Video Event Modeling and Recognition in Generalized Stochastic Petri Nets
IEEE Transactions on Circuits and Systems for Video Technology(2010)
摘要
In this paper, we propose the surveillance event recognition framework using Petri Nets (SERF-PN) for recognition of event occurrences in video. The Petri Net (PN) formalism allows a robust way to express semantic knowledge about the event domain as well as efficient algorithms for recognizing events as they occur in a particular video sequence. The major novelties of this paper are extensions to both the modeling and the recognition capacities of the Object PN paradigm. The first contribution of this paper is the extension of the PN representational capacities by introducing stochastic timed transitions to allow modeling of events which have some variance in duration. These stochastic timed transitions sample the duration of the condition from a parametrized distribution. The parameters of this distribution can be specified manually or learned from available video data. A second representational novelty is the use of a single PN to represent the entire event domain, as opposed to previous approaches which have utilized several networks, one for each event of interest. A third contribution of this paper is the capacity to probabilistically predict future events by constructing a discrete time Markov chain model of transitions between states. The experiments section of the paper thoroughly evaluates the application of the SERF-PN framework in the event domains of surveillance and traffic monitoring and provides comparison to other approaches using the CAVIAR dataset , a standard dataset for video analysis applications.
更多查看译文
关键词
single pn,surveillance event recognition framework,particular video sequence,object pn paradigm,video event modeling,available video data,pn representational capacity,generalized stochastic petri nets,future event,event occurrence,entire event domain,event domain,petri net,predictive models,action,behavior,event,data analysis,activity,stochastic processes,petri nets,semantic knowledge,scenario,markov processes,robustness
AI 理解论文
溯源树
样例
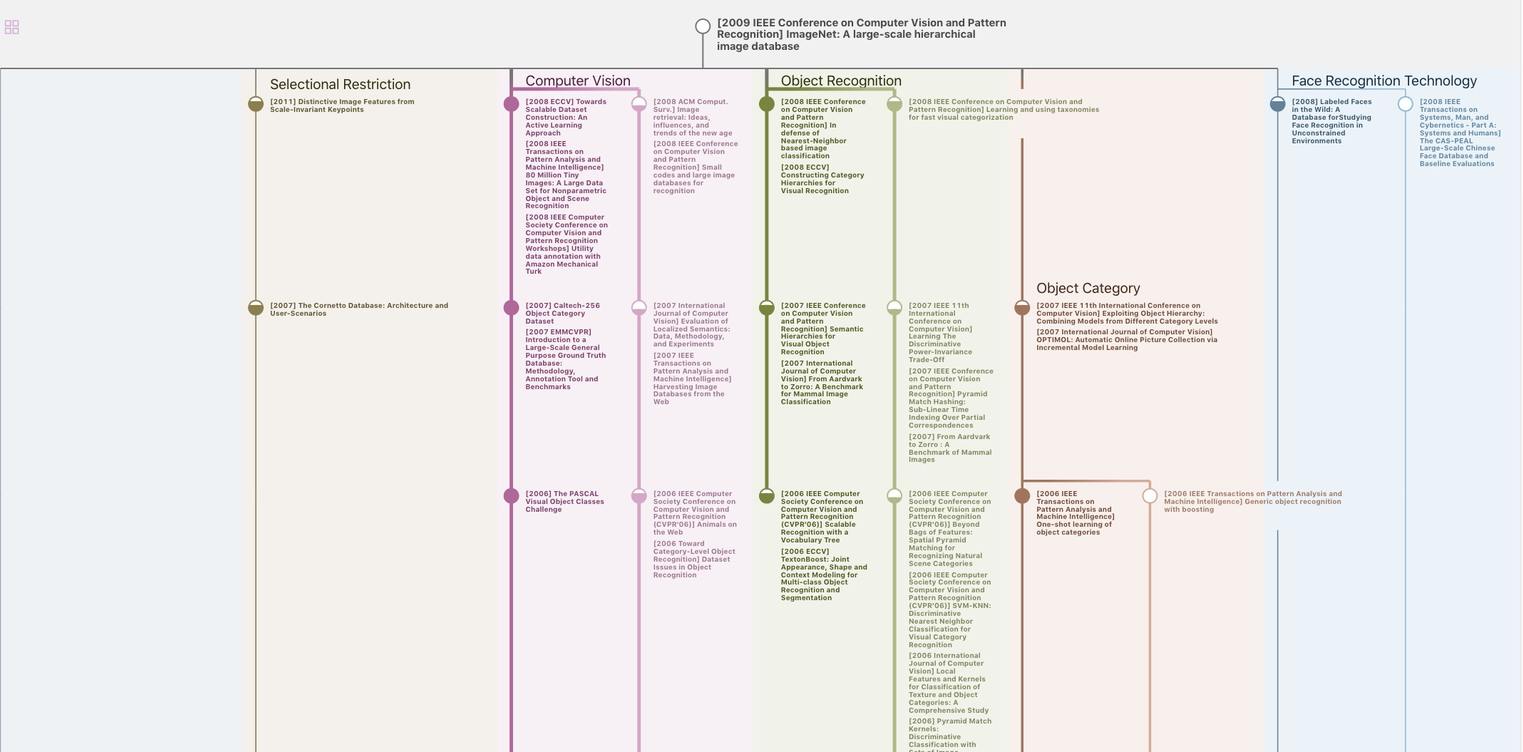
生成溯源树,研究论文发展脉络
Chat Paper
正在生成论文摘要