Effects of data grouping on calibration measures of classifiers
COMPUTER AIDED SYSTEMS THEORY - EUROCAST 2011, PT I(2011)
摘要
The calibration of a probabilistic classifier refers to the extend to which its probability estimates match the true class membership probabilities. Measuring the calibration of a classifier usually relies on performing chi-squared goodness-of-fit tests between grouped probabilities and the observations in these groups. We considered alternatives to the Hosmer-Lemeshow test, the standard chi-squared test with groups based on sorted model outputs. Since this grouping does not represent "natural" groupings in data space, we investigated a chi-squared test with grouping strategies in data space. Using a series of artificial data sets for which the correct models are known, and one real-world data set, we analyzed the performance of the Pigeon-Heyse test with groupings by self-organizing maps, k-means clustering, and random assignment of points to groups. We observed that the Pigeon-Heyse test offers slightly better performance than the Hosmer-Lemeshow test while being able to locate regions of poor calibration in data space.
更多查看译文
关键词
real-world data,poor calibration,chi-squared goodness-of-fit test,chi-squared test,artificial data set,data space,hosmer-lemeshow test,pigeon-heyse test,calibration measure,standard chi-squared test,better performance
AI 理解论文
溯源树
样例
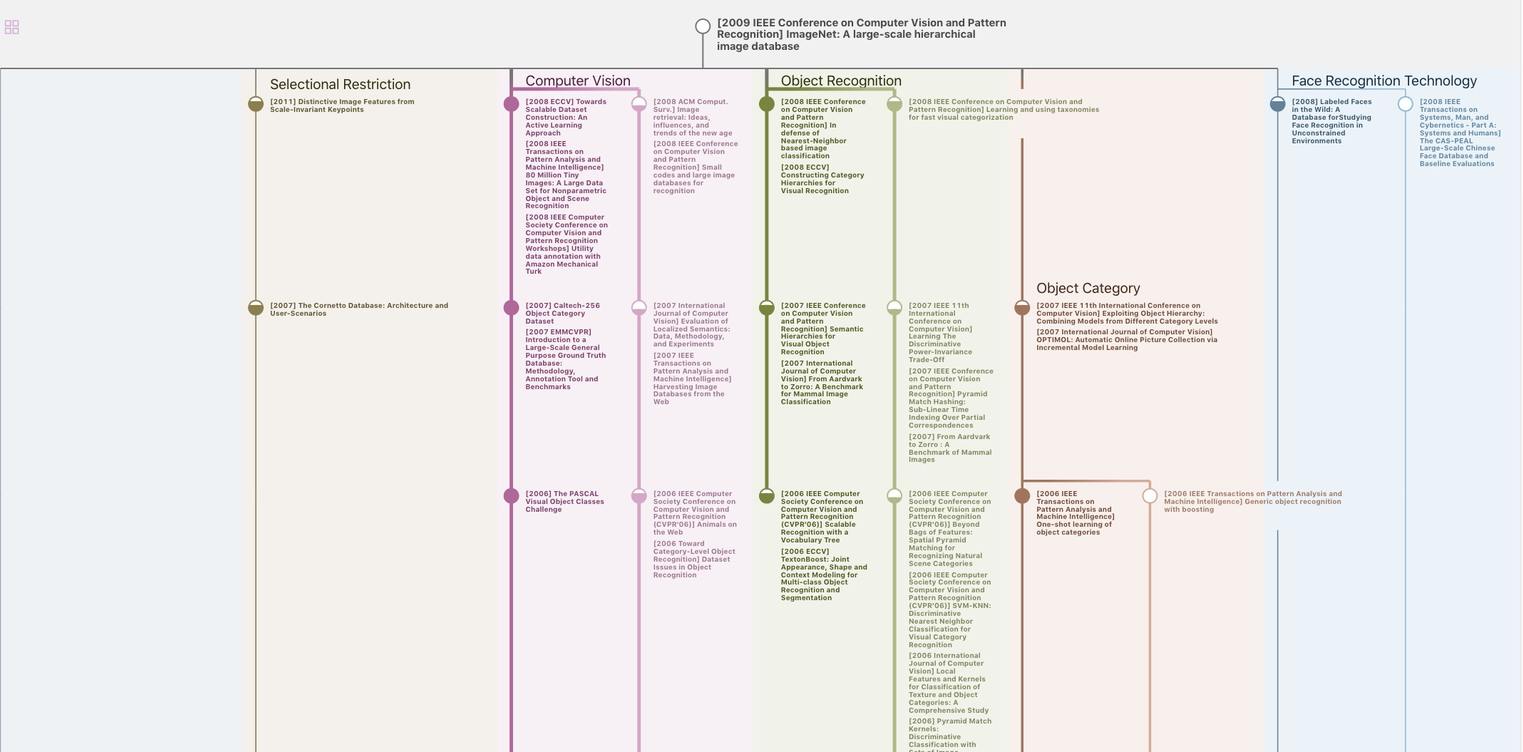
生成溯源树,研究论文发展脉络
Chat Paper
正在生成论文摘要