Information-Driven Distributed Maximum Likelihood Estimation Based on Gauss-Newton Method in Wireless Sensor Networks
IEEE Transactions on Signal Processing(2007)
摘要
In this paper, we develop an energy-efficient distributed estimation method that can be used in applications such as the estimation of a diffusive source and the localization and tracking of an acoustic target in wireless sensor networks. We first propose a statistical measurement model in which we separate the linear and nonlinear parameters. This modeling strategy reduce the processing complexity. We then study the distributed implementation of the Gauss-Newton method in the maximum likelihood estimation. After that we propose a fully distributed estimation approach based on an incremental realization of the Gauss-Newton method. We derive three modifications of the basic algorithm to improve the distributed processing performance while still considering the energy restriction. We implement the idea of information-driven collaborative signal processing and provide a sensor-node scheduling scheme in which the Cramer-Rao bound (CRB) is used as the performance and information utility measure to select the next sensor node. Numerical examples are used to study the performance of the distributed estimation, and we show that of the methods considered here, the proposed multiple iteration Kalman filtering method has the most advantages for wireless sensor networks.
更多查看译文
关键词
gauss-newton method,cramer-rao bound,gauss–newton method,statistical measurement model,kalman filters,signal processing,next sensor node,sensor node scheduling,energy-efficient distributed estimation method,maximum likelihood estimation,wireless sensor network,acoustic target,newton method,maximum-likelihood estimation,estimation method,basic algorithm,iteration kalman filtering method,sensor-node scheduling scheme,energy-efficient distributed estimation,wireless sensor networks,distributed processing performance,information-driven collaborative signal processing,processing complexity,estimation approach,kalman filter,distributed processing,cramer rao bound,energy efficient,maximum likelihood estimate
AI 理解论文
溯源树
样例
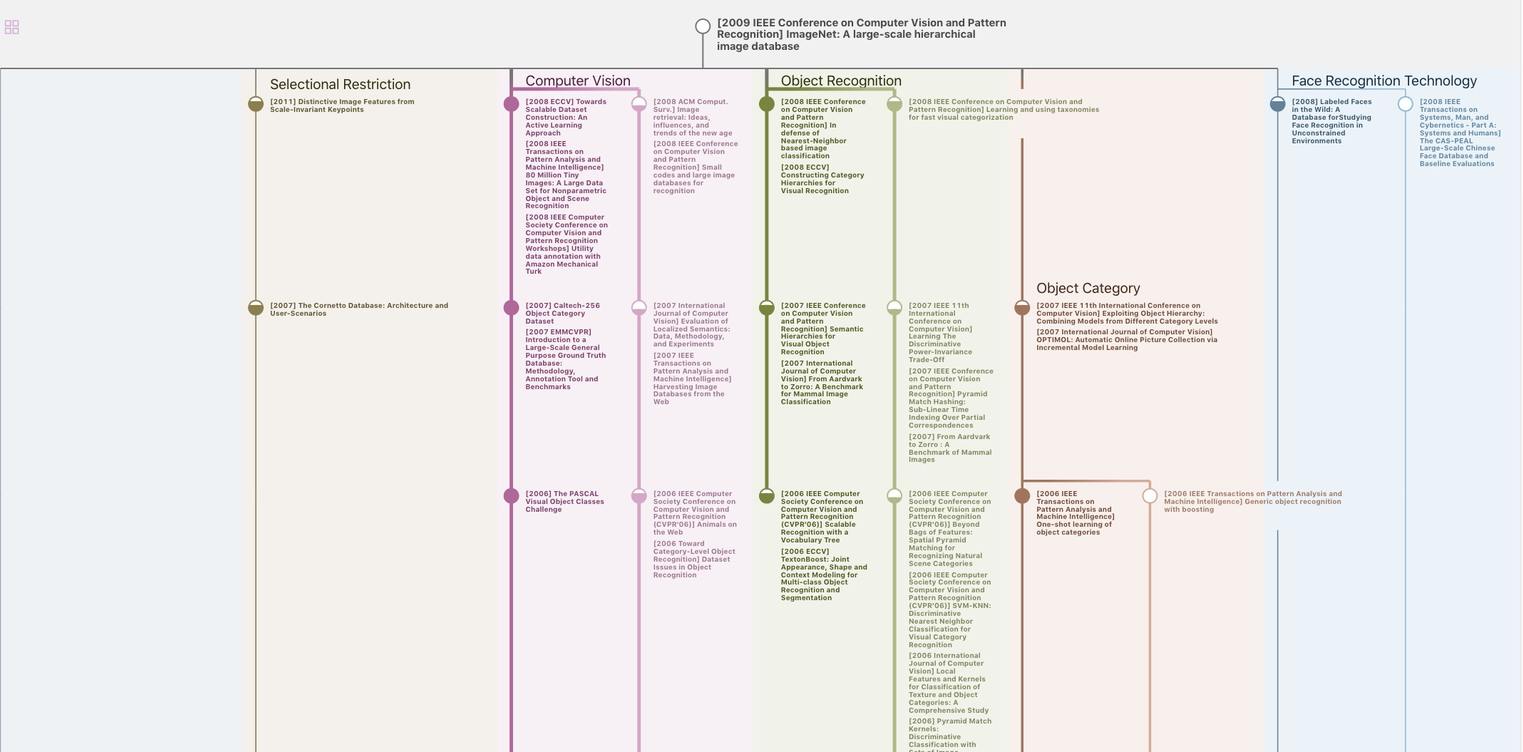
生成溯源树,研究论文发展脉络
Chat Paper
正在生成论文摘要