Content-based collaborative filtering for question difficulty calibration
PRICAI(2012)
摘要
Question calibration especially on difficulty degree is important for supporting Web-based testing and assessment. Currently, Item Response Theory (IRT) has traditionally applied for question difficulty calibration. However, it is tedious and time-consuming to collect sufficient historical response information manually, and computational expensive to calibrate question difficulty especially for large-scale question datasets. In this paper, we propose an effective Content-based Collaborative Filtering (CCF) approach for automatic calibration of question difficulty degree. In the proposed approach, a dataset of questions with available user responses and knowledge features is first gathered. Next, collaborative filtering is used to predict unknown user responses from known responses of questions. With all the responses, the difficulty degree of each question in the dataset is then estimated using IRT. Finally, when a new question is queried, the content-based similarity approach is used to find similar existing questions from the dataset based on the knowledge features for estimating the new question's difficulty degree. In this paper, we will present the proposed CCF approach and its performance evaluation in comparison with other techniques.
更多查看译文
关键词
content-based similarity approach,question difficulty calibration,new question,difficulty degree,question calibration,knowledge feature,content-based collaborative,similar existing question,question difficulty degree,question difficulty,large-scale question datasets,collaborative filtering,item response theory
AI 理解论文
溯源树
样例
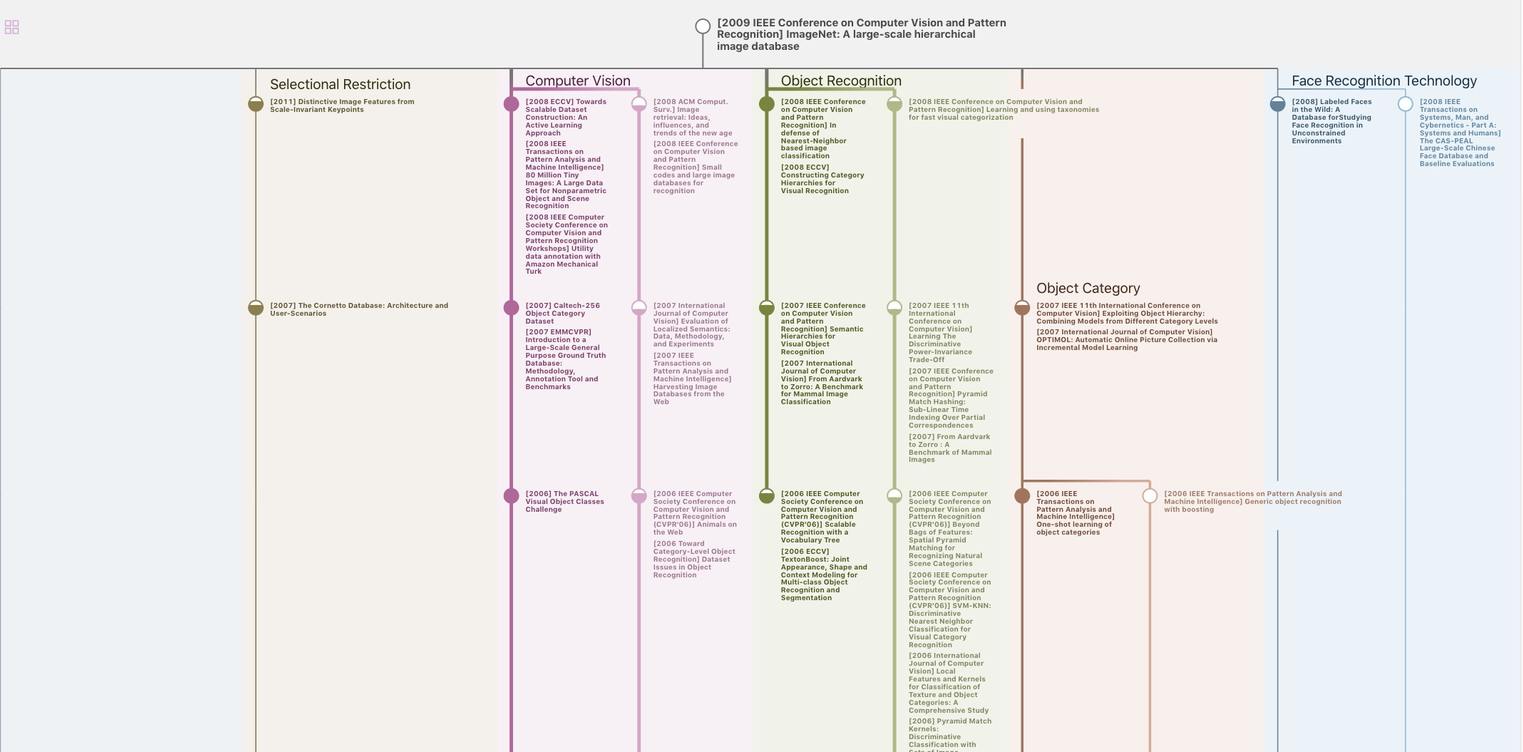
生成溯源树,研究论文发展脉络
Chat Paper
正在生成论文摘要