Robust minimum information loss estimation
Computational Statistics & Data Analysis(2013)
摘要
Two robust estimators of a matrix-valued location parameter are introduced and discussed. Each is the average of the members of a subsample–typically of covariance or cross-spectrum matrices–with the subsample chosen to minimize a function of its average. In one case this function is the Kullback–Leibler discrimination information loss incurred when the subsample is summarized by its average; in the other it is the determinant, subject to a certain side condition. For each, the authors give an efficient computing algorithm, and show that the estimator has, asymptotically, the maximum possible breakdown point. The main motivation is the need for efficient and robust estimation of cross-spectrum matrices, and they present a case study in which the data points originate as multichannel electroencephalogram recordings but are then summarized by the corresponding sample cross-spectrum matrices.
更多查看译文
关键词
Breakdown,Covariance,Cross-spectrum matrix,Electroencephalogram recording,Genetic algorithm,Minimum covariance determinant,Minimum information loss determinant estimate,Spectrum,Trimmed minimum information loss estimate
AI 理解论文
溯源树
样例
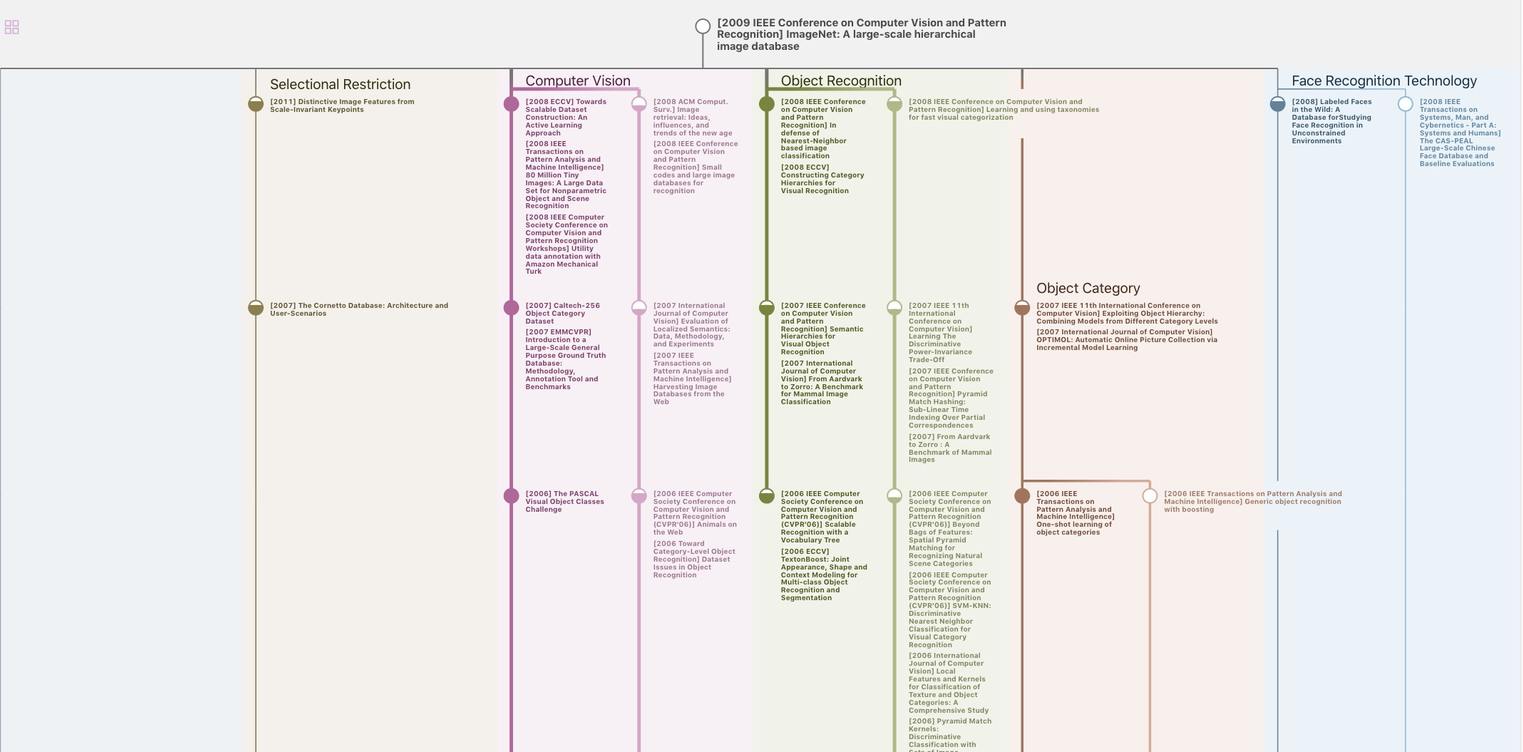
生成溯源树,研究论文发展脉络
Chat Paper
正在生成论文摘要