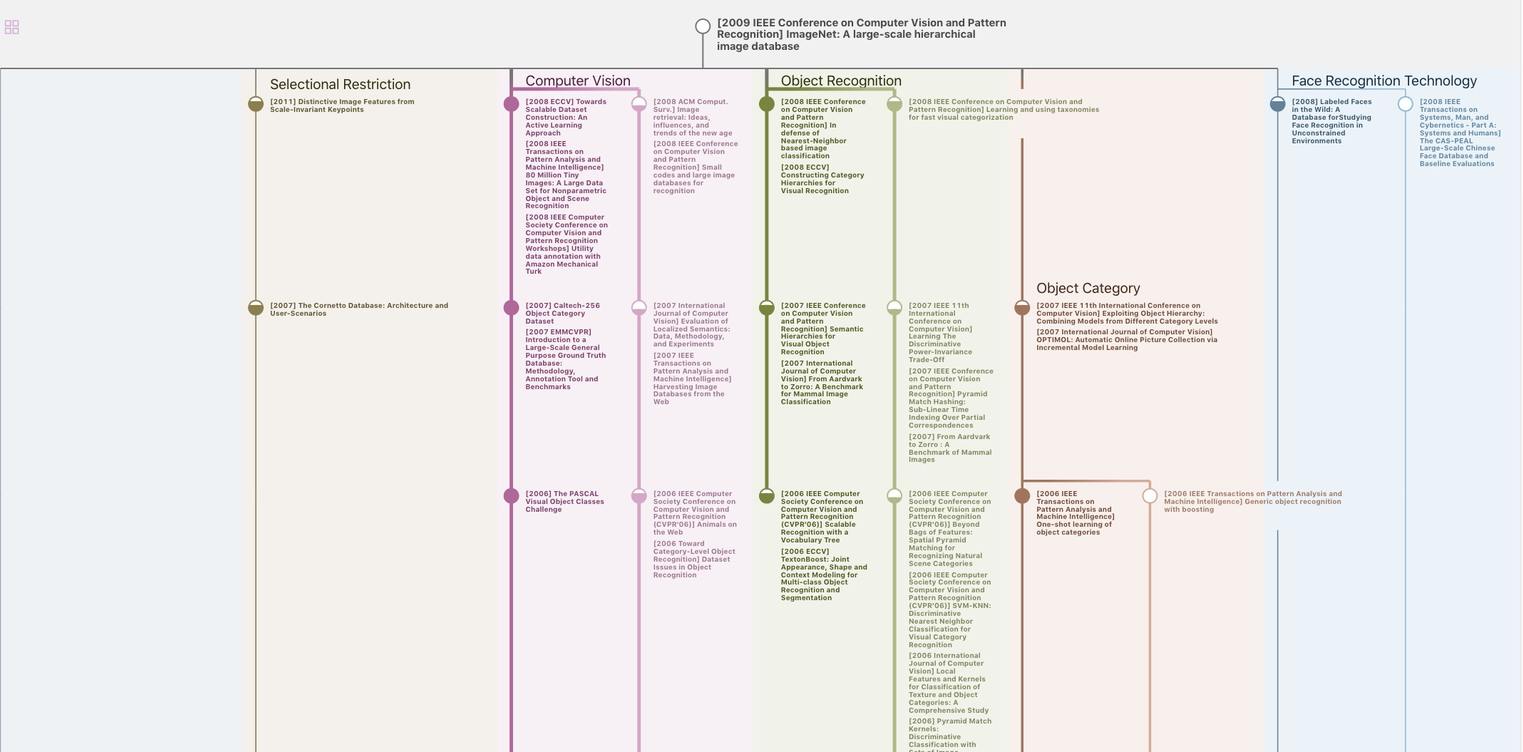
生成溯源树,研究论文发展脉络
Chat Paper
正在生成论文摘要