Genetic programming-based model output statistics for short-range temperature prediction
EvoApplications'13 Proceedings of the 16th European conference on Applications of Evolutionary Computation(2013)
摘要
This paper introduces GP (Genetic Programming) based robust compensation technique for temperature prediction in short-range. MOS (Model Output Statistics) is a statistical technique that corrects the systematic errors of the model. Development of an efficient MOS is very important, but most of MOS are based on the idea of relating model forecasts to observations through a linear regression. Therefore it is hard to manage complex and irregular natures of the prediction. In order to solve the problem, a nonlinear and symbolic regression method using GP is suggested as the first attempt. The purpose of this study is to evaluate the accuracy of the estimation by GP based nonlinear MOS for the 3 days temperatures for Korean regions. This method is then compared to the UM model and shows superior results. The training period of summer in 2007-2009 is used, and the data of 2010 summer is adopted for verification.
更多查看译文
关键词
genetic programming-based model output,genetic programming,short-range temperature prediction,efficient mos,statistical technique,linear regression,nonlinear mos,temperature prediction,um model,model forecast,symbolic regression method,robust compensation technique
AI 理解论文
溯源树
样例
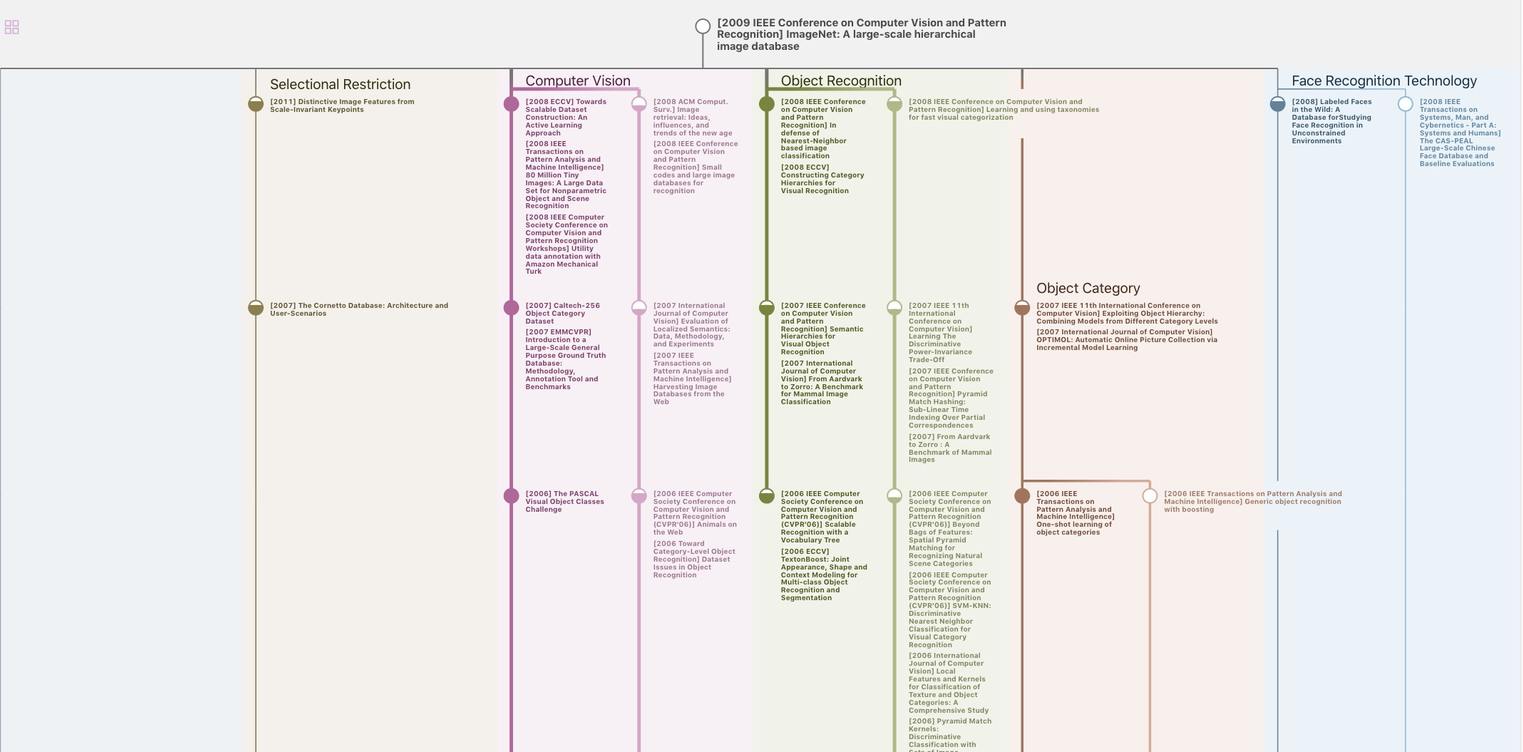
生成溯源树,研究论文发展脉络
Chat Paper
正在生成论文摘要