Active learning on sentiment classification by selecting both words and documents
CLSW(2013)
摘要
Currently, sentiment analysis has become a hot research topic in the natural language processing (NLP) field as it is highly valuable for many real applications.. One basic task in sentiment analysis is sentiment classification which aims to predict the sentiment orientation (positive or negative) of a document. Current approaches to this problem are mainly based on supervised machine learning technologies. The main drawback of such approaches lies in their needs of large amounts of labeled data. How to reduce the annotation cost has become an important issue in sentiment classification. In this study, we propose a novel active learning approach to select both "informative" word and document samples for annotation. Experimental results show that our approach apparently outperforms random selection or uncertainty sampling on documents.
更多查看译文
关键词
hot research topic,sentiment orientation,annotation cost,current approach,basic task,novel active learning approach,document sample,sentiment classification,sentiment analysis,active learning
AI 理解论文
溯源树
样例
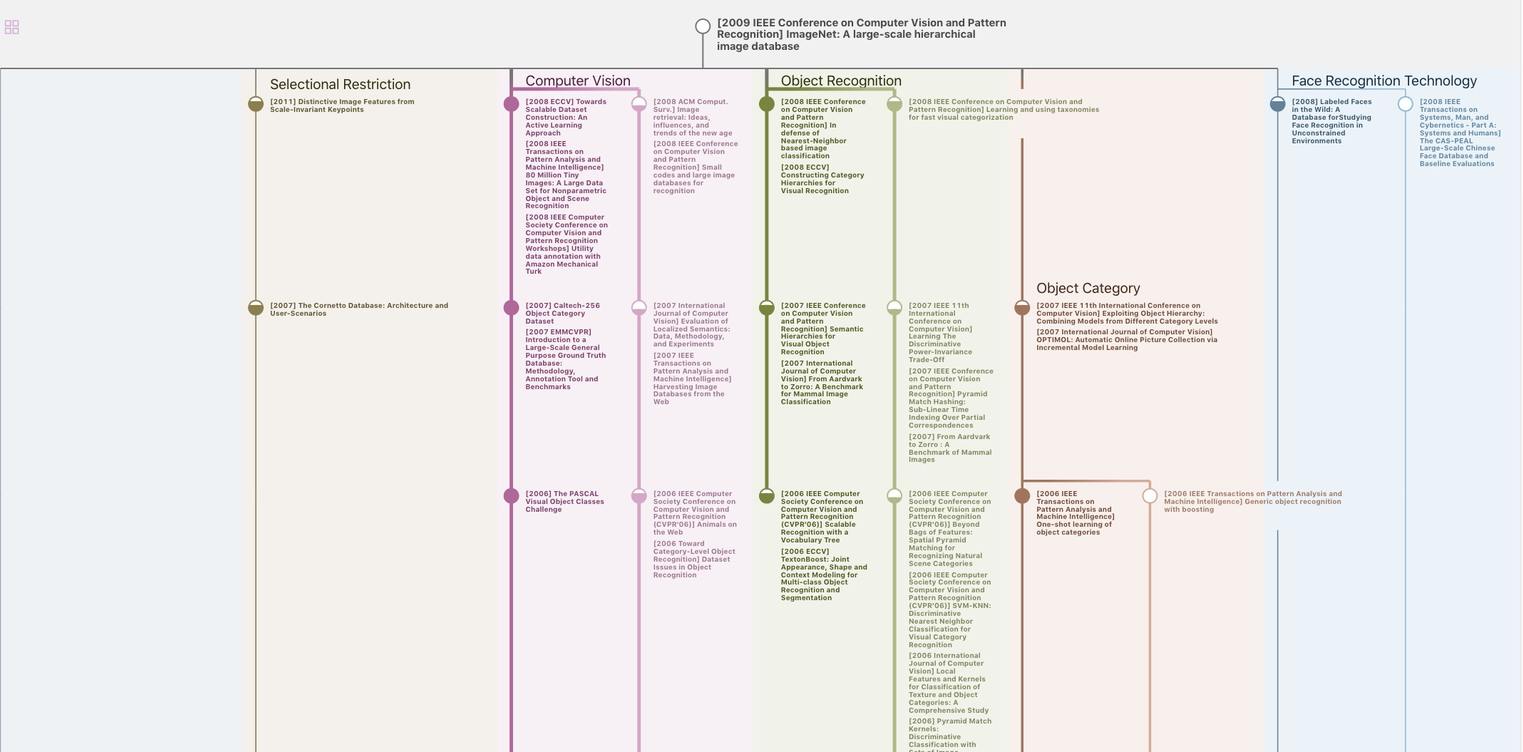
生成溯源树,研究论文发展脉络
Chat Paper
正在生成论文摘要