A parallel genetic algorithm for propensity modeling in consumer finance
SWARM AND EVOLUTIONARY COMPUTATION(2012)
摘要
We consider the problem of propensity modeling in consumer finance. These modeling problems are characterized by the two aspects: the model needs to optimize a business objective which may be nonstandard, and the rate of occurence of the event to be modeled may be very low. Traditional methods such as logistic regression are ill-equipped to deal with nonstandard objectives and low event rates. Methods which deal with the low event rate problem by learning on biased samples face the problem of overlearning. We propose a parallel genetic algorithm method that addresses these challenges. Each parallel process evolves propensity models based on a different biased sample, while a mechanism for validation and cross-pollination between the islands helps address the overlearning issue. We demonstrate the utility of the method on a real-life dataset.
更多查看译文
关键词
traditional method,modeling problem,propensity modeling,overlearning issue,low event rate,nonstandard objective,business objective,consumer finance,parallel genetic algorithm method,low event rate problem,parallel process evolves propensity
AI 理解论文
溯源树
样例
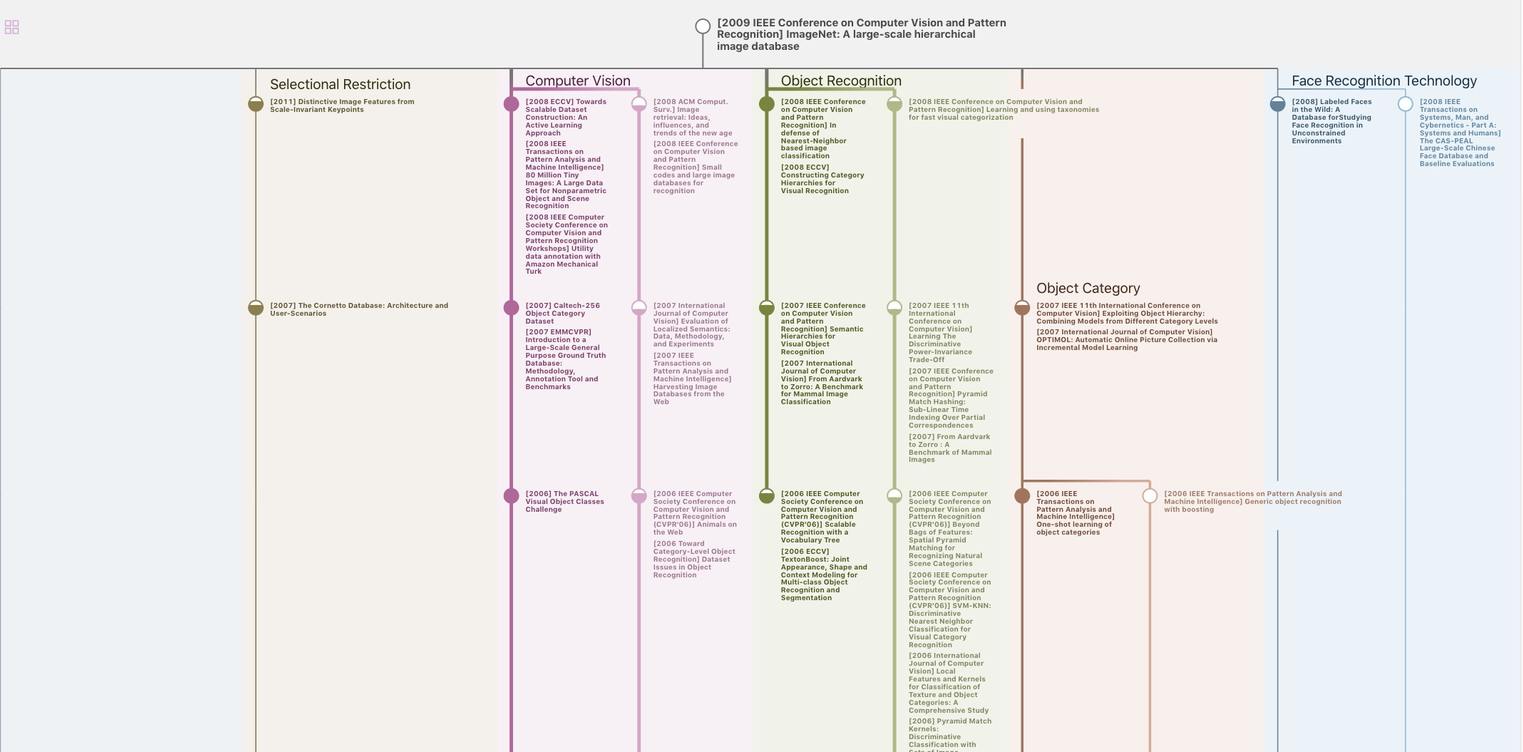
生成溯源树,研究论文发展脉络
Chat Paper
正在生成论文摘要