Constrained SPSA controller for operations processes
Systems, Man and Cybernetics, Part A: Systems and Humans, IEEE Transactions(1999)
摘要
Continuous quality improvement calls for employing methodologies that assist in continual reduction of variations in process performance characteristics around their target values. In a complex operations process the underlying structure of the process is unknown to the operations managers. Hence, identification of the source of variations and variation reduction are difficult and time consuming tasks. Under the assumption that the process design is capable of producing products that meet customer's requirements, the emphasis is on continually improving performance and conformance dimensions of the quality of a complex/nonlinear operations process when there exists almost no knowledge about the process structure. The study considers a case in which some of the process parameters must take values only in pre-specified ranges. It also includes the customer requirements on the product characteristics' values as constraints in the optimization process. Further, the study employs a penalty function for transforming a constrained optimization to an unconstrained one, along with a neural network feedforward controller which is based on simultaneous perturbation stochastic gradient approximation (SPSA). Simulation findings indicate that the constrained optimization will result in a fewer non-conforming outputs than the unconstrained optimization method
更多查看译文
关键词
process performance characteristic,neural network-based model-free controller,neural network,process variation,process dynamic,process performance,constrained spsa controller,operations process parameter,control value,neural network model-free,optimization process,penalty function,process design,constraint optimization,stochastic processes,operations management,process control,feedforward neural networks,quality improvement,operations managers,constrained optimization,statistical process control,neural networks,stress,approximation theory,variation reduction
AI 理解论文
溯源树
样例
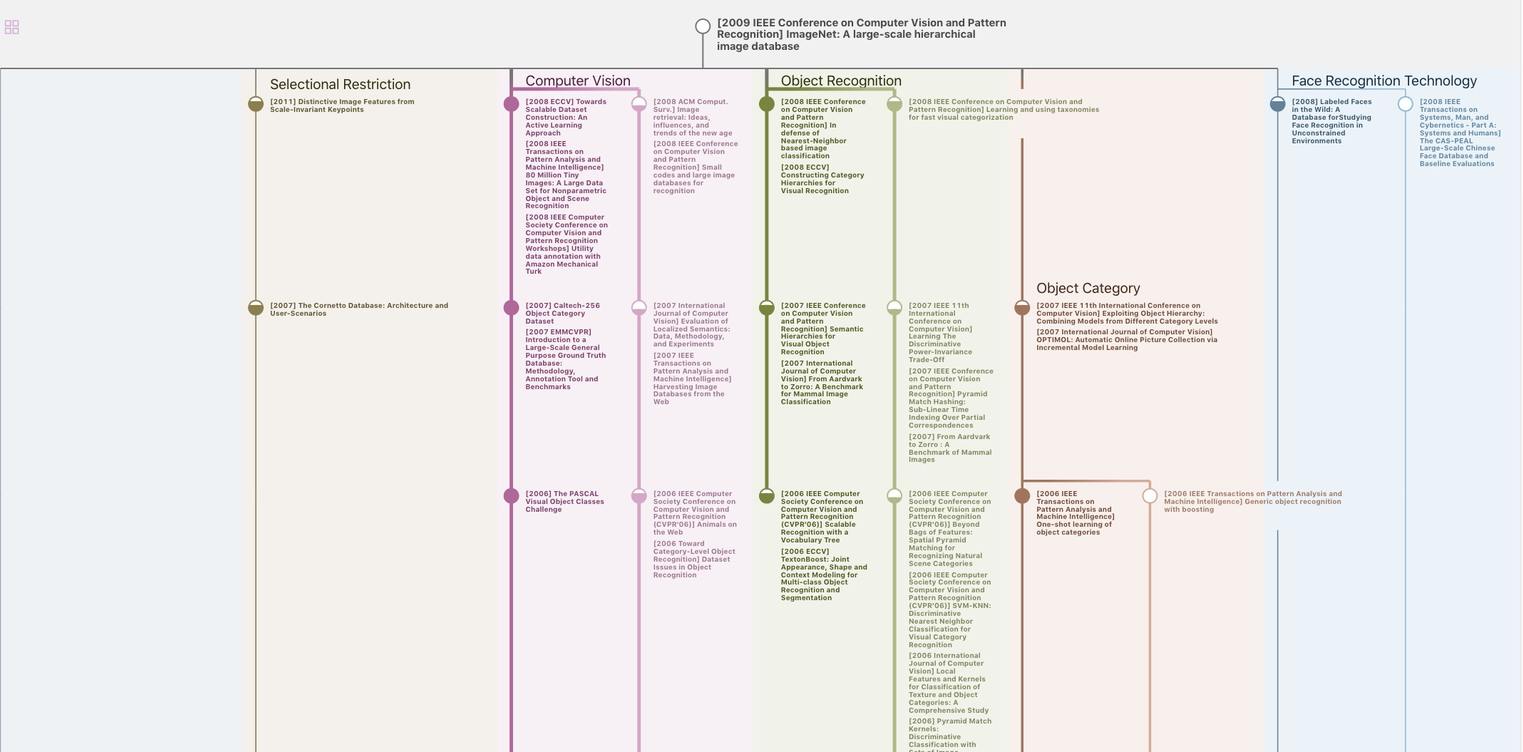
生成溯源树,研究论文发展脉络
Chat Paper
正在生成论文摘要