PAMR: Passive aggressive mean reversion strategy for portfolio selection
Machine Learning(2012)
摘要
This article proposes a novel online portfolio selection strategy named “Passive Aggressive Mean Reversion” (PAMR). Unlike traditional trend following approaches, the proposed approach relies upon the mean reversion relation of financial markets. Equipped with online passive aggressive learning technique from machine learning, the proposed portfolio selection strategy can effectively exploit the mean reversion property of markets. By analyzing PAMR’s update scheme, we find that it nicely trades off between portfolio return and volatility risk and reflects the mean reversion trading principle. We also present several variants of PAMR algorithm, including a mixture algorithm which mixes PAMR and other strategies. We conduct extensive numerical experiments to evaluate the empirical performance of the proposed algorithms on various real datasets. The encouraging results show that in most cases the proposed PAMR strategy outperforms all benchmarks and almost all state-of-the-art portfolio selection strategies under various performance metrics. In addition to its superior performance, the proposed PAMR runs extremely fast and thus is very suitable for real-life online trading applications. The experimental testbed including source codes and data sets is available at http://www.cais.ntu.edu.sg/~chhoi/PAMR/ .
更多查看译文
关键词
Portfolio selection,Mean reversion,Passive aggressive learning,Online learning
AI 理解论文
溯源树
样例
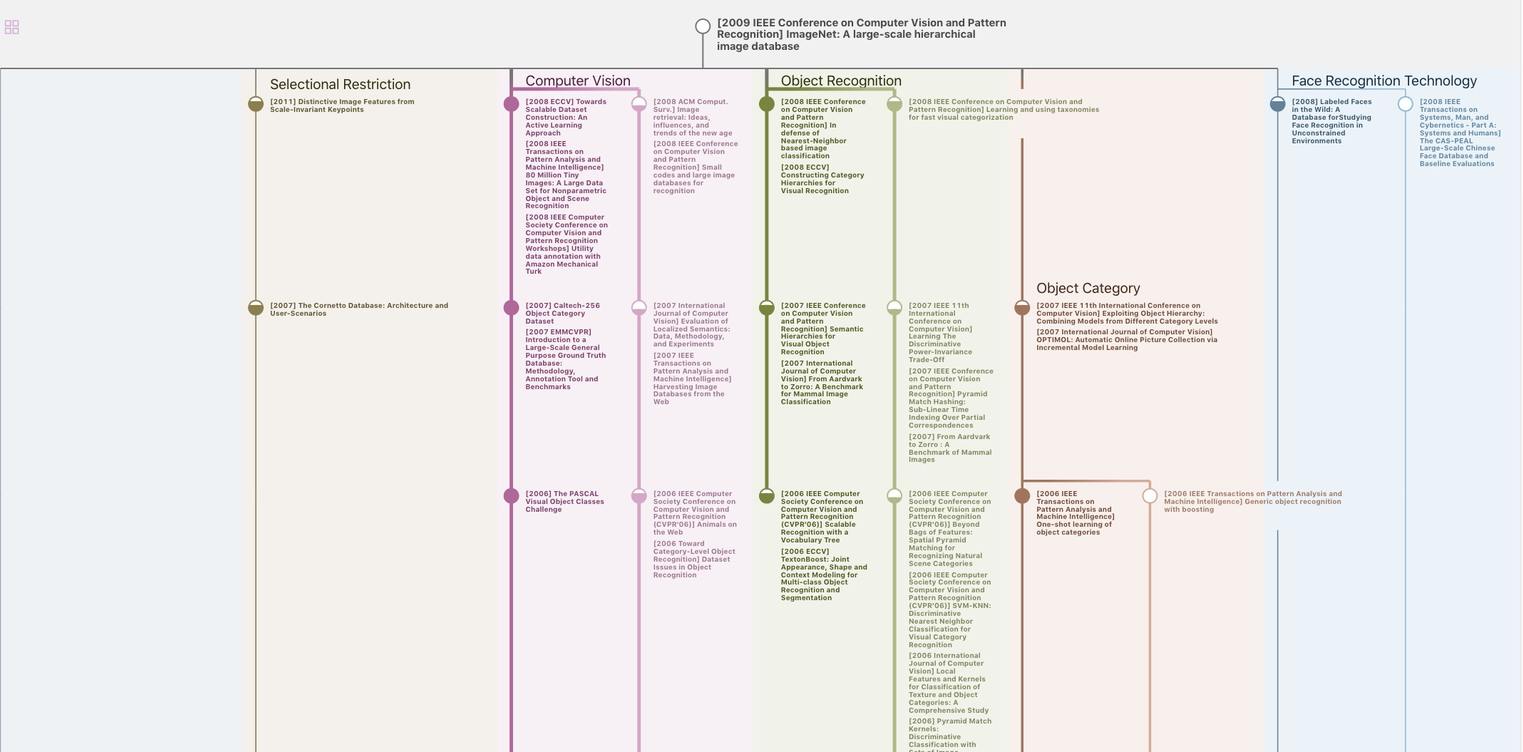
生成溯源树,研究论文发展脉络
Chat Paper
正在生成论文摘要