Contextual slip and prediction of student performance after use of an intelligent tutor
USER MODELING, ADAPTATION, AND PERSONALIZATION, PROCEEDINGS(2010)
摘要
Intelligent tutoring systems that utilize Bayesian Knowledge Tracing have achieved the ability to accurately predict student performance not only within the intelligent tutoring system, but on paper post-tests outside of the system Recent work has suggested that contextual estimation of student guessing and slipping leads to better prediction within the tutoring software (Baker, Corbett, & Aleven, 2008a, 2008b) However, it is not yet clear whether this new variant on knowledge tracing is effective at predicting the latent student knowledge that leads to successful post-test performance In this paper, we compare the Contextual-Guess-and-Slip variant on Bayesian Knowledge Tracing to classical four-parameter Bayesian Knowledge Tracing and the Individual Difference Weights variant of Bayesian Knowledge Tracing (Corbett & Anderson, 1995), investigating how well each model variant predicts post-test performance We also test other ways to utilize contextual estimation of slipping within the tutor in post-test prediction, and discuss hypotheses for why slipping during tutor use is a significant predictor of post-test performance, even after Bayesian Knowledge Tracing estimates are controlled for.
更多查看译文
关键词
Student Modeling, Bayesian Knowledge Tracing, Intelligent Tutoring Systems, Educational Data Mining, Contextual Slip
AI 理解论文
溯源树
样例
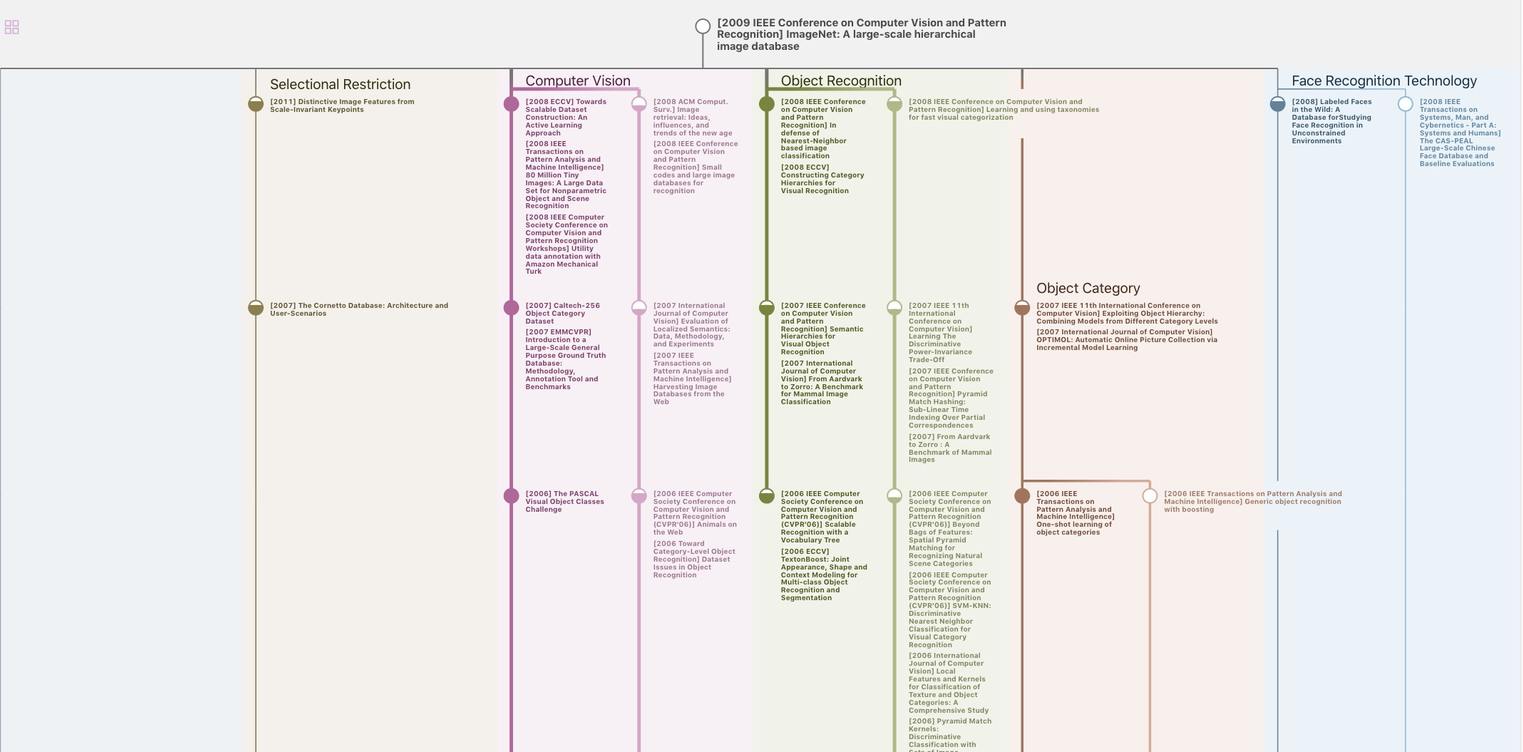
生成溯源树,研究论文发展脉络
Chat Paper
正在生成论文摘要