Relaxed pairwise learned metric for person re-identification
COMPUTER VISION - ECCV 2012, PT VI(2012)
摘要
Matching persons across non-overlapping cameras is a rather challenging task. Thus, successful methods often build on complex feature representations or sophisticated learners. A recent trend to tackle this problem is to use metric learning to find a suitable space for matching samples from different cameras. However, most of these approaches ignore the transition from one camera to the other. In this paper, we propose to learn a metric from pairs of samples from different cameras. In this way, even less sophisticated features describing color and texture information are sufficient for finally getting state-of-the-art classification results. Moreover, once the metric has been learned, only linear projections are necessary at search time, where a simple nearest neighbor classification is performed. The approach is demonstrated on three publicly available datasets of different complexity, where it can be seen that state-of-the-art results can be obtained at much lower computational costs.
更多查看译文
关键词
sophisticated feature,sophisticated learner,challenging task,person re-identification,matching person,different complexity,available datasets,simple nearest neighbor classification,state-of-the-art result,different camera,state-of-the-art classification result
AI 理解论文
溯源树
样例
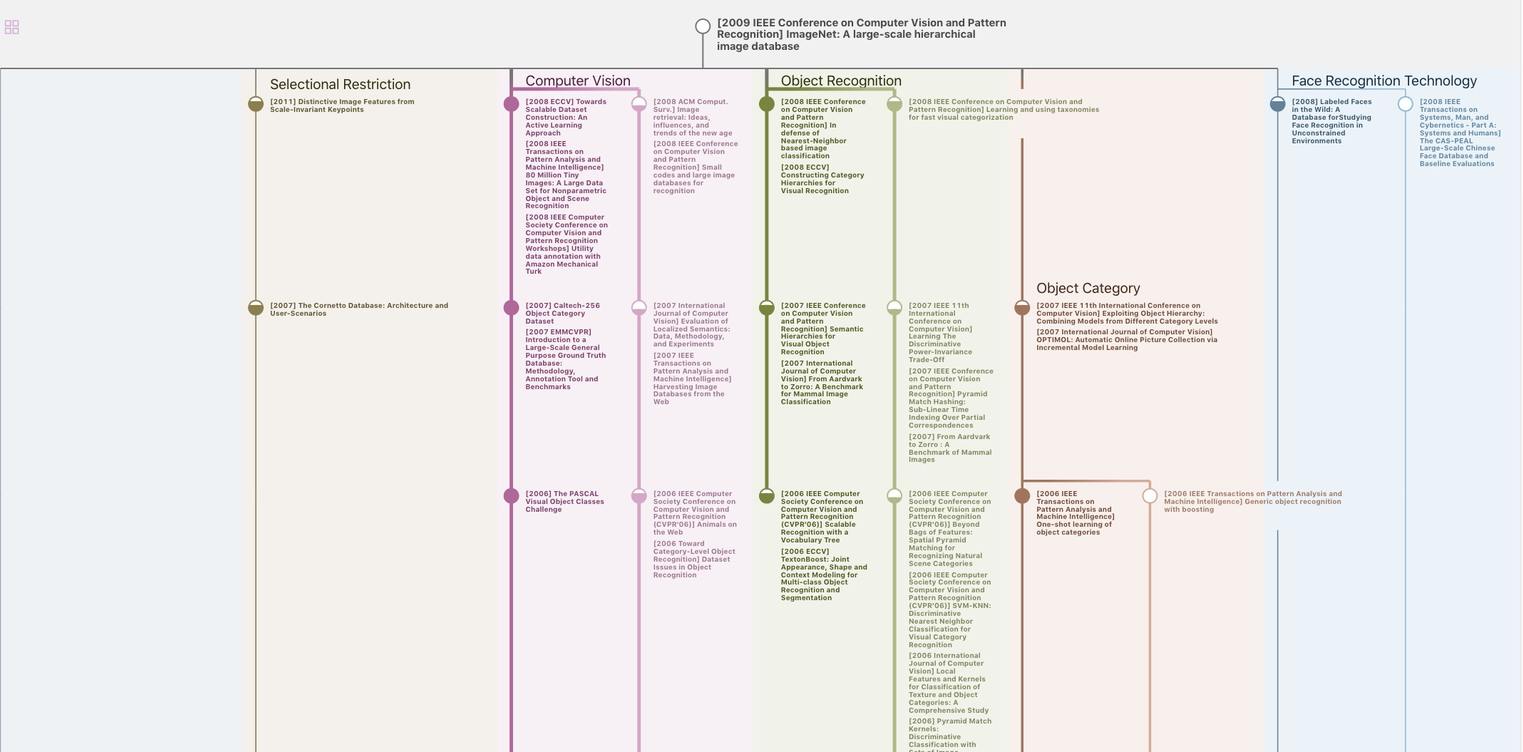
生成溯源树,研究论文发展脉络
Chat Paper
正在生成论文摘要