Pushing the envelope of modern methods for bundle adjustment.
IEEE Transactions on Pattern Analysis and Machine Intelligence(2012)
摘要
In this paper, we present results and experiments with several methods for bundle adjustment, producing the fastest bundle adjuster ever published in terms of computation and convergence. From a computational perspective, the fastest methods naturally handle the block-sparse pattern that arises in a reduced camera system. Adapting to the naturally arising block-sparsity allows the use of BLAS3, efficient memory handling, fast variable ordering, and customized sparse solving, all simultaneously. We present two methods; one uses exact minimum degree ordering and block-based LDL solving and the other uses block-based preconditioned conjugate gradients. Both methods are performed on the reduced camera system. We show experimentally that the adaptation to the natural block sparsity allows both of these methods to perform better than previous methods. Further improvements in convergence speed are achieved by the novel use of embedded point iterations. Embedded point iterations take place inside each camera update step, yielding a greater cost decrease from each camera update step and, consequently, a lower minimum. This is especially true for points projecting far out on the flatter region of the robustifier. Intensive analyses from various angles demonstrate the improved performance of the presented bundler.
更多查看译文
关键词
sparse solving,block-based,bundle adjustment,camera update step,memory handling,exact minimum degree ordering method,modern methods,embedded point iteration,exact minimum degree,computational perspective,point iterations.,block-based ldl,convergence speed,block-based ldl solving method,fastest method,blas3,image reconstruction,block-sparse pattern,3d reconstruction,fastest bundle adjuster,cameras,structure from motion,computer vision,sparse linear solving,variable ordering,block-sparsity,convergence persepctive,conjugate gradient methods,iterative methods,lower minimum,block-based preconditioned conjugate gradient method,image motion analysis,reduced camera system,memory management,barium,sparse matrices,convergence,linear systems,linear system
AI 理解论文
溯源树
样例
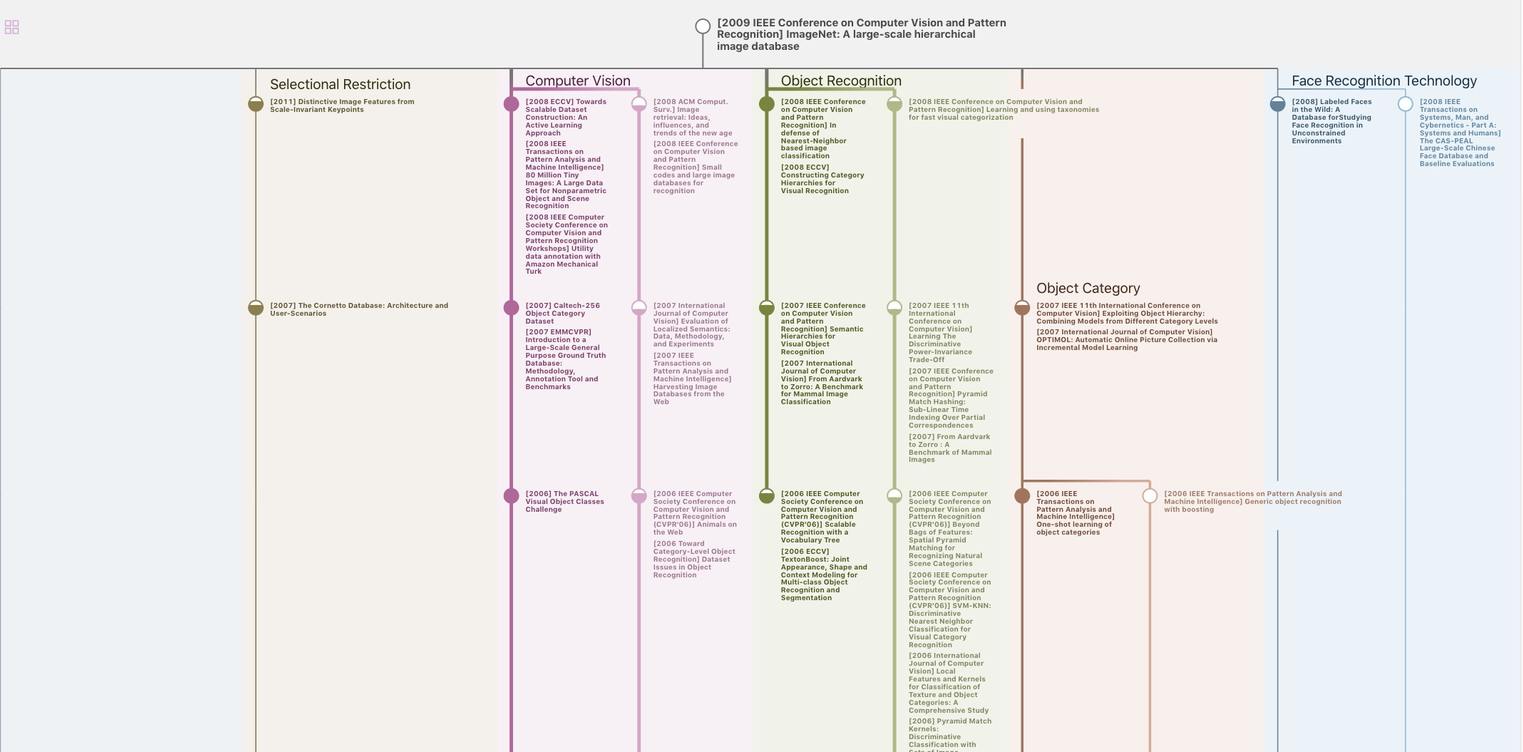
生成溯源树,研究论文发展脉络
Chat Paper
正在生成论文摘要