Understanding Propagation Error and Its Effect on Collective Classification
Data Mining(2011)
摘要
Recent empirical evaluation has shown that the performance of collective classification models can vary based on the amount of class label information available for use during inference. In this paper, we further demonstrate that the relative performance of statistical relational models learned with different estimation methods changes as the availability of test set labels increases. We reason about the cause of this phenomenon from an information-theoretic perspective and this points to a previously unidentified consideration in the development of relational learning algorithms. In particular, we characterize the high propagation error of collective inference models that are estimated with maximum pseudolikelihood estimation (MPLE), and show how this affects performance across the spectrum of label availability when compared to MLE, which has low propagation error. Our formal study leads to a quantitative characterization that can be used to predict the confidence of local propagation for MPLE models. We use this to propose a mixture model that can learn the best trade-off between high and low propagation models. Empirical evaluation on synthetic and real-world data show that our proposed method achieves comparable, or superior, results to both MPLE and low propagation models across the full spectrum of label availability.
更多查看译文
关键词
class label information,low propagation error,relative performance,mple model,low propagation model,collective inference model,understanding propagation error,collective classification,collective classification model,local propagation,high propagation error,label availability,learning artificial intelligence,spectrum,information theory,relational learning,relational model,mixture model,maximum likelihood estimation,statistical relational learning
AI 理解论文
溯源树
样例
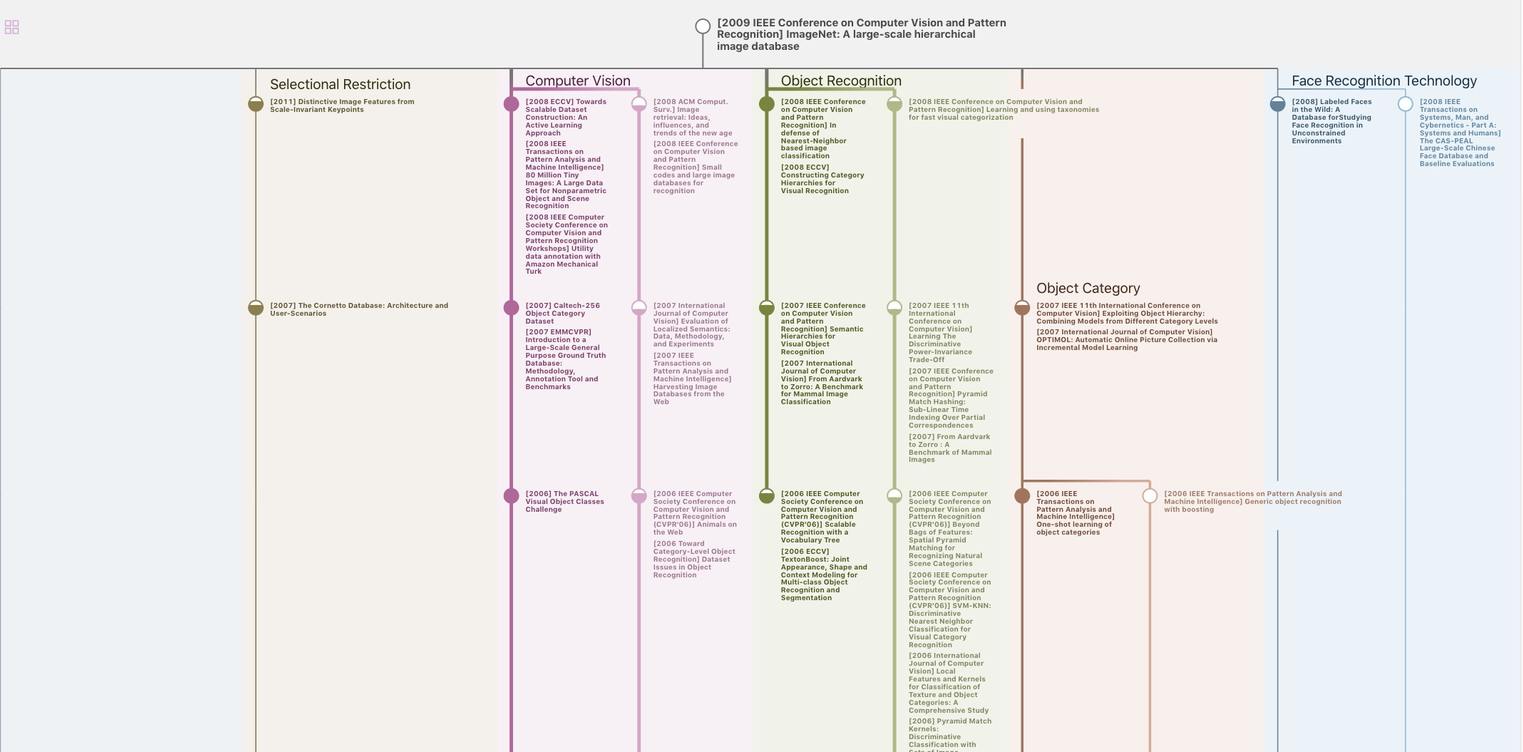
生成溯源树,研究论文发展脉络
Chat Paper
正在生成论文摘要