Intrinsically Motivated Reinforcement Learning: An Evolutionary Perspective
Autonomous Mental Development, IEEE Transactions(2010)
摘要
There is great interest in building intrinsic motivation into artificial systems using the reinforcement learning framework. Yet, what intrinsic motivation may mean computationally, and how it may differ from extrinsic motivation, remains a murky and controversial subject. In this paper, we adopt an evolutionary perspective and define a new optimal reward framework that captures the pressure to design good primary reward functions that lead to evolutionary success across environments. The results of two computational experiments show that optimal primary reward signals may yield both emergent intrinsic and extrinsic motivation. The evolutionary perspective and the associated optimal reward framework thus lead to the conclusion that there are no hard and fast features distinguishing intrinsic and extrinsic reward computationally. Rather, the directness of the relationship between rewarding behavior and evolutionary success varies along a continuum.
更多查看译文
关键词
history,cognitive science,learning artificial intelligence,psychology,helium,system performance,computer experiment,intrinsic motivation,genetic algorithms,computer science,reinforcement learning
AI 理解论文
溯源树
样例
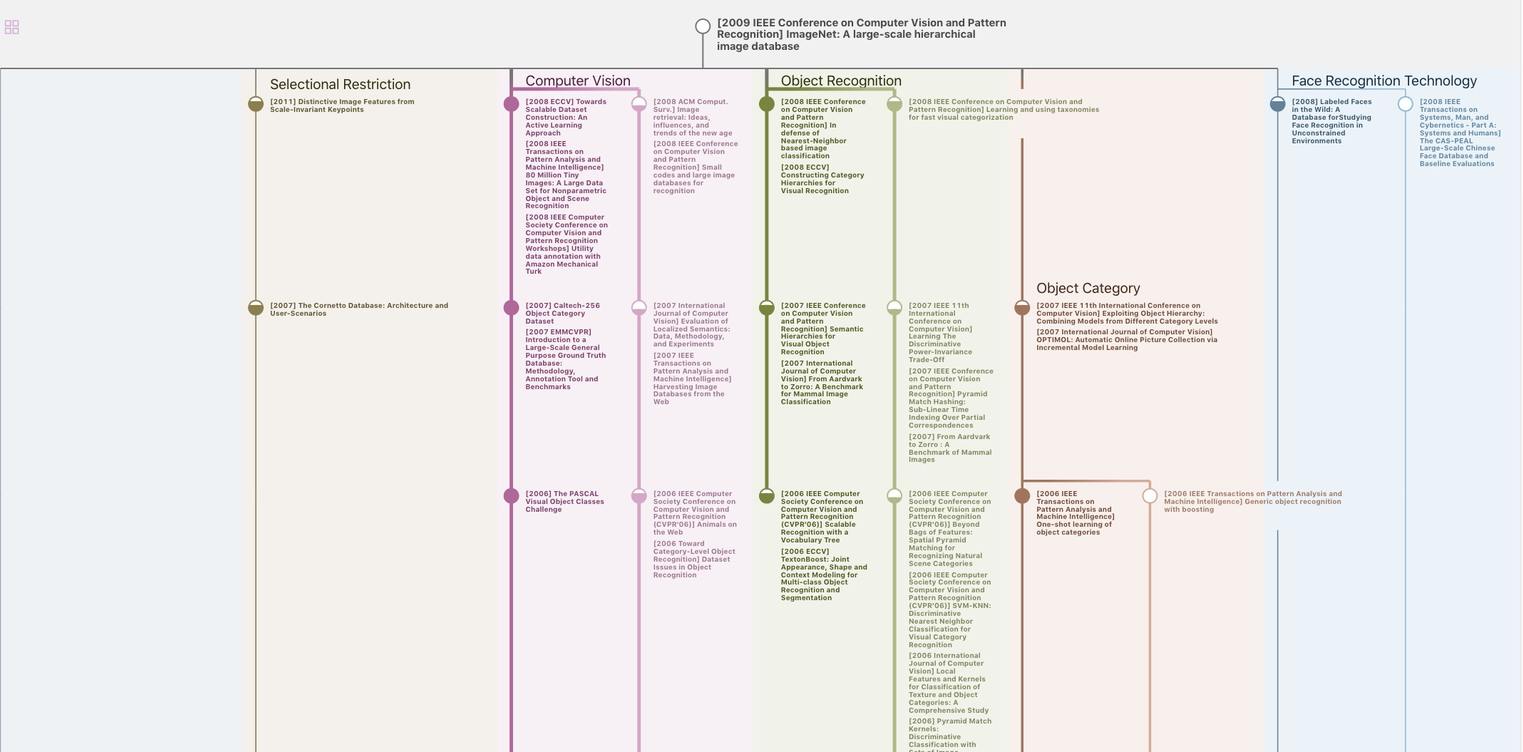
生成溯源树,研究论文发展脉络
Chat Paper
正在生成论文摘要