Learning more from experience in case-based reasoning
CASE-BASED REASONING RESEARCH AND DEVELOPMENT, ICCBR 2011(2011)
摘要
Recent concerns about the effects of feedback delays on solution quality in case-based reasoning (CBR) have prompted research interest in feedback propagation as an approach to addressing the problem. We argue in this paper that the ability of CBR systems to learn from experience in the absence of immediate feedback is limited by eager commitment to the adaptation paths used to solve previous problems. Moreover, it is this departure from lazy learning in CBR that creates the need for maintenance interventions such as feedback propagation. We also show that adaptation path length has no direct effect on solution quality in many adaptation methods and examine the implications for problem solving and learning in CBR. For such "path invariant" adaptation methods, we demonstrate the effectiveness of a "lazier" approach to learning/problem solving in CBR that avoids commitment to previous adaptation paths and hence the need for feedback propagation.
更多查看译文
关键词
cbr system,immediate feedback,adaptation path,case-based reasoning,adaptation path length,feedback delay,previous adaptation path,adaptation method,feedback propagation,solution quality,lazy learning
AI 理解论文
溯源树
样例
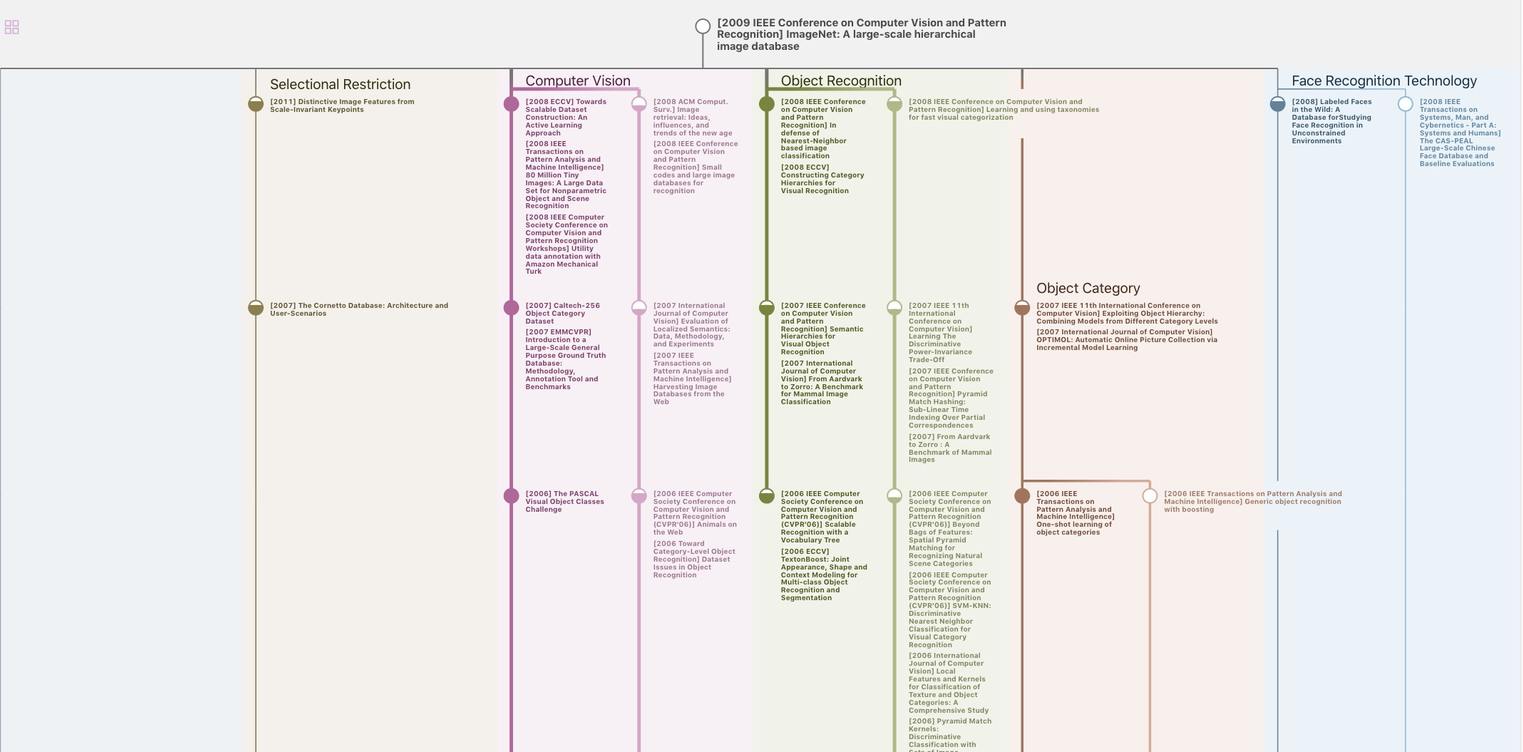
生成溯源树,研究论文发展脉络
Chat Paper
正在生成论文摘要