Robust spatiotemporal matching of electronic slides to presentation videos.
IEEE Transactions on Image Processing(2011)
摘要
We describe a robust and efficient method for automatically matching and time-aligning electronic slides to videos of corresponding presentations. Matching electronic slides to videos provides new methods for indexing, searching, and browsing videos in distance-learning applications. However, robust automatic matching is challenging due to varied frame composition, slide distortion, camera movement, low-quality video capture, and arbitrary slides sequence. Our fully automatic approach combines image-based matching of slide to video frames with a temporal model for slide changes and camera events. To address these challenges, we begin by extracting scale-invariant feature-transformation (SIFT) keypoints from both slides and video frames, and matching them subject to a consistent projective transformation (homography) by using random sample consensus (RANSAC). We use the initial set of matches to construct a background model and a binary classifier for separating video frames showing slides from those without. We then introduce a new matching scheme for exploiting less distinctive SIFT keypoints that enables us to tackle more difficult images. Finally, we improve upon the matching based on visual information by using estimated matching probabilities as part of a hidden Markov model (HMM) that integrates temporal information and detected camera operations. Detailed quantitative experiments characterize each part of our approach and demonstrate an average accuracy of over 95% in 13 presentation videos.
更多查看译文
关键词
video signal processing,homography constraint,visual information,video frames,video indexing and browsing,video browsing,presentation videos,image matching,video searching,camera movement,slide distortion,random processes,distance-learning applications,homography,video presentation,detected camera operations,temporal model,hmm,camera event,image-based matching,presentation video,projective transformation,frame composition,low-quality video,new matching scheme,scale-invariant feature-transformation (sift) keypoints,temporal information,browsing video,background model,quantitative experiments,low-quality video capture,electronic slide,sift keypoints,estimated matching probability,robust automatic matching,electronic slides,video indexing,arbitrary slides sequence,hidden markov model,distance learning,robust spatiotemporal matching,camera events,transforms,scale-invariant feature-transformation keypoints,hidden markov models,technical presentation,binary classifier,random sample consensus,ransac,matching slides to video frames,video frame,probability,indexation,synchronization,accuracy,random sampling,robustness,scale invariant feature transform
AI 理解论文
溯源树
样例
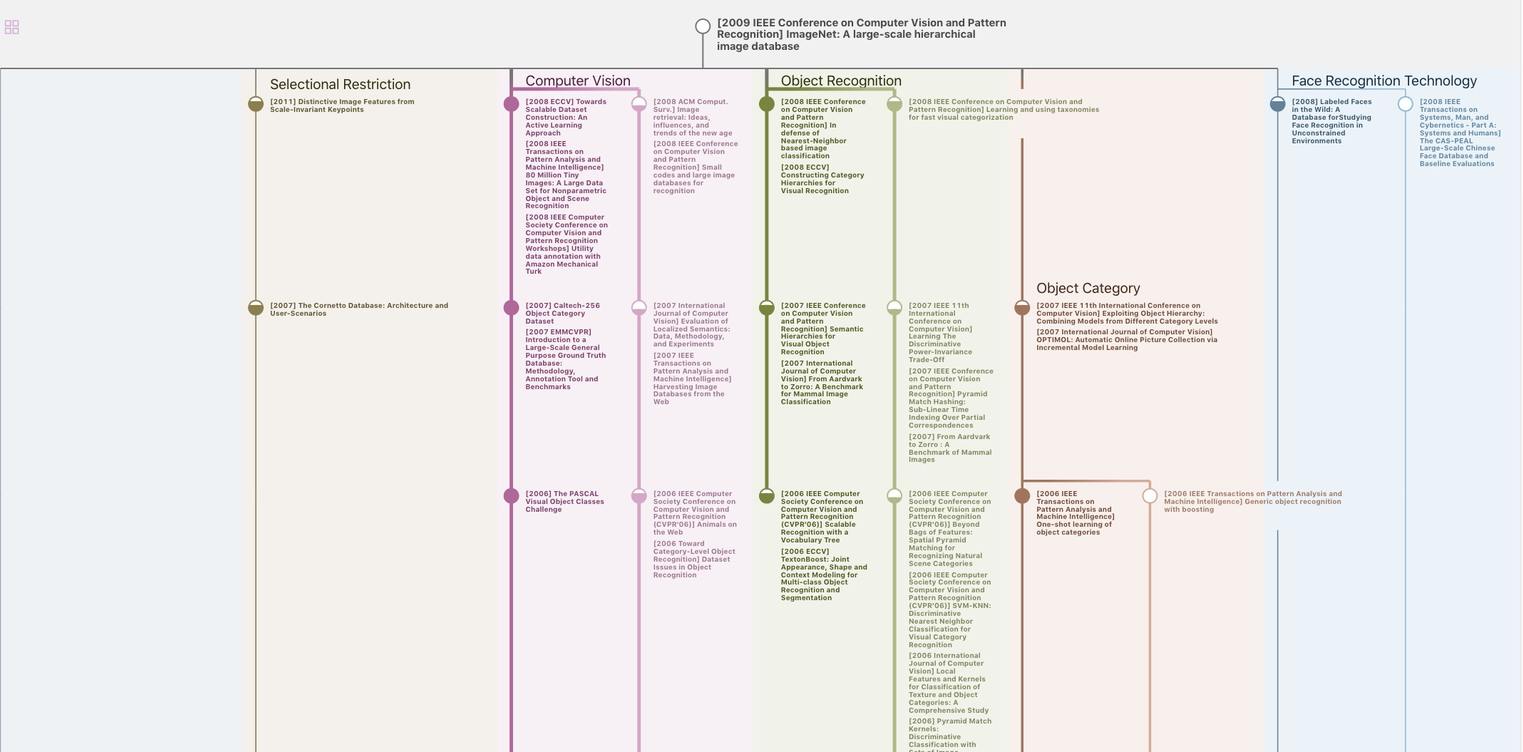
生成溯源树,研究论文发展脉络
Chat Paper
正在生成论文摘要