Saliency from hierarchical adaptation through decorrelation and variance normalization
Image and Vision Computing(2012)
摘要
This paper presents a novel approach to visual saliency that relies on a contextually adapted representation produced through adaptive whitening of color and scale features. Unlike previous models, the proposal is grounded on the specific adaptation of the basis of low level features to the statistical structure of the image. Adaptation is achieved through decorrelation and contrast normalization in several steps in a hierarchical approach, in compliance with coarse features described in biological visual systems. Saliency is simply computed as the square of the vector norm in the resulting representation. The performance of the model is compared with several state-of-the-art approaches, in predicting human fixations using three different eye-tracking datasets. Referring this measure to the performance of human priority maps, the model proves to be the only one able to keep the same behavior through different datasets, showing free of biases. Moreover, it is able to predict a wide set of relevant psychophysical observations, to our knowledge, not reproduced together by any other model before.
更多查看译文
关键词
novel approach,previous model,hierarchical adaptation,different eye-tracking datasets,different datasets,human priority map,variance normalization,specific adaptation,hierarchical approach,human fixation,biological visual system,resulting representation,bottom up,decorrelation,saliency
AI 理解论文
溯源树
样例
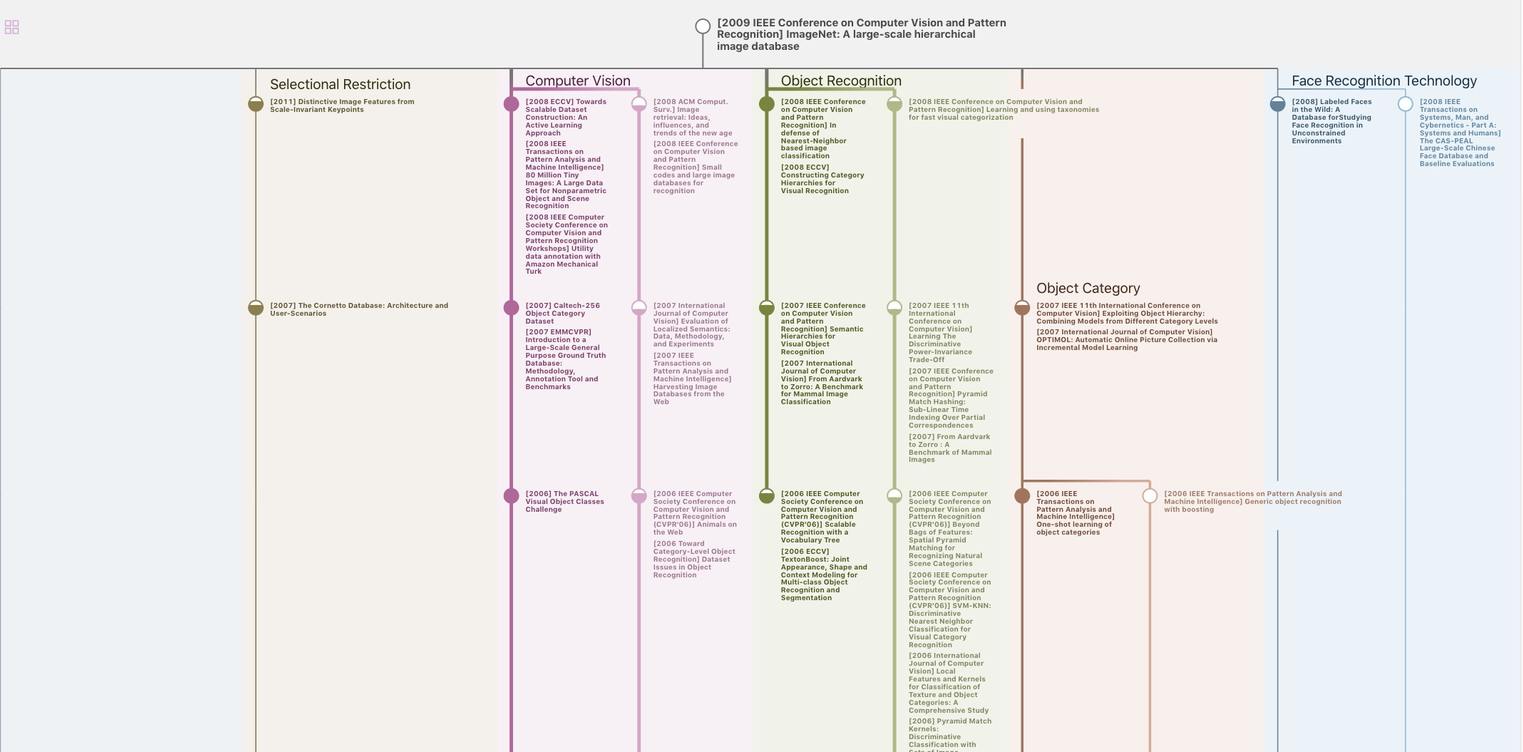
生成溯源树,研究论文发展脉络
Chat Paper
正在生成论文摘要