Score-based methods for learning Markov boundaries by searching in constrained spaces
Data Mining and Knowledge Discovery(2011)
摘要
Within probabilistic classification problems, learning the Markov boundary of the class variable consists in the optimal approach for feature subset selection. In this paper we propose two algorithms that learn the Markov boundary of a selected variable. These algorithms are based on the score+search paradigm for learning Bayesian networks. Both algorithms use standard scoring functions but they perform the search in constrained spaces of class-focused directed acyclic graphs, going through the space by means of operators adapted for the problem. The algorithms have been validated experimentally by using a wide spectrum of databases, and their results show a performance competitive with the state-of-the-art.
更多查看译文
关键词
Feature subset selection,Markov boundary,Bayesian networks,Local learning,Score+search
AI 理解论文
溯源树
样例
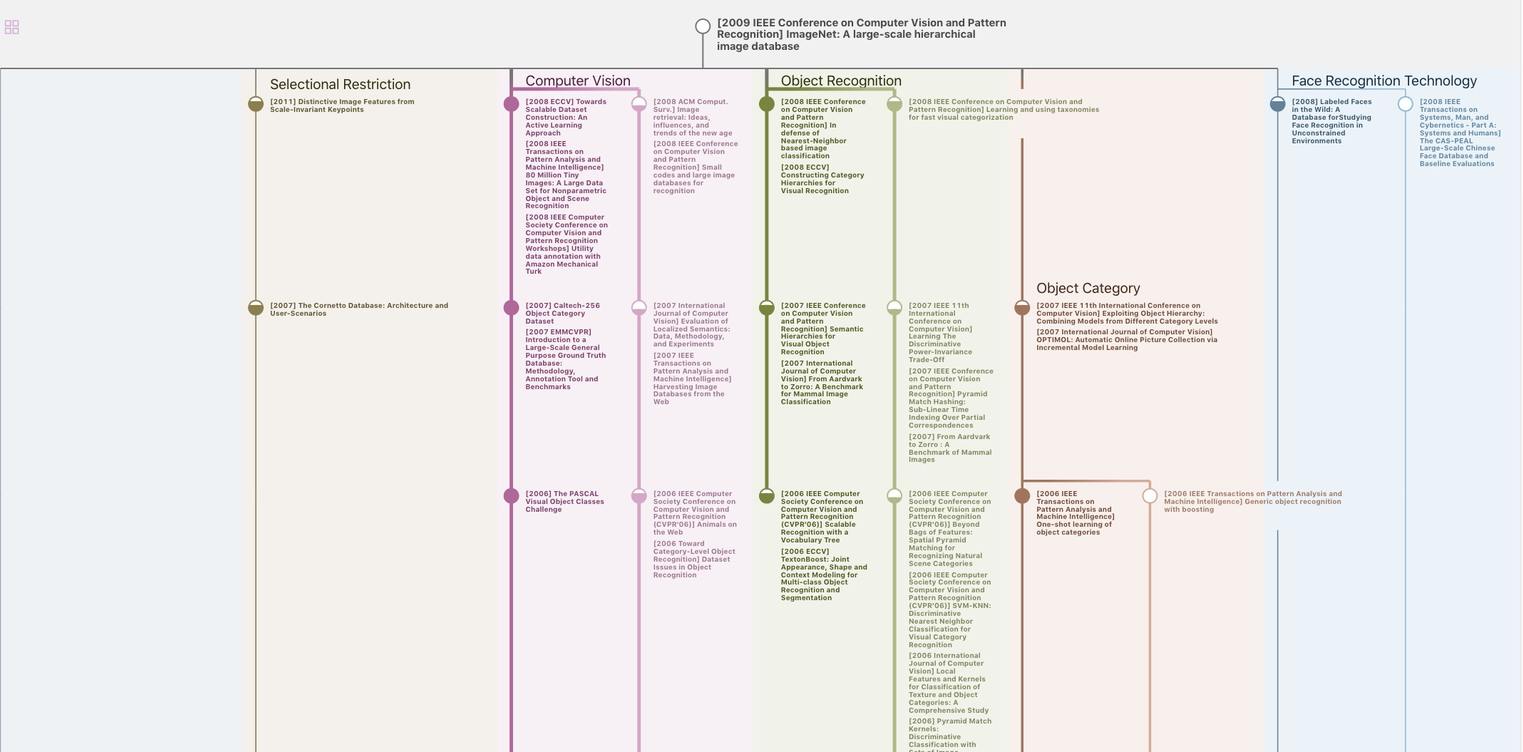
生成溯源树,研究论文发展脉络
Chat Paper
正在生成论文摘要