Dimensional Testing for Multi-step Similarity Search
Data Mining(2012)
摘要
In data mining applications such as subspace clustering or feature selection, changes to the underlying feature set can require the reconstruction of search indices to support fundamental data mining tasks. For such situations, multi-step search approaches have been proposed that can accommodate changes in the underlying similarity measure without the need to rebuild the index. In this paper, we present a heuristic multi-step search algorithm that utilizes a measure of intrinsic dimension, the generalized expansion dimension (GED), as the basis of its search termination condition. Compared to the current state-of-the-art method, experimental results show that our heuristic approach is able to obtain significant improvements in both the number of candidates and the running time, while losing very little in the accuracy of the query results.
更多查看译文
关键词
data mining,pattern clustering,query processing,GED,data mining,dimensional testing,feature selection,feature set,generalized expansion dimension,heuristic multistep search algorithm,intrinsic dimension,multistep similarity search,query result,search indices,search termination condition,similarity measure,subspace clustering,Similarity search,adaptive similarity,intrinsic dimensionality,kNN,multi-step,nearest neighbor
AI 理解论文
溯源树
样例
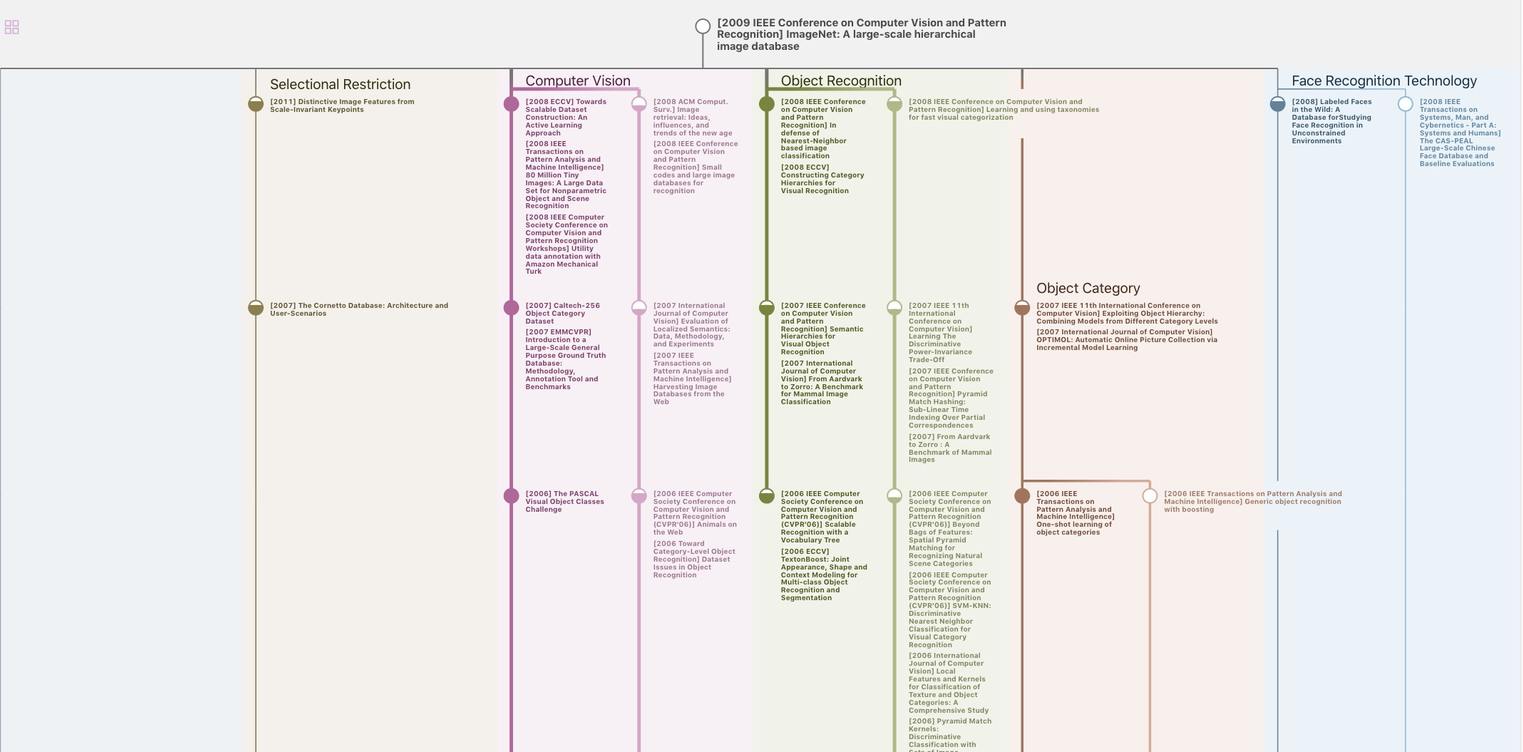
生成溯源树,研究论文发展脉络
Chat Paper
正在生成论文摘要