Regularized extreme learning machine for regression with missing data
Neurocomputing(2013)
摘要
This paper proposes a method which is the advanced modification of the original extreme learning machine with a new tool for solving the missing data problem. It uses a cascade of L"1 penalty (LARS) and L"2 penalty (Tikhonov regularization) on ELM (TROP-ELM) to regularize the matrix computations and hence makes the MSE computation more reliable, and on the other hand, it estimates the expected pairwise distances between samples directly on incomplete data so that it offers the ELM a solution to solve the missing data issues. According to the experiments on five data sets, the method shows its significant advantages: fast computational speed, no parameter need to be tuned and it appears more stable and reliable generalization performance by the two penalties. Moreover, it completes ELM with a new tool to solve missing data problem even when half of the training data are missing as the extreme case.
更多查看译文
关键词
training data,regularized extreme,reliable generalization performance,new tool,original extreme,missing data issue,missing data problem,mse computation,extreme case,incomplete data,ridge regression,lars,missing data,tikhonov regularization
AI 理解论文
溯源树
样例
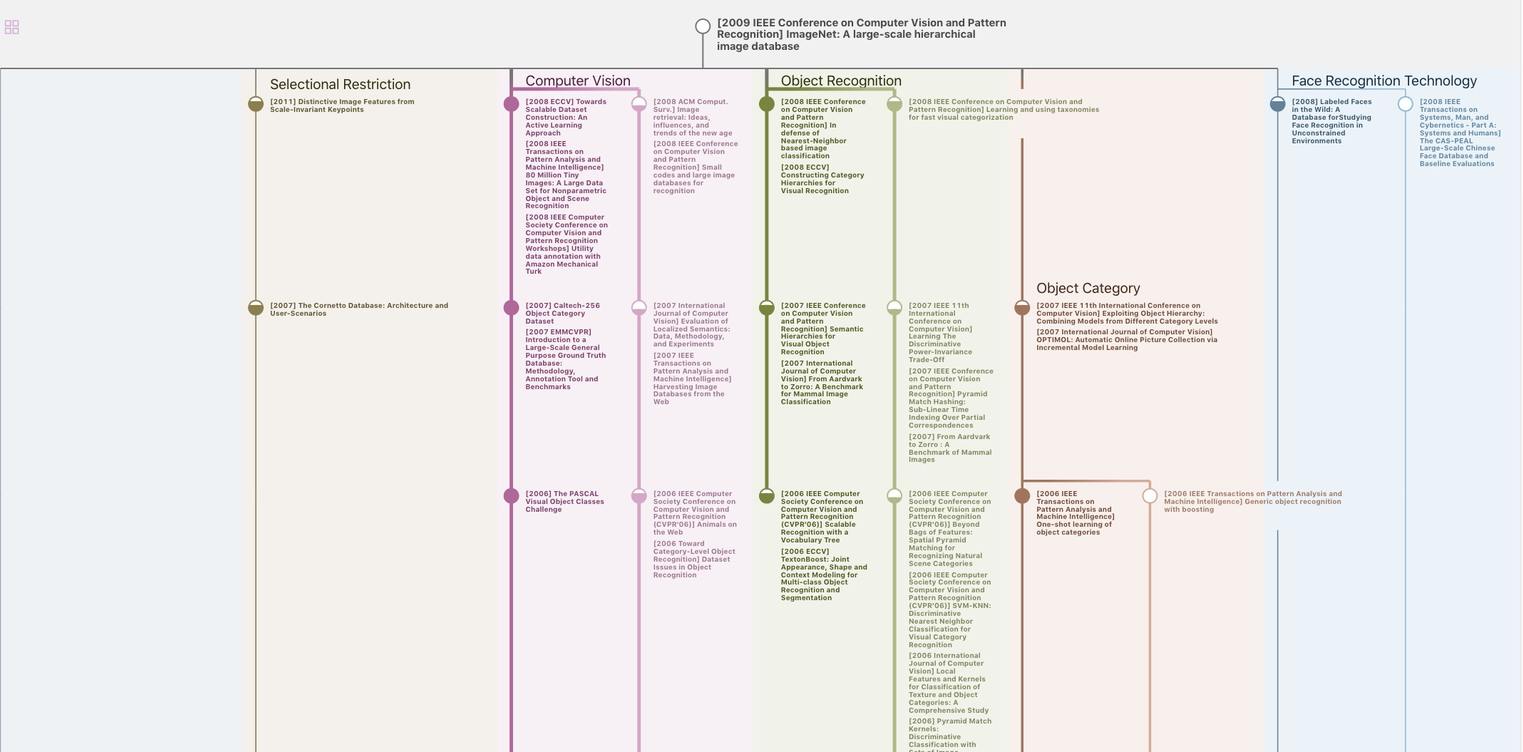
生成溯源树,研究论文发展脉络
Chat Paper
正在生成论文摘要