Kernel Least Mean Square Algorithm With Mixed Kernel
IEEE Transactions on Signal Processing(2018)
摘要
This paper presents a novel kernel least square algorithm with mixed kernel (KLMS-MK) to improve the filtering performance of kernel least mean square (KLMS). By applying the convex combination method to the kernel function in KLMS, KLMS-MK bears the advantages of both the Gaussian kernel and the Laplace kernel. In KLMS-MK, the mixed parameter for the convex combination is updated with the stochastic gradient descent. Therefore, the steady-state mean square error (MSE) and the convergence rate are improved by KLMS-MK, simultaneously. Simulation results on chaotic time series prediction and nonlinear regression validate the excellent performance of KLMS-MK from the aspects of the convergence rate and estimation accuracy.
更多查看译文
关键词
Kernel least mean square algorithm, Gaussian kernel, Laplace kernel, mixed parameter
AI 理解论文
溯源树
样例
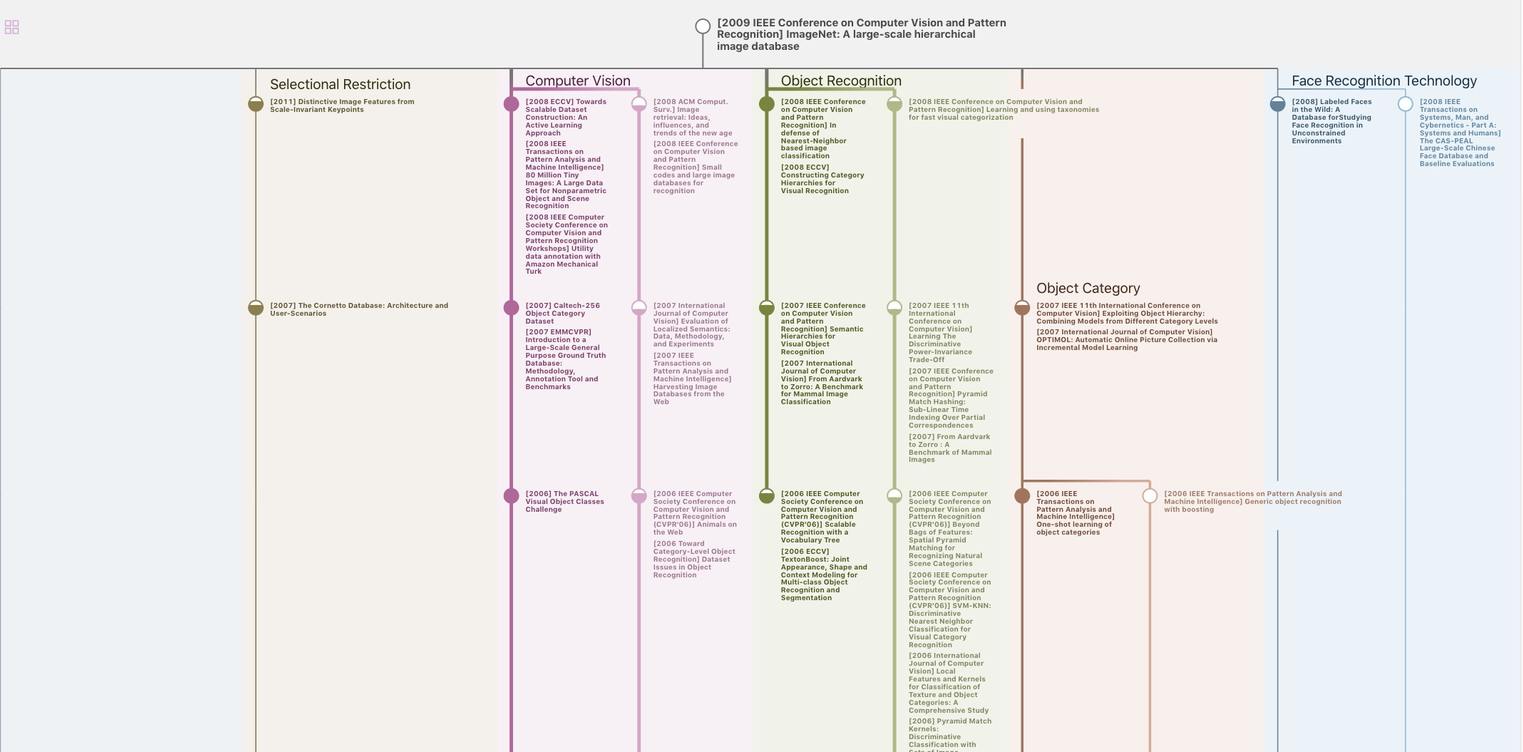
生成溯源树,研究论文发展脉络
Chat Paper
正在生成论文摘要