Detection of divided planar object for augmented reality applications
Virtual Reality Conference(2011)
摘要
In this research study, we propose a divided planar-object detection method for augmented reality(AR) applications. There are mainly two types of camera-registration methods for AR applications: marker-based methods, and natural-feature-based methods. In addition, the latter methods are classified into visual SLAM and object detection methods. With respect to object detection methods, particularly for planar objects such as paper, methods for dealing with bending, folding, and occlusion are proposed. However, the division of objects has not been studied. Once an object is divided, a conventional object detection method cannot identify each of the pieces because the feature points of only a single piece are recognized as the target object, and the other feature points are regarded as outliers. The proposed system prepares a database of the target object's natural features, and applies progressive sample consensus(PROSAC), which is a robust estimation method, for iterative homography calculation to achieve the multiple planar-object detection. Moreover, the proposed method can detect shapes of pieces by simultaneously using an occlusion detection method. We demonstrate that it is possible to interact with an arbitrarily divided planar object in real time by our method to implement some AR applications.
更多查看译文
关键词
robust estimation method,feature point,multiple planar-object detection,augmented reality,detection method,latter method,ar application,planar-object detection method,augmented reality application,conventional object detection method,occlusion detection method,target object,proposed system,planar object,marker-based method,camera-registration method,virtual reality,computer vision,real time systems,iterative methods,feature extraction,art,robust estimator,real time,robustness,indexing terms,estimation,databases,image reconstruction,media
AI 理解论文
溯源树
样例
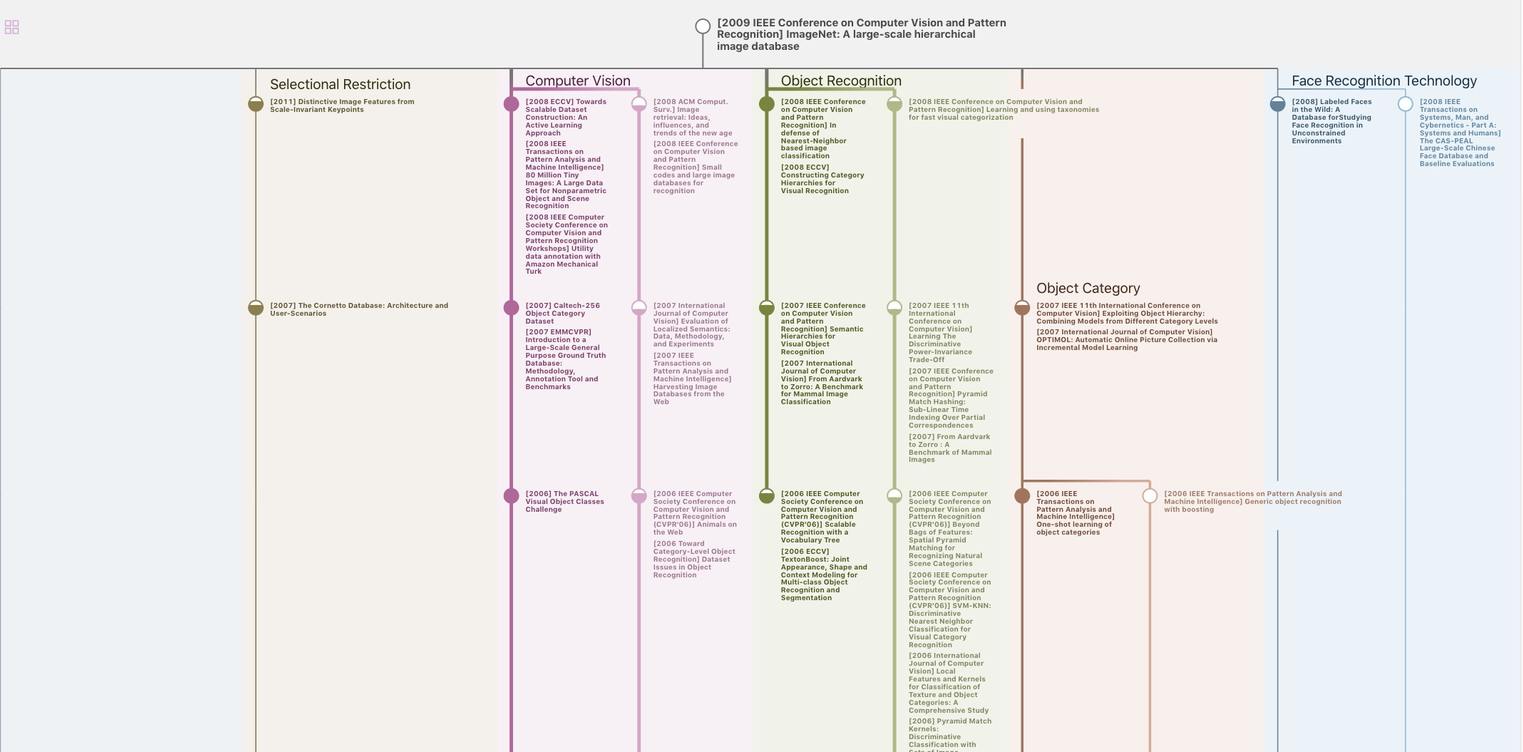
生成溯源树,研究论文发展脉络
Chat Paper
正在生成论文摘要