Classifying relational data with neural networks
INDUCTIVE LOGIC PROGRAMMING, PROCEEDINGS(2005)
摘要
We introduce a novel method for relational learning with neural networks. The contributions of this paper are threefold. First, we introduce the concept of relational neural networks: feedforward networks with some recurrent components, the structure of which is determined by the relational database schema. For classifying a single tuple, they take as inputs the attribute values of not only the tuple itself, but also of sets of related tuples. We discuss several possible architectures for such networks. Second, we relate the expressiveness of these networks to the ‘aggregation vs. selection' dichotomy in current relational learners, and argue that relational neural networks can learn non-trivial combinations of aggregation and selection, a task beyond the capabilities of most current relational learners. Third, we present and motivate different possible training strategies for such networks. We present experimental results on synthetic and benchmark data sets that support our claims and yield insight in the behaviour of the proposed training strategies.
更多查看译文
关键词
single tuple,attribute value,possible architecture,neural network,relational learning,different possible training strategy,proposed training strategy,relational neural network,relational database schema,current relational learner,relational data,relational database
AI 理解论文
溯源树
样例
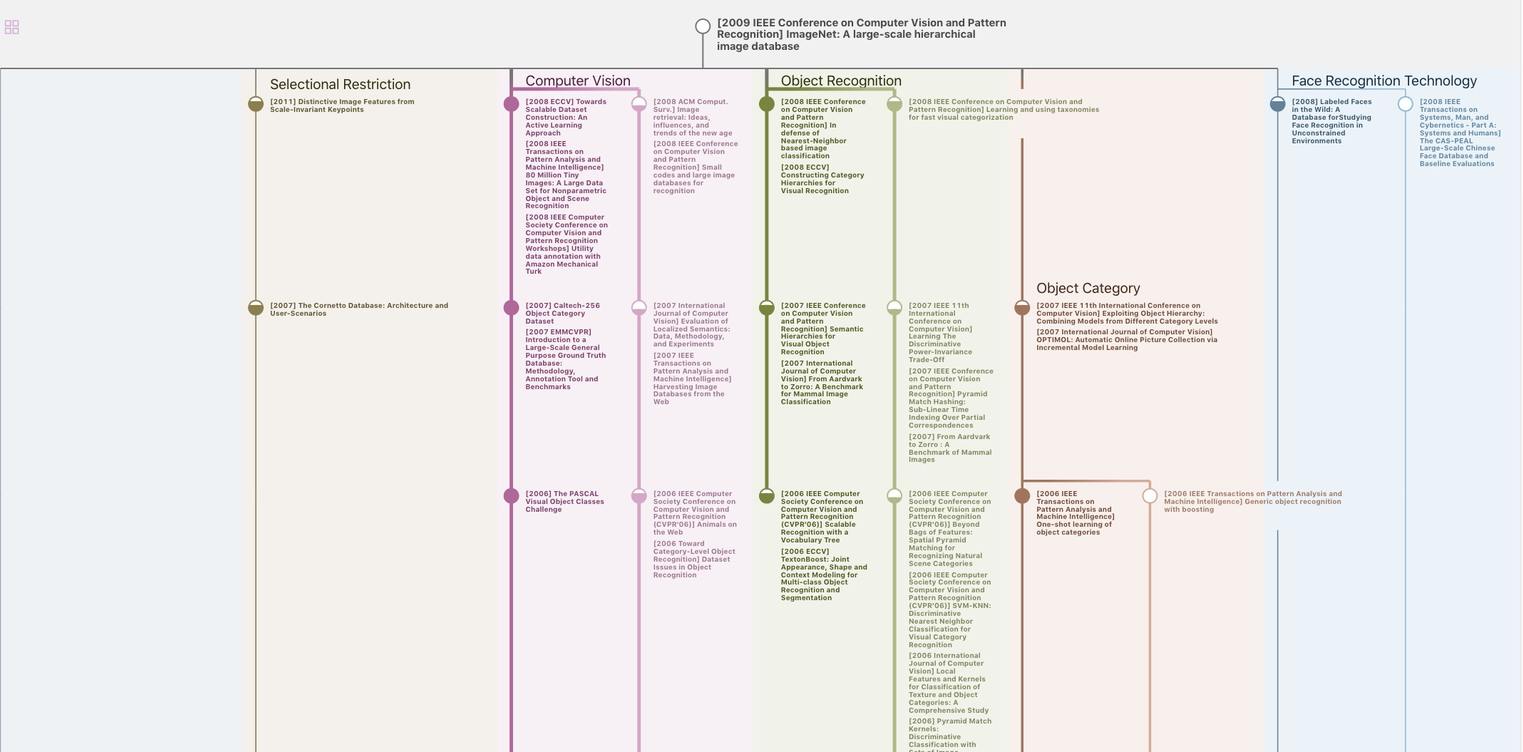
生成溯源树,研究论文发展脉络
Chat Paper
正在生成论文摘要