Robust Adaptive Beamforming Using Multidimensional Covariance Fitting
IEEE Transactions on Signal Processing(2012)
摘要
Over the last decade, several set-based worst-case beamformers have been proposed. It has been shown that some of these beamformers can be formulated equivalently as one-dimensional (1D) covariance fitting problems. Based on this formulation, we show that these beamformers lead to inherently nonoptimum results in the presence of interferers. To mitigate the detrimental effect of interferers, we extend the 1D covariance fitting approach to multidimensional (MD) covariance fitting, modeling the source steering vectors by means of uncertainty sets. The proposed MD covariance fitting approach leads to a nonconvex optimization problem. We develop a convex approximation of this problem, which can be solved, for example, by means of the logarithmic barrier method. The complexity required to compute the barrier function and its first- and second-order derivatives is derived. Simulation results show that the proposed beamformer based on MD covariance fitting achieves an improved performance as compared to the state-of-the-art narrowband beamformers in scenarios with large sample support.
更多查看译文
关键词
covariance fitting,state-of-the-art narrowband beamformers,covariance fitting problem,multidimensional covariance fitting,proposed beamformer,set-based worst-case beamformers,covariance fitting approach,robust adaptive beamforming,md covariance fitting approach,beamformers lead,md covariance fitting,barrier function,adaptive beamforming,robustness,vectors,sensitivity,covariance matrix,second order,semidefinite programming
AI 理解论文
溯源树
样例
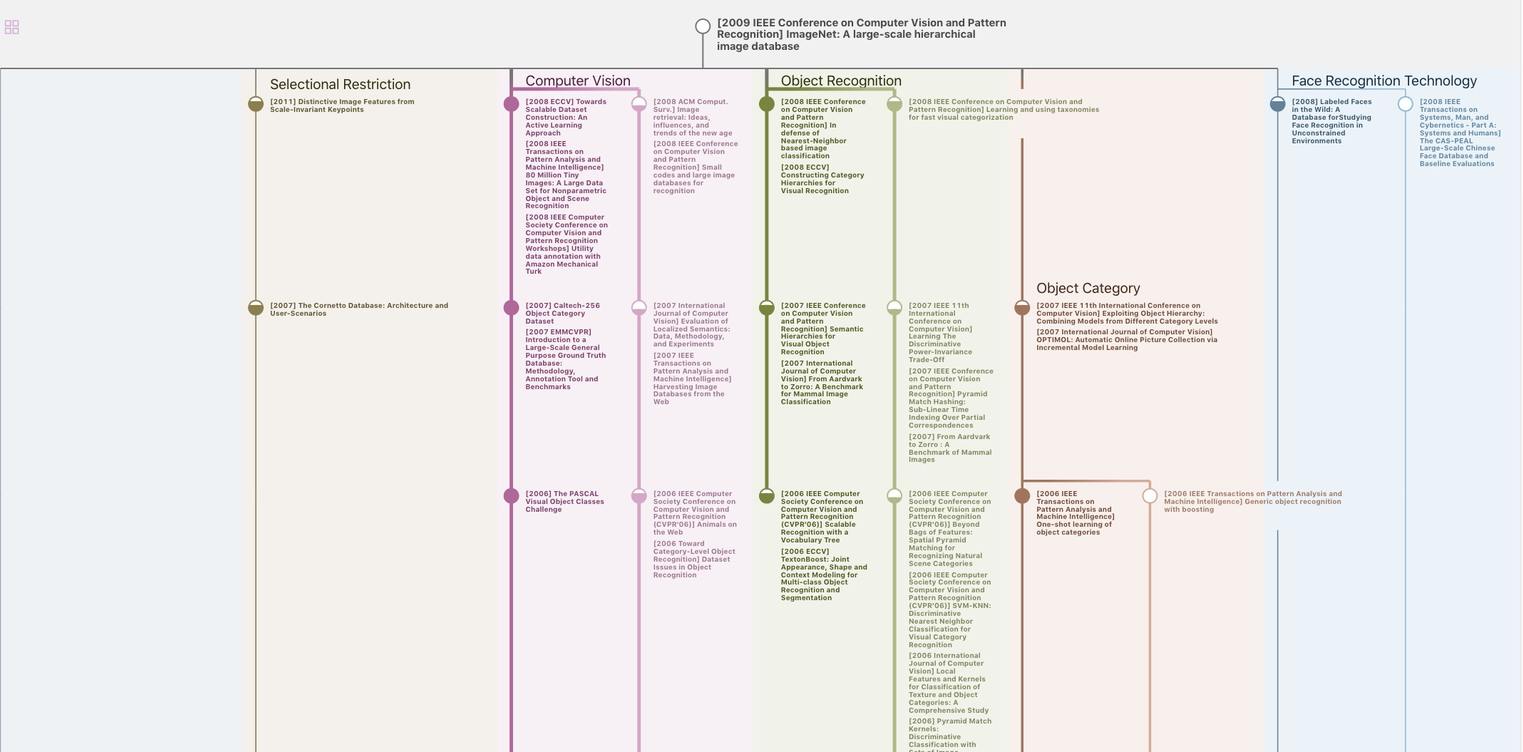
生成溯源树,研究论文发展脉络
Chat Paper
正在生成论文摘要