ACME: an associative classifier based on maximum entropy principle
ALGORITHMIC LEARNING THEORY(2005)
摘要
Recent studies in classification have proposed ways of exploiting the association rule mining paradigm. These studies have performed extensive experiments to show their techniques to be both efficient and accurate. However, existing studies in this paradigm either do not provide any theoretical justification behind their approaches or assume independence between some parameters. In this work, we propose a new classifier based on association rule mining. Our classifier rests on the maximum entropy principle for its statistical basis and does not assume any independence not inferred from the given dataset. We use the classical generalized iterative scaling algorithm (GIS) to create our classification model. We show that GIS fails in some cases when itemsets are used as features and provide modifications to rectify this problem. We show that this modified GIS runs much faster than the original GIS. We also describe techniques to make GIS tractable for large feature spaces – we provide a new technique to divide a feature space into independent clusters each of which can be handled separately. Our experimental results show that our classifier is generally more accurate than the existing classification methods.
更多查看译文
关键词
associative classifier,maximum entropy principle,classification model,association rule mining,gis tractable,original gis,modified gis,large feature space,existing classification method,feature space,association rule mining paradigm,new classifier,generalized iterative scaling
AI 理解论文
溯源树
样例
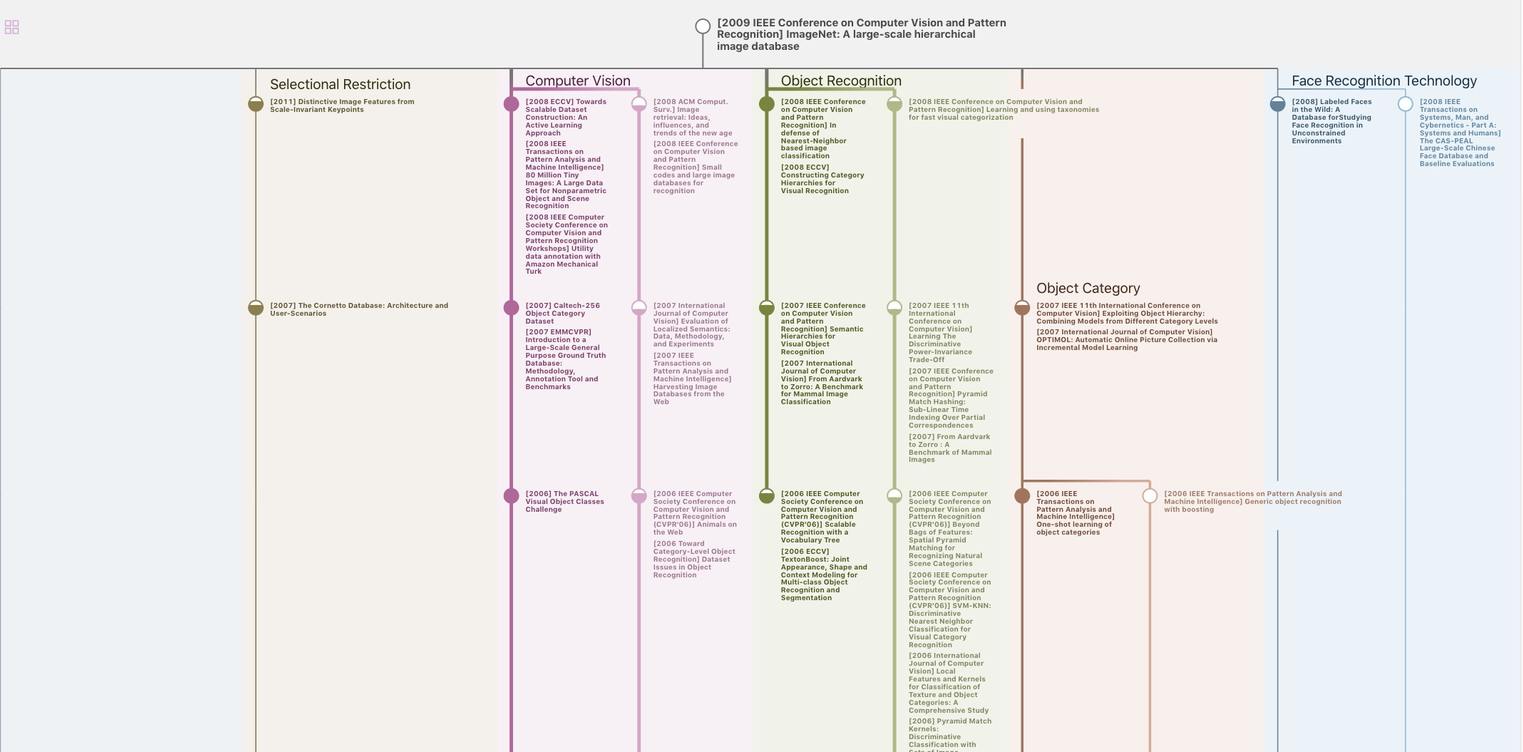
生成溯源树,研究论文发展脉络
Chat Paper
正在生成论文摘要