Learning invariance through imitation
Computer Vision and Pattern Recognition(2011)
摘要
Supervised methods for learning an embedding aim to map high-dimensional images to a space in which perceptually similar observations have high measurable similarity. Most approaches rely on binary similarity, typically defined by class membership where labels are expensive to obtain and/or difficult to define. In this paper we propose crowd-sourcing similar images by soliciting human imitations. We exploit temporal coherence in video to generate additional pairwise graded similarities between the user-contributed imitations. We introduce two methods for learning nonlinear, invariant mappings that exploit graded similarities. We learn a model that is highly effective at matching people in similar pose. It exhibits remarkable invariance to identity, clothing, background, lighting, shift and scale.
更多查看译文
关键词
image matching,learning (artificial intelligence),pose estimation,binary similarity,crowd-sourcing,high-dimensional images,human imitations,invariance learning,invariant mappings,pose,supervised learning methods,temporal coherence
AI 理解论文
溯源树
样例
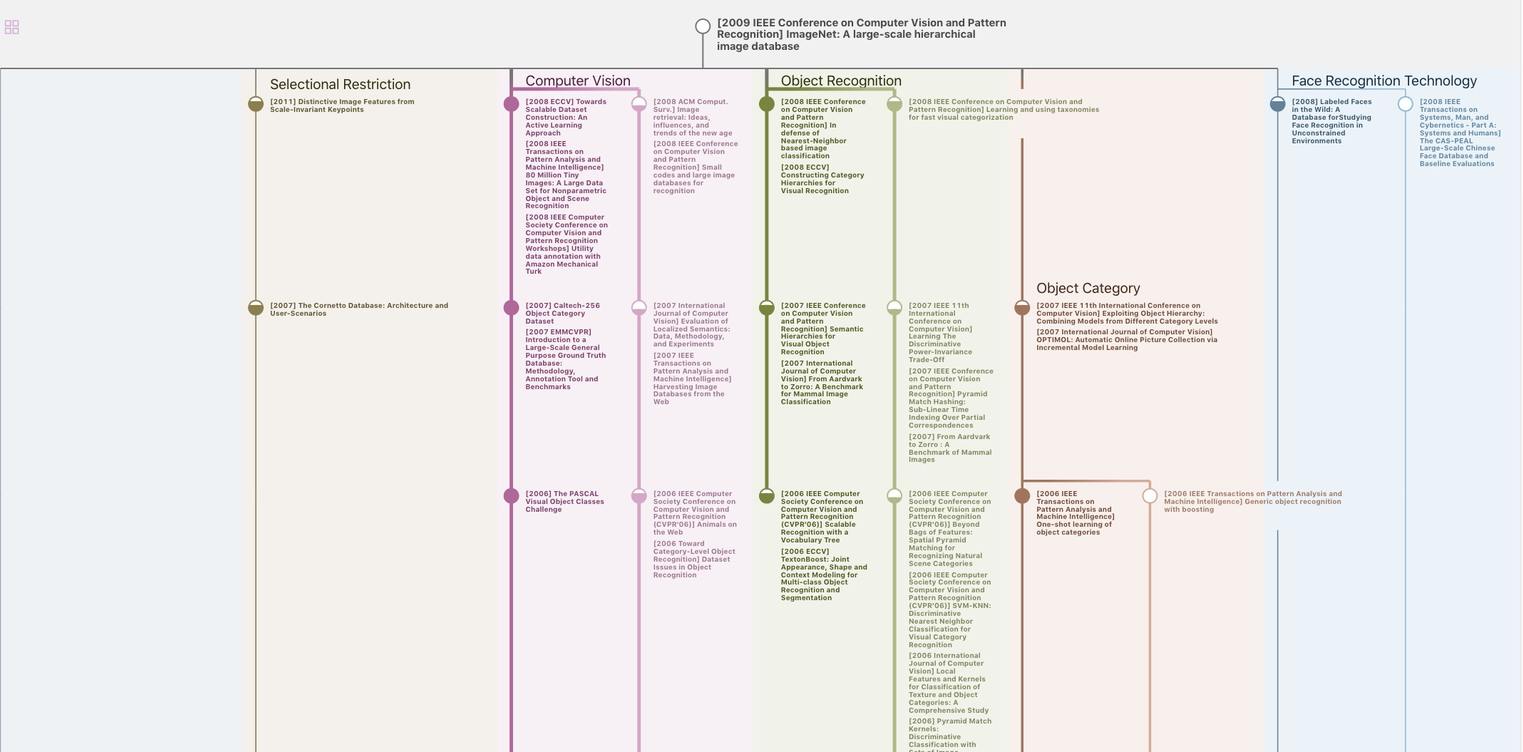
生成溯源树,研究论文发展脉络
Chat Paper
正在生成论文摘要