A machine learning approach to modeling power and performance of chip multiprocessors
Computer Design(2011)
摘要
Exploring the vast microarchitectural design space of chip multiprocessors (CMPs) through the traditional approach of exhaustive simulations is impractical due to the long simulation times and its super-linear increase with core scaling. Kernel based statistical machine learning algorithms can potentially help predict multiple performance metrics with non-linear dependence on the CMP design parameters. In this paper, we describe and evaluate a machine learning framework that uses Kernel Canonical Correlation Analysis (KCCA) to predict the power dissipation and performance of CMPs. Specifically we focus on modeling the microarchitecture of a highly multithreaded CMP targeted towards packet processing. We use a cycle accurate CMP simulator to generate training samples required to build the model. Despite sampling only 0.016% of the design space we observe a median error of 6-10% in the KCCA predicted processor power dissipation and performance.
更多查看译文
关键词
chip multiprocessor,microarchitectural design space,design space,cmp design parameter,kernel canonical correlation analysis,microprocessor chips,cycle accurate cmp simulator,power dissipation,kernel based statistical machine learning,learning (artificial intelligence),statistical machine,architecture,multithreaded cmp,multiprocessing systems,vast microarchitectural design space,processor power dissipation,kcca,computer architecture,chip multiprocessors,power,cpi,machine learning,modeling,multiple performance metrics,packet processing,electronic engineering computing,instruction sets,predictive models,measurement,learning artificial intelligence,vectors,microarchitecture,kernel
AI 理解论文
溯源树
样例
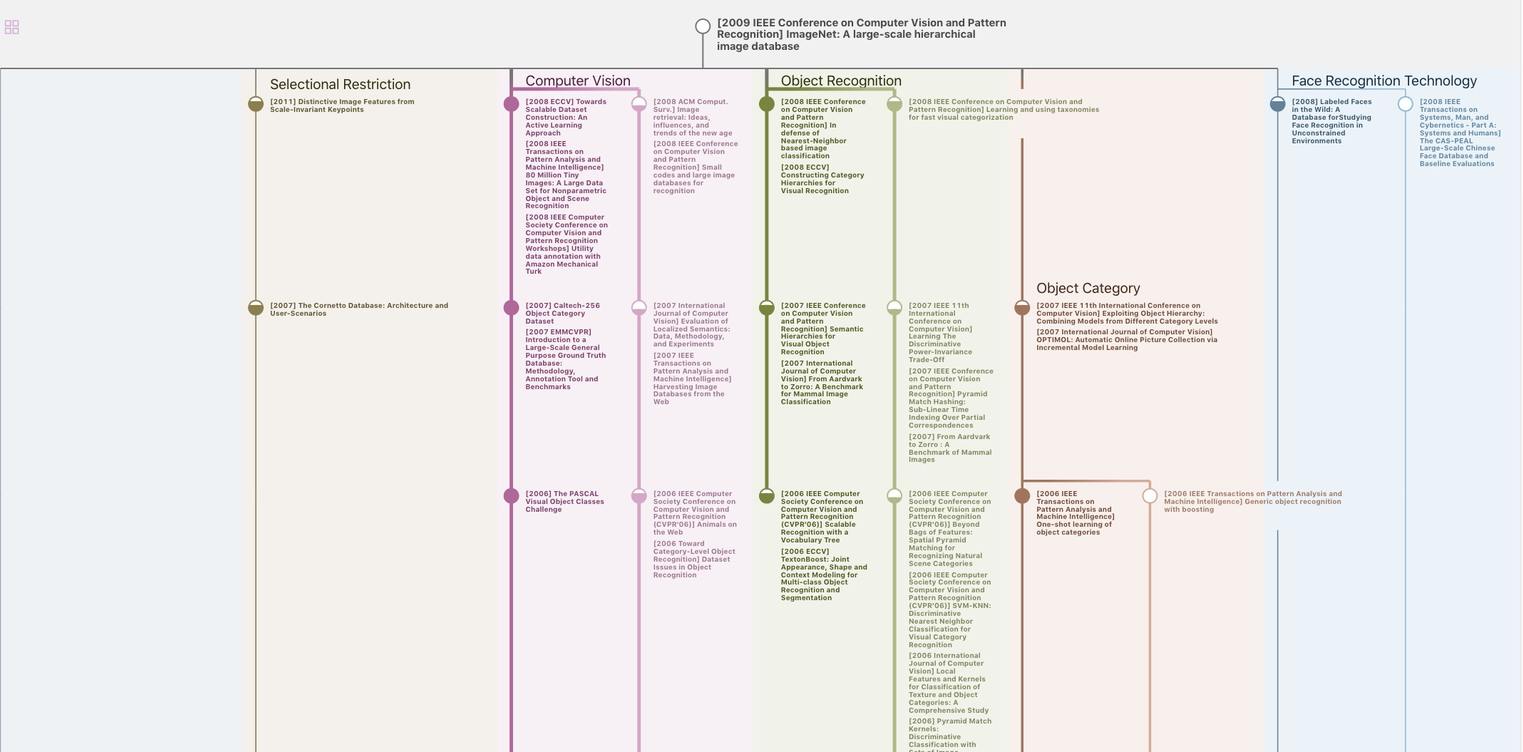
生成溯源树,研究论文发展脉络
Chat Paper
正在生成论文摘要