Information-Theoretic based feature selection for multi-voxel pattern analysis of fMRI data
Brain Informatics(2012)
摘要
Multi-voxel pattern analysis (MVPA) of functional magnetic resonance imaging (fMRI) data is an emerging approach for probing the neural correlates of cognition. MVPA allows cognitive representations and processing to be modeled as distributed patterns of neural activity, which can be used to build a classification model to partition activity patterns according to stimulus conditions. In machine learning, MVPA is a very challenging classification problem because the number of voxels (features) greatly exceeds the number of data instances. Thus, there is a need to select informative voxels before building a classification model. We introduce a feature selection method based on mutual information (MI), which is used to quantify the statistical dependency between features and stimulus conditions. To evaluate the utility of our approach, we employed several linear classification algorithms on a publicly available fMRI data set that has been widely used to benchmark MVPA performance [1]. The computational results suggest that feature selection based on the MI ranking can drastically improve the classification accuracy. Additionally, high-ranked features provide meaningful insights into the functional-anatomic relationship of neural activity and the associated tasks.
更多查看译文
关键词
feature selection,multi-voxel pattern analysis,activity pattern,stimulus condition,challenging classification problem,neural activity,classification model,benchmark mvpa performance,available fmri data set,classification accuracy,linear classification algorithm,data instance
AI 理解论文
溯源树
样例
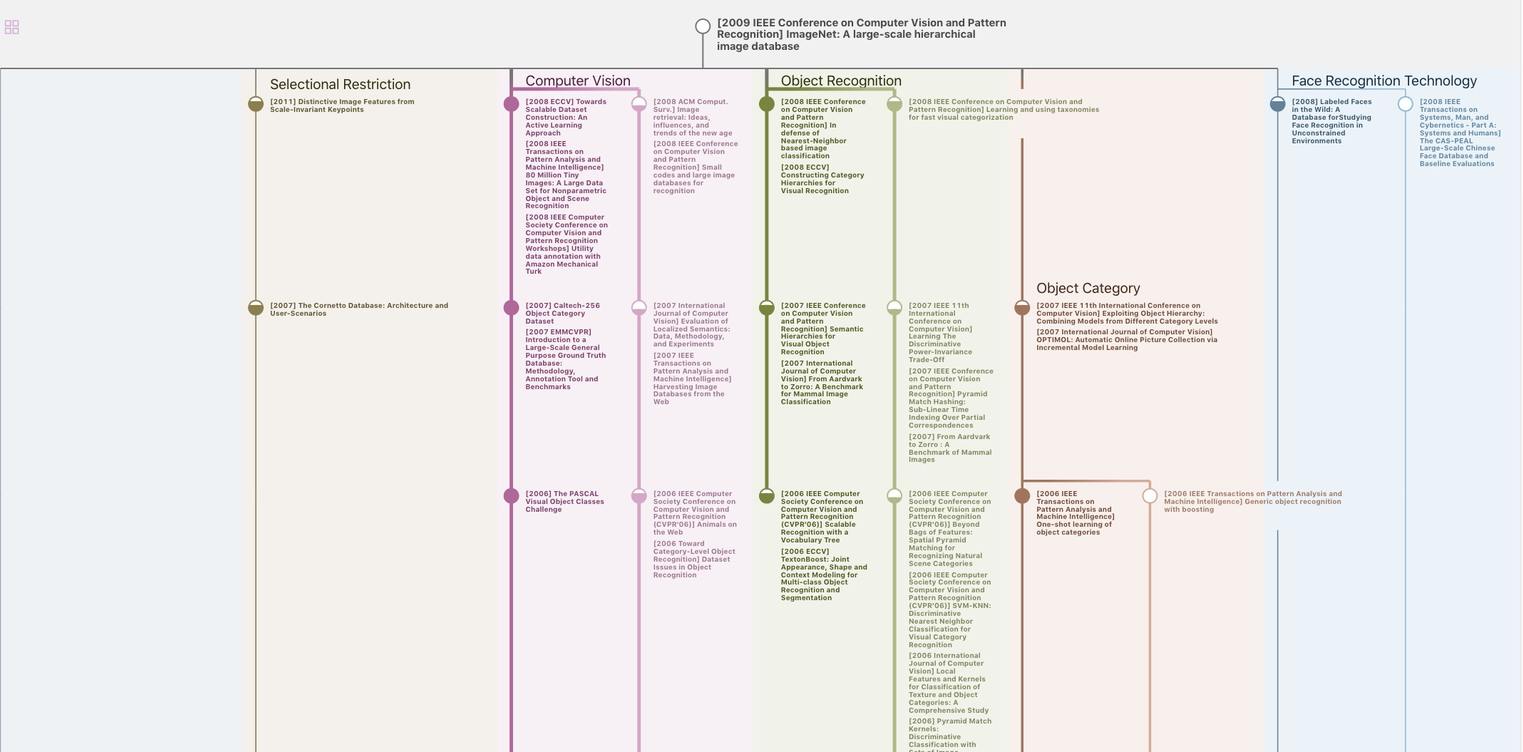
生成溯源树,研究论文发展脉络
Chat Paper
正在生成论文摘要