The factor graph network model for biological systems
RESEARCH IN COMPUTATIONAL MOLECULAR BIOLOGY, PROCEEDINGS(2005)
摘要
We introduce an extended computational framework for studying biological systems. Our approach combines formalization of existing qualitative models that are in wide but informal use today, with probabilistic modeling and integration of high throughput experimental data. Using our methods, it is possible to interpret genomewide measurements in the context of prior knowledge on the system, to assign statistical meaning to the accuracy of such knowledge and to learn refined models with improved fit to the experiments. Our model is represented as a probabilistic factor graph and the framework accommodates partial measurements of diverse biological elements. We develop methods for inference and learning in the model. We compare the performance of standard inference algorithms and tailor-made ones and show that hidden variables can be reliably inferred even in the presence of feedback loops and complex logic. We develop a formulation for the learning problem in our model which is based on deterministic hypothesis testing, and show how to derive p-values for learned model features. We test our methodology and algorithms on both simulated and real yeast data. In particular, we use our method to study the response of S. cerevisiae to hyper-osmotic shock, and explore uncharacterized logical relations between important regulators in the system.
更多查看译文
关键词
extended computational framework,probabilistic factor graph,diverse biological element,high throughput experimental data,model feature,informal use,biological system,refined model,prior knowledge,qualitative model,factor graph network model,factor graph,network model,probabilistic model,feedback loop,biological systems,high throughput,hidden variables,hypothesis test
AI 理解论文
溯源树
样例
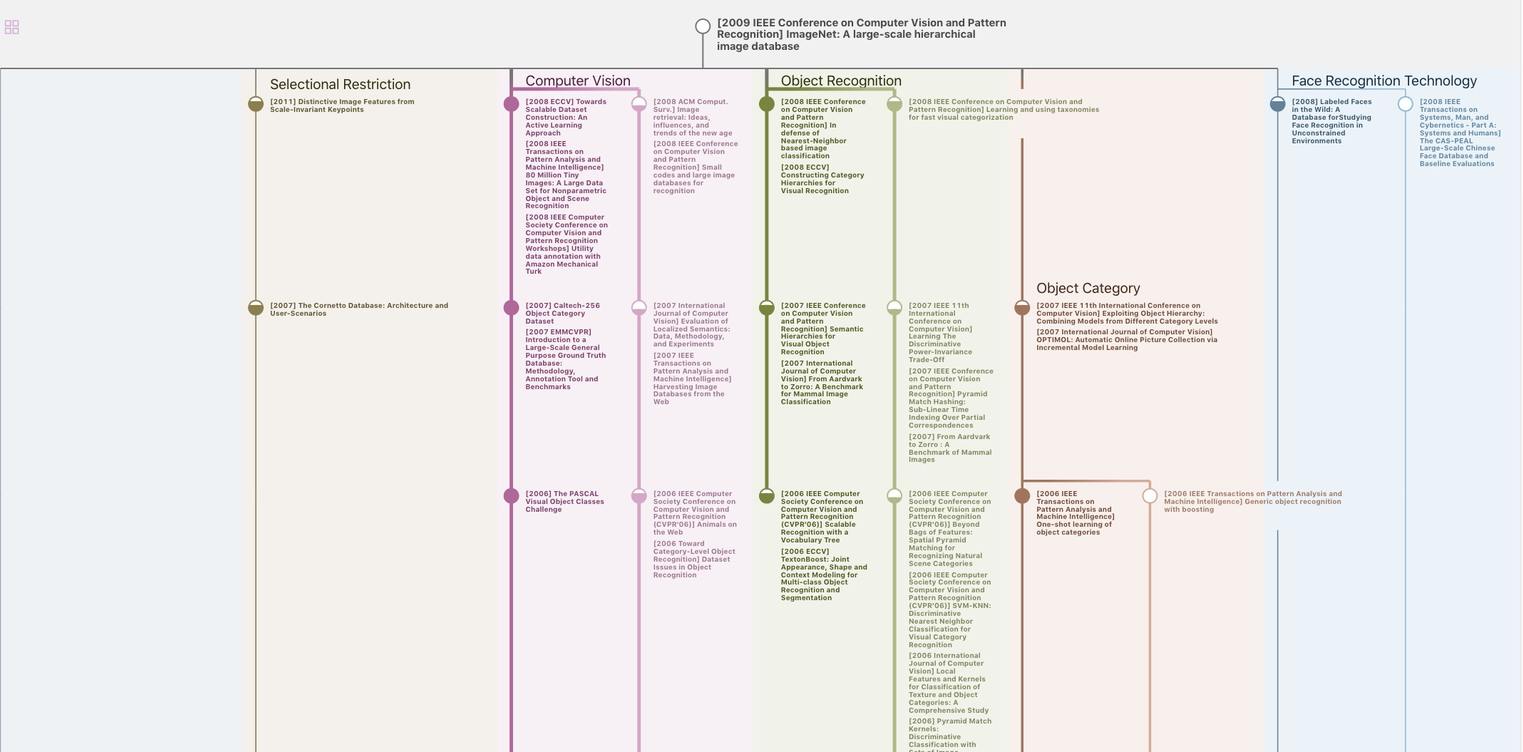
生成溯源树,研究论文发展脉络
Chat Paper
正在生成论文摘要