Continuous upper confidence trees
LION(2011)
摘要
Upper Confidence Trees are a very efficient tool for solving Markov Decision Processes; originating in difficult games like the game of Go, it is in particular surprisingly efficient in high dimensional problems. It is known that it can be adapted to continuous domains in some cases (in particular continuous action spaces). We here present an extension of Upper Confidence Trees to continuous stochastic problems. We (i) show a deceptive problem on which the classical Upper Confidence Tree approach does not work, even with arbitrarily large computational power and with progressive widening (ii) propose an improvement, termed double-progressive widening, which takes care of the compromise between variance (we want infinitely many simulations for each action/state) and bias (we want sufficiently many nodes to avoid a bias by the first nodes) and which extends the classical progressive widening (iii) discuss its consistency and show experimentally that it performs well on the deceptive problem and on experimental benchmarks. We guess that the double-progressive widening trick can be used for other algorithms as well, as a general tool for ensuring a good bias/variance compromise in search algorithms.
更多查看译文
关键词
classical progressive widening,double-progressive widening,continuous upper confidence tree,upper confidence trees,progressive widening,continuous domain,deceptive problem,continuous stochastic problem,classical upper confidence tree,double-progressive widening trick,good bias
AI 理解论文
溯源树
样例
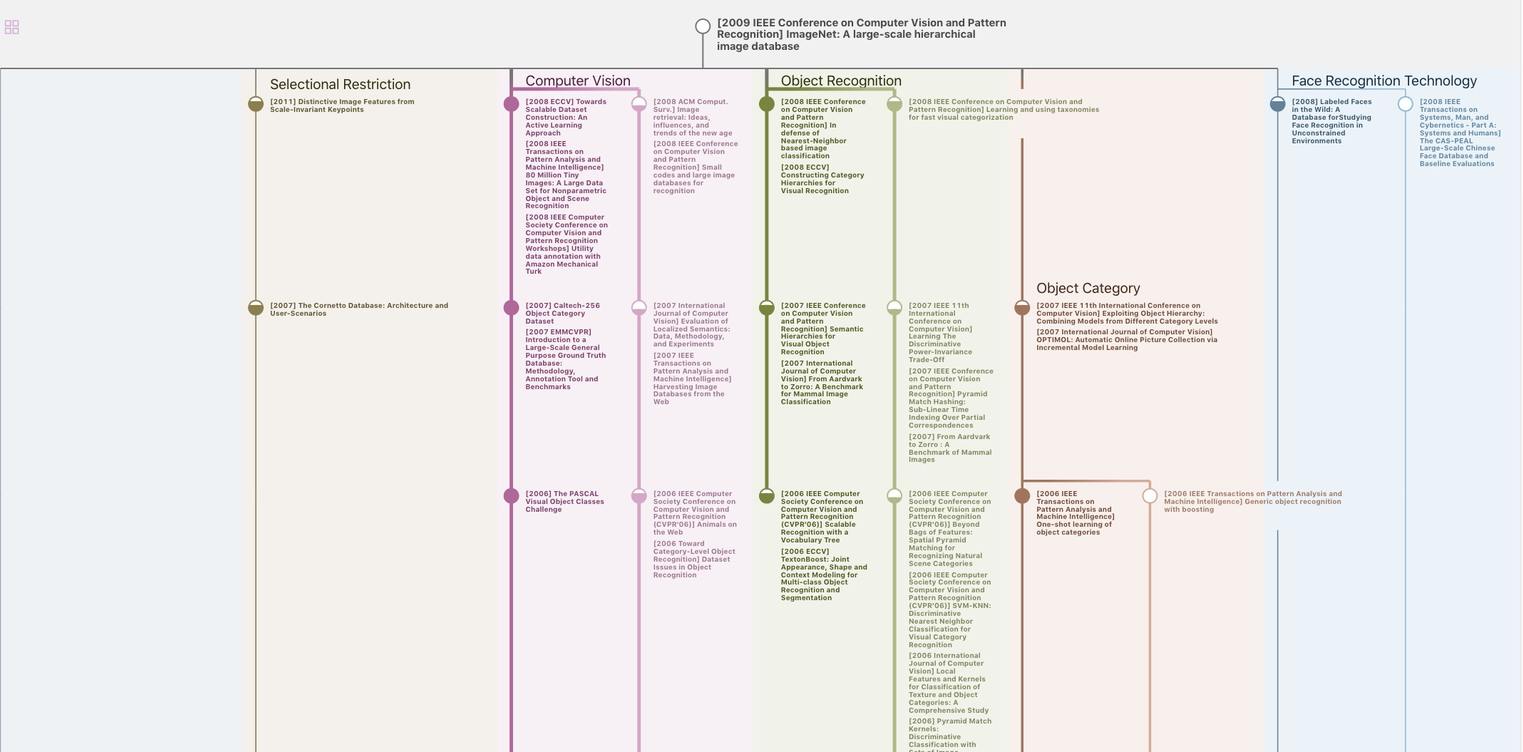
生成溯源树,研究论文发展脉络
Chat Paper
正在生成论文摘要