Adaptive feedback inhibition improves pattern discrimination learning
ARTIFICIAL NEURAL NETWORKS IN PATTERN RECOGNITION, PROCEEDINGS(2006)
摘要
Neural network models for unsupervised pattern recognition learning are challenged when the difference between the patterns of the training set is small. The standard neural network architecture for pattern recognition learning consists of adaptive forward connections and lateral inhibition, which provides competition between output neurons. We propose an additional adaptive inhibitory feedback mechanism, to emphasize the difference between training patterns and improve learning. We present an implementation of adaptive feedback inhibition for spiking neural network models, based on spike timing dependent plasticity (STDP). When the inhibitory feedback connections are adjusted using an anti-Hebbian learning rule, feedback inhibition suppresses the redundant activity of input units which code the overlap between similar stimuli. We show, that learning speed and pattern discriminatability can be increased by adding this mechanism to the standard architecture.
更多查看译文
关键词
adaptive feedback inhibition,additional adaptive inhibitory feedback,lateral inhibition,adaptive forward connection,unsupervised pattern recognition learning,pattern recognition learning,feedback inhibition,neural network model,pattern discriminatability,inhibitory feedback connection,pattern discrimination learning,neural network,pattern recognition,discrimination learning,feedback mechanism,spiking neural network,hebbian learning
AI 理解论文
溯源树
样例
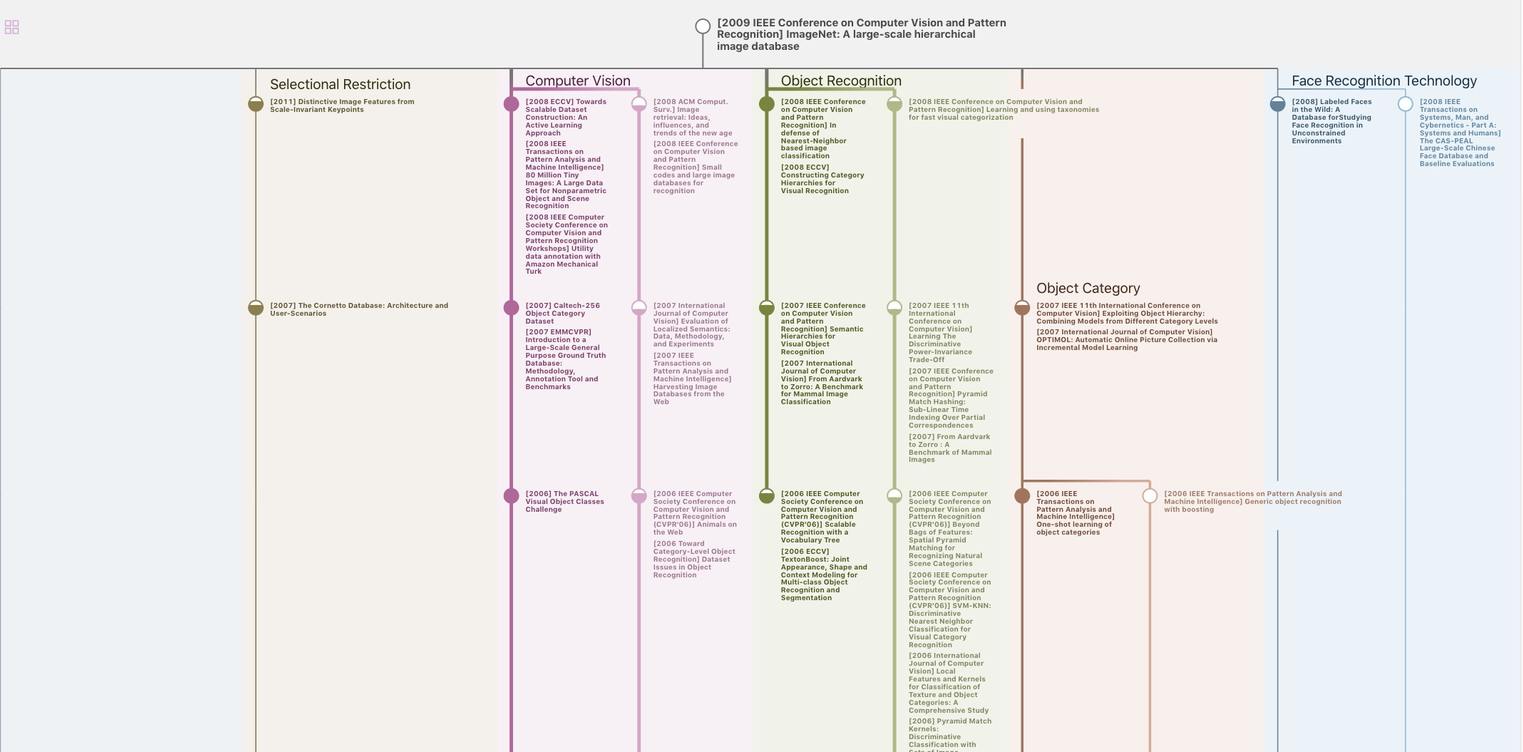
生成溯源树,研究论文发展脉络
Chat Paper
正在生成论文摘要