Self-Adjusting Models for Semi-supervised Learning in Partially Observed Settings
Data Mining(2012)
摘要
We present a new direction for semi-supervised learning where self-adjusting generative models replace fixed ones and unlabeled data can potentially improve learning even when labeled data is only partially-observed. We model each class data by a mixture model and use a hierarchical Dirichlet process (HDP) to model observed as well as unobserved classes. We extend the standard HDP model to accommodate unlabeled samples and introduce a new sharing strategy, within the context of Gaussian mixture models, that restricts sharing with covariance matrices while leaving the mean vectors free. Our research is mainly driven by real-world applications with evolving data-generating mechanisms where obtaining a fully-observed labeled data set is impractical. We demonstrate the feasibility of the proposed approach for semi-supervised learning in two such applications.
更多查看译文
关键词
Gaussian processes,covariance matrices,data models,learning (artificial intelligence),Gaussian mixture models,HDP,covariance matrices,data model,data-generating mechanisms,hierarchical Dirichlet process,mean vectors,partially observed settings,real-world applications,self-adjusting generative models,semisupervised learning,sharing strategy,standard HDP model,unlabeled data,unobserved classes,class discovery,gaussian mixture model,hierarchical dirichlet process,partially-observed data sets,semi-supervised learning
AI 理解论文
溯源树
样例
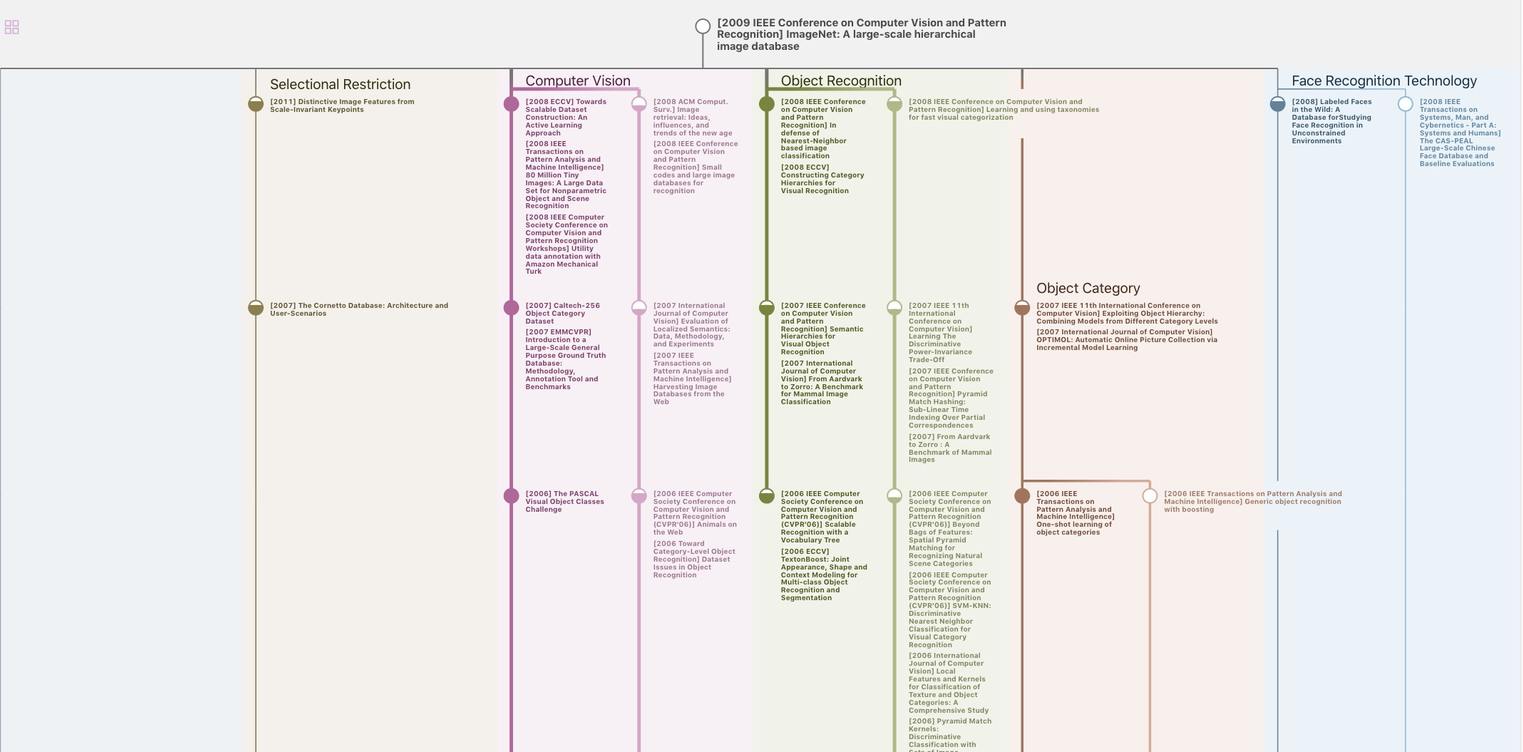
生成溯源树,研究论文发展脉络
Chat Paper
正在生成论文摘要