Performance of unknown and arbitrary sparse signal detection using convex programming method with compressive measurements
Signal Processing and Information Technology(2011)
摘要
We consider the detection of arbitrary and unknown sparse signals against background noise. Under a Neyman-Pearson framework, a new detection scheme referred to as the likelihood ratio test with sparse estimation (LRT-SE) is proposed and analyzed. The error probability of LRT-SE is characterized with respect to the signal-to-noise ratio (SNR) and the estimation error under the high SNR regime. For the low SNR regime, it is shown that there exists a detection boundary on the SNR, above which Chernoff-consistent detection is achievable for LRT-SE. The detection boundary can be calculated using fidelity results on the sparse estimation, and it allows the signal to be consistently detected under vanishing SNR. The error exponent of LRT-SE is also characterized and compared with the oracle exponent assuming signal knowledge. Numerical experiments are used to shown that the proposed method performs in the vicinity of the LRT method and the error probability decays exponentially with the number of observations. Results in this paper also have important implications in showing how well the performance of sparse estimation technique transforms into a hypothesis testing setup.
更多查看译文
关键词
arbitrary sparse signal detection,error probability,compressive measurement,new detection scheme,chernoff-consistent detection,error exponent,detection boundary,convex programming method,sparse estimation,error probability decays exponentially,high snr regime,estimation error,low snr regime,signal to noise ratio,signal detection,statistical testing,likelihood ratio test,convex programming,hypothesis test
AI 理解论文
溯源树
样例
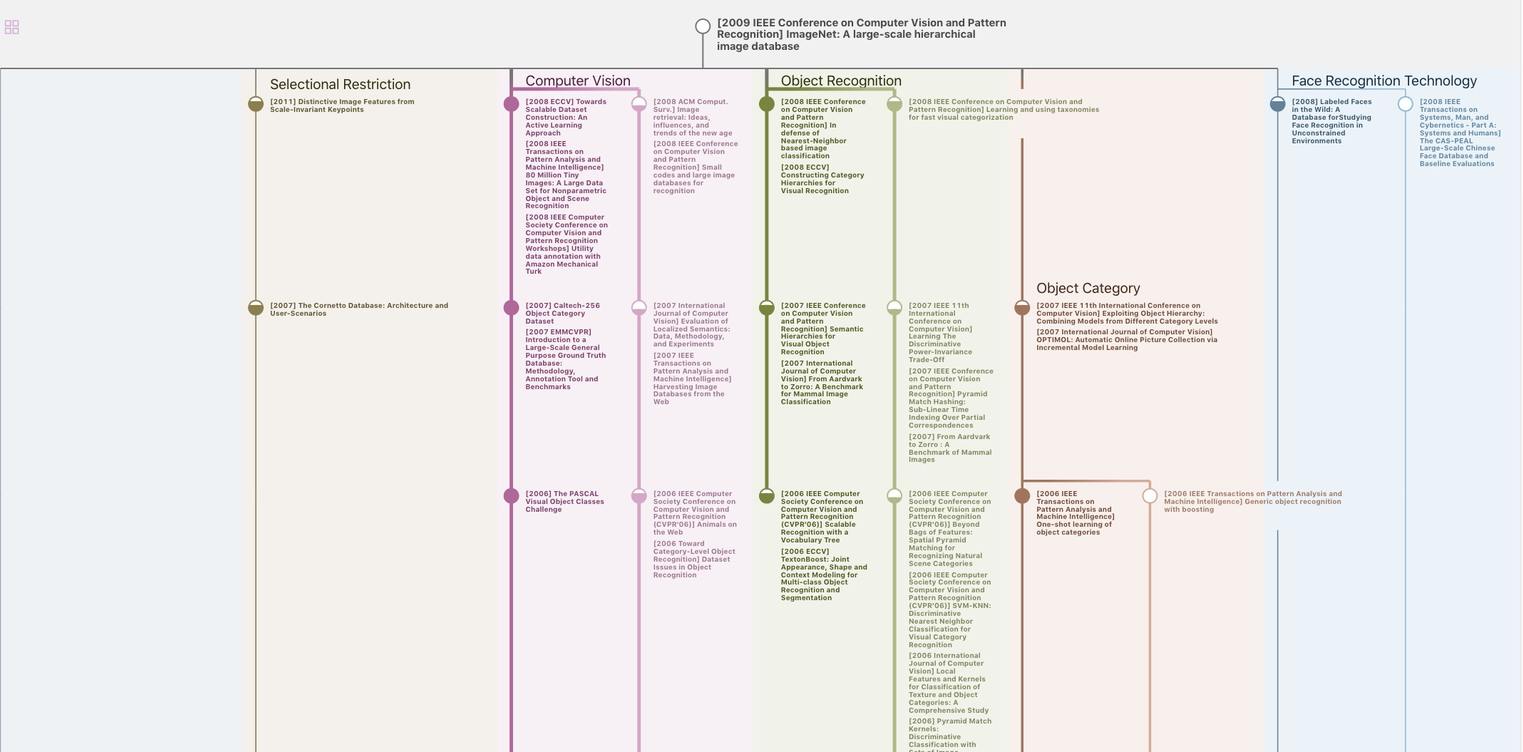
生成溯源树,研究论文发展脉络
Chat Paper
正在生成论文摘要