Optimizing multimedia retrieval using multimodal fusion and relevance feedback techniques
ADVANCES IN MULTIMEDIA MODELING(2012)
摘要
This paper introduces a novel approach for search and retrieval of multimedia content. The proposed framework retrieves multiple media types simultaneously, namely 3D objects, 2D images and audio files, by utilizing an appropriately modified manifold learning algorithm. The latter, which is based on Laplacian Eigenmaps, is able to map the mono-modal low-level descriptors of the different modalities into a new low-dimensional multimodal feature space. In order to accelerate search and retrieval and make the framework suitable even for large-scale applications, a new multimedia indexing scheme is adopted. The retrieval accuracy of the proposed method is further improved through relevance feedback, which enables users to refine their queries by marking the retrieved results as relevant or non-relevant. Experiments performed on a multimodal dataset demonstrate the effectiveness and efficiency of our approach. Finally, the proposed framework can be easily extended to involve as many heterogeneous modalities as possible.
更多查看译文
关键词
new low-dimensional multimodal feature,retrieval accuracy,novel approach,multimedia content,proposed framework,new multimedia indexing scheme,laplacian eigenmaps,multimodal fusion,relevance feedback technique,different modality,audio file,optimizing multimedia retrieval
AI 理解论文
溯源树
样例
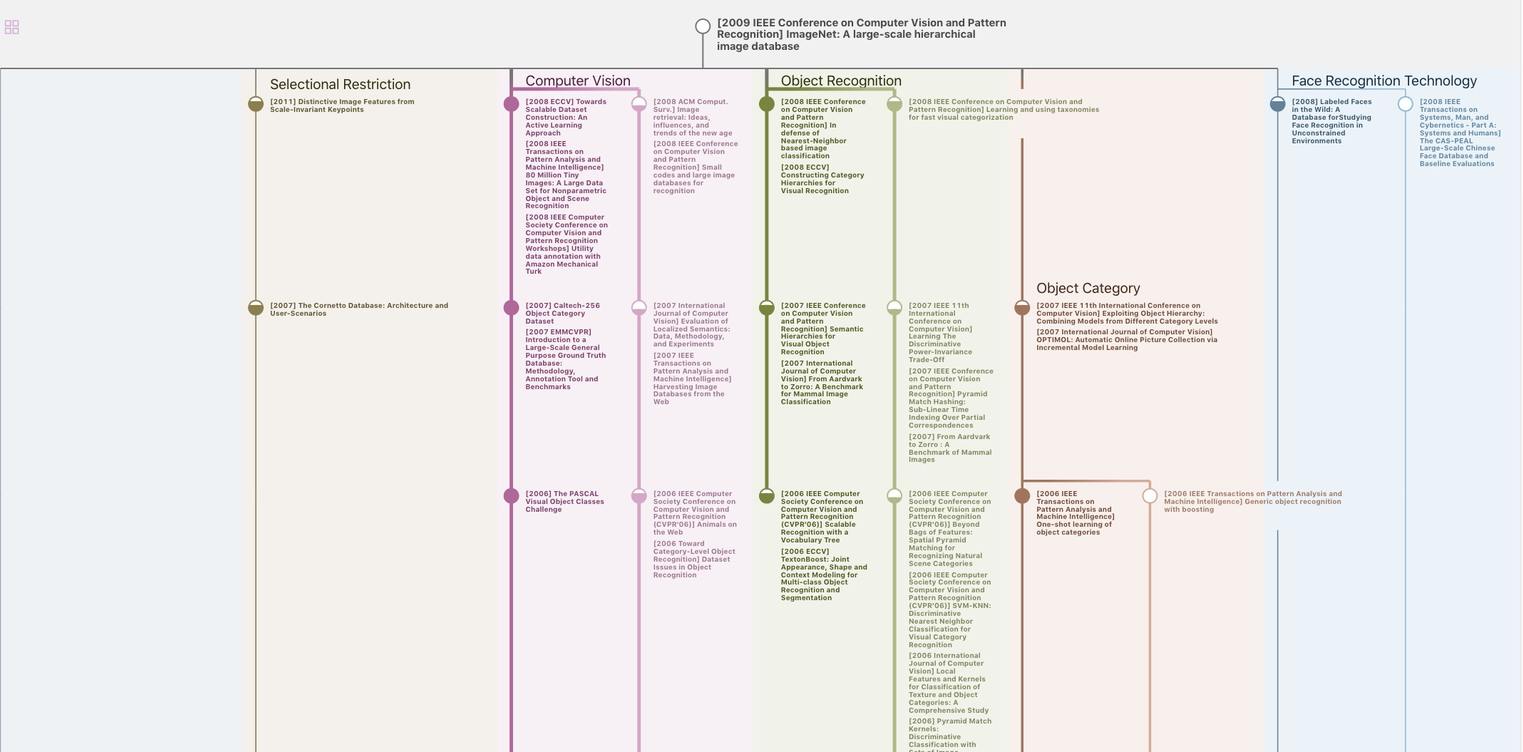
生成溯源树,研究论文发展脉络
Chat Paper
正在生成论文摘要