Differential evolution with local information for neuro-fuzzy systems optimisation
Knowledge-Based Systems(2013)
摘要
This paper proposes a differential evolution with local information (DELI) algorithm for Takagi-Sugeno-Kang-type (TSK-type) neuro-fuzzy systems (NFSs) optimisation. The DELI algorithm uses a modified mutation operation that considers a neighbourhood relationship for each individual to maintain the diversity of the population and to increase the search capability. This paper also proposes an adaptive fuzzy c-means method for determining the number of rules and for identifying suitable initial parameters for the rules. Initially, there are no rules in the NFS model; the rules are automatically generated by the fuzzy measure and the fuzzy c-means method. Until the firing strengths of all of the training patterns satisfy a pre-specified threshold, the process of rule generation is terminated. Subsequently, the DELI algorithm optimises all of the free parameters for NFSs design. To enhance the performance of the DELI algorithm, an adaptive parameter tuning based on the 1/5th rule is used for the tuning scale factor F. The 1/5th rule dynamically adjusts the tuning scale factor in each period to enhance the search capability of the DELI algorithm. Finally, the proposed NFS with DELI model (NFS-DELI) is applied to nonlinear control and prediction problems. The results of this paper demonstrate the effectiveness of the proposed NFS-DELI model.
更多查看译文
关键词
deli model,search capability,deli algorithm,neuro-fuzzy systems optimisation,local information,fuzzy measure,nfs model,tuning scale factor,fuzzy c-means method,adaptive fuzzy c-means method,proposed nfs-deli model,differential evolution,rule dynamically
AI 理解论文
溯源树
样例
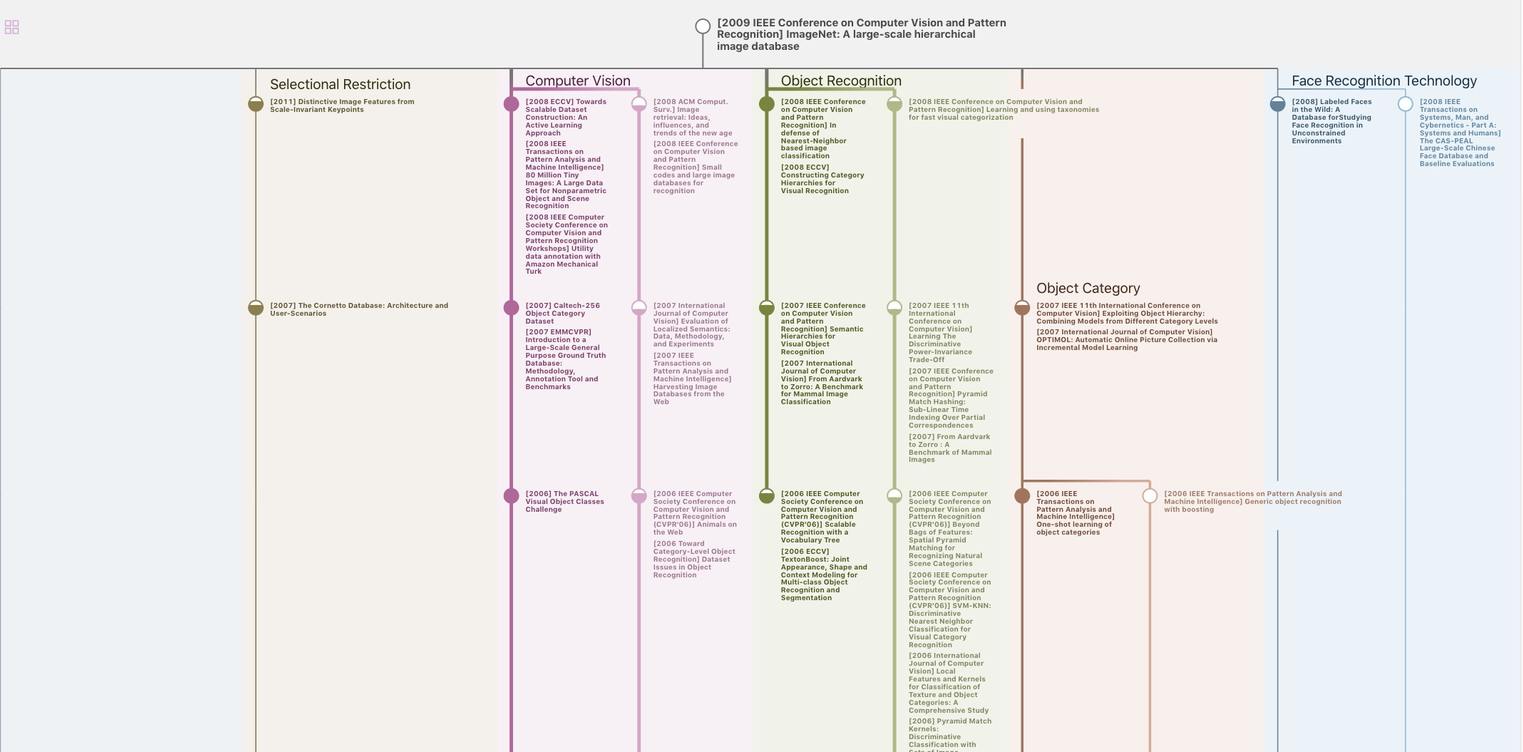
生成溯源树,研究论文发展脉络
Chat Paper
正在生成论文摘要