Resilient Identity Crime Detection
IEEE Transactions on Knowledge and Data Engineering(2012)
摘要
Identity crime is well known, prevalent, and costly; and credit application fraud is a specific case of identity crime. The existing nondata mining detection system of business rules and scorecards, and known fraud matching have limitations. To address these limitations and combat identity crime in real time, this paper proposes a new multilayered detection system complemented with two additional layers: communal detection (CD) and spike detection (SD). CD finds real social relationships to reduce the suspicion score, and is tamper resistant to synthetic social relationships. It is the whitelist-oriented approach on a fixed set of attributes. SD finds spikes in duplicates to increase the suspicion score, and is probe-resistant for attributes. It is the attribute-oriented approach on a variable-size set of attributes. Together, CD and SD can detect more types of attacks, better account for changing legal behavior, and remove the redundant attributes. Experiments were carried out on CD and SD with several million real credit applications. Results on the data support the hypothesis that successful credit application fraud patterns are sudden and exhibit sharp spikes in duplicates. Although this research is specific to credit application fraud detection, the concept of resilience, together with adaptivity and quality data discussed in the paper, are general to the design, implementation, and evaluation of all detection systems.
更多查看译文
关键词
computer crime,data mining,fraud,business rules,communal detection,credit application fraud detection,fraud matching,legal behavior,multilayered detection system,nondata mining detection system,resilient identity crime detection,spike detection,synthetic social relationships,whitelist-oriented approach,Data mining-based fraud detection,anomaly detection.,data stream mining,security
AI 理解论文
溯源树
样例
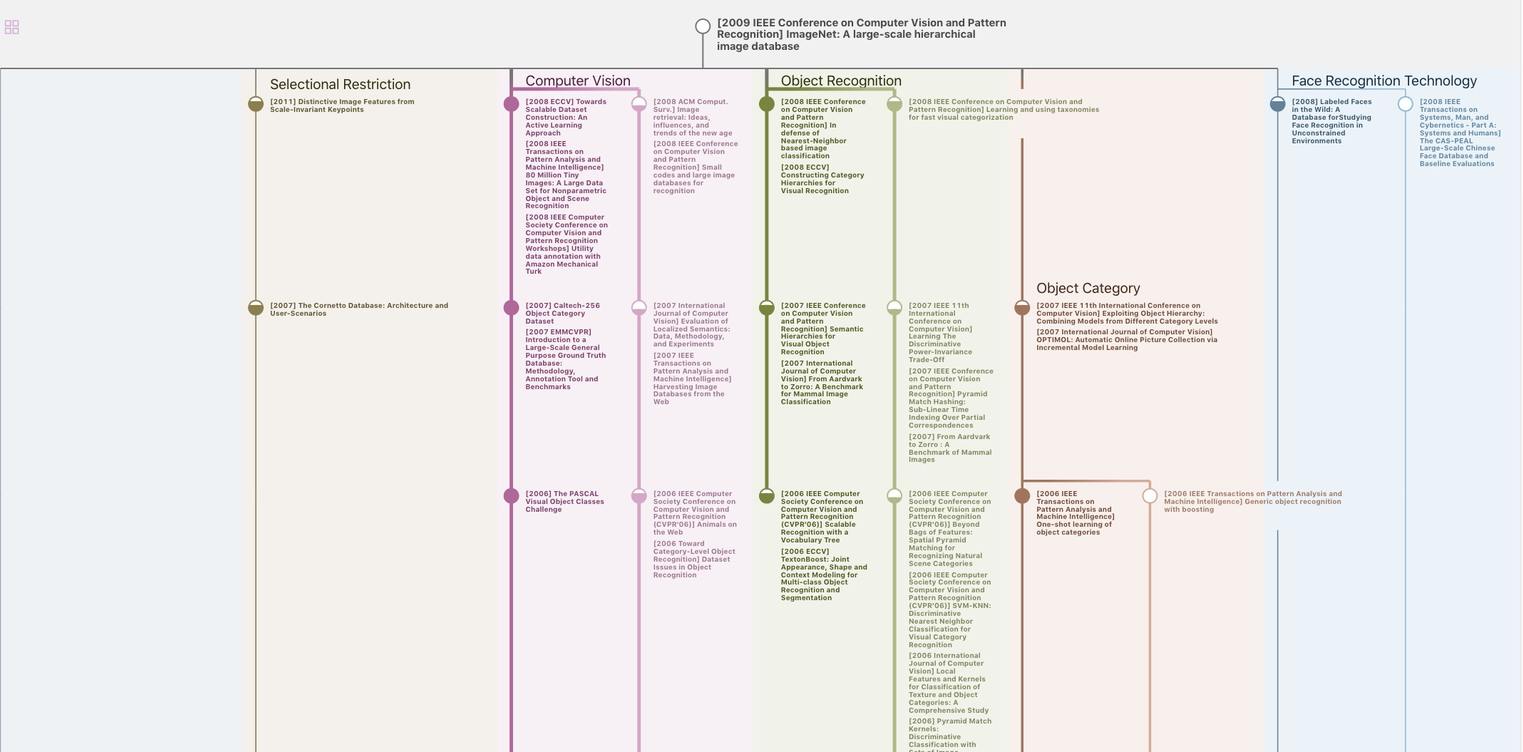
生成溯源树,研究论文发展脉络
Chat Paper
正在生成论文摘要