Self-adaptive local Fisher discriminant analysis for semi-supervised image recognition
Int. J. Biom.(2012)
摘要
In this paper, we present a self-adaptive semi-supervised dimension reduction framework for image class recognition. Compared with the Semi-supervised Local Fisher Discriminant Analysis (SELF), whose classification performance is significantly influenced by some parameters - Neighbour size k of every labelled sample, Affinity Matrix A = {Aij} and Trade-off parameter β, our sparse algorithm is developed based on the distribution of the data set, which is much more adaptive to data set themselves. To develop a more tractable and practical approach, we in particular impose neighourhood structure constraint on the labelled samples in the minimum reconstruction criterion and develop a quadratic optimisation technique to approximately estimate the affine matrix used in the Local Fisher Discriminant Analysis (LFDA). We also give a novel approach to estimate the β automatically. Our experiments on semi-supervised face recognition task demonstrate that the proposed method is more robust and efficient in dealing with the semi-supervised problems in face recognition when compared with the related SELF methods
更多查看译文
关键词
semi-supervised learning, image classification, LFDA, local Fisher discriminant analysis, hypergraph, quadratic programming
AI 理解论文
溯源树
样例
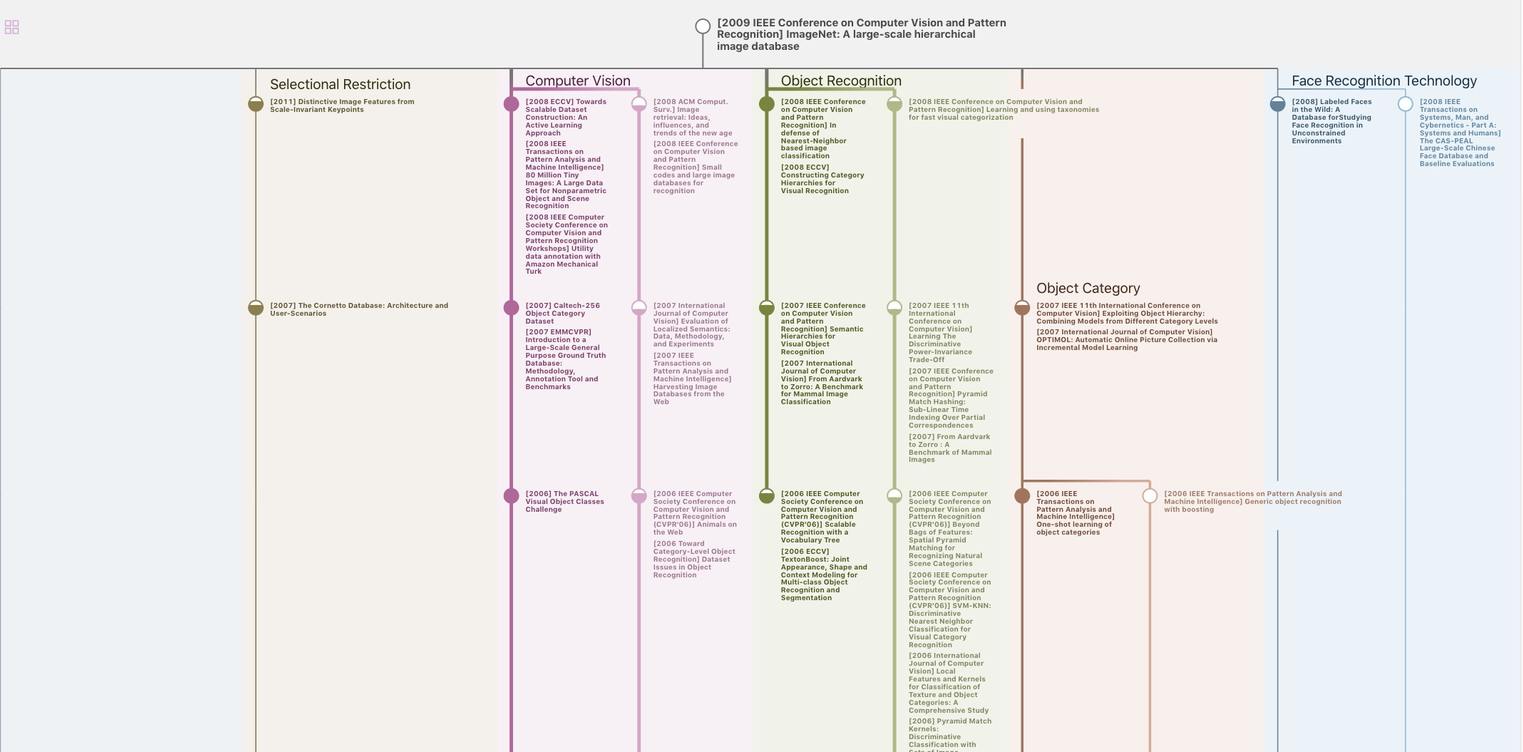
生成溯源树,研究论文发展脉络
Chat Paper
正在生成论文摘要