Evaluating semantic evaluations: how RTE measures up
MACHINE LEARNING CHALLENGES: EVALUATING PREDICTIVE UNCERTAINTY VISUAL OBJECT CLASSIFICATION AND RECOGNIZING TEXTUAL ENTAILMENT(2005)
摘要
In this paper, we discuss paradigms for evaluating open-domain semantic interpretation as they apply to the PASCAL Recognizing Textual Entailment (RTE) evaluation (Dagan et al. 2005). We focus on three aspects critical to a successful evaluation: creation of large quantities of reasonably good training data, analysis of inter-annotator agreement, and joint analysis of test item difficulty and test-taker proficiency (Rasch analysis). We found that although RTE does not correspond to a “real” or naturally occurring language processing task, it nonetheless provides clear and simple metrics, a tolerable cost of corpus development, good annotator reliability (with the potential to exploit the remaining variability), and the possibility of finding noisy but plentiful training material.
更多查看译文
关键词
language processing task,good annotator reliability,rasch analysis,pascal recognizing textual entailment,corpus development,successful evaluation,plentiful training material,joint analysis,good training data,semantic evaluation,inter-annotator agreement,semantic interpretation,data analysis
AI 理解论文
溯源树
样例
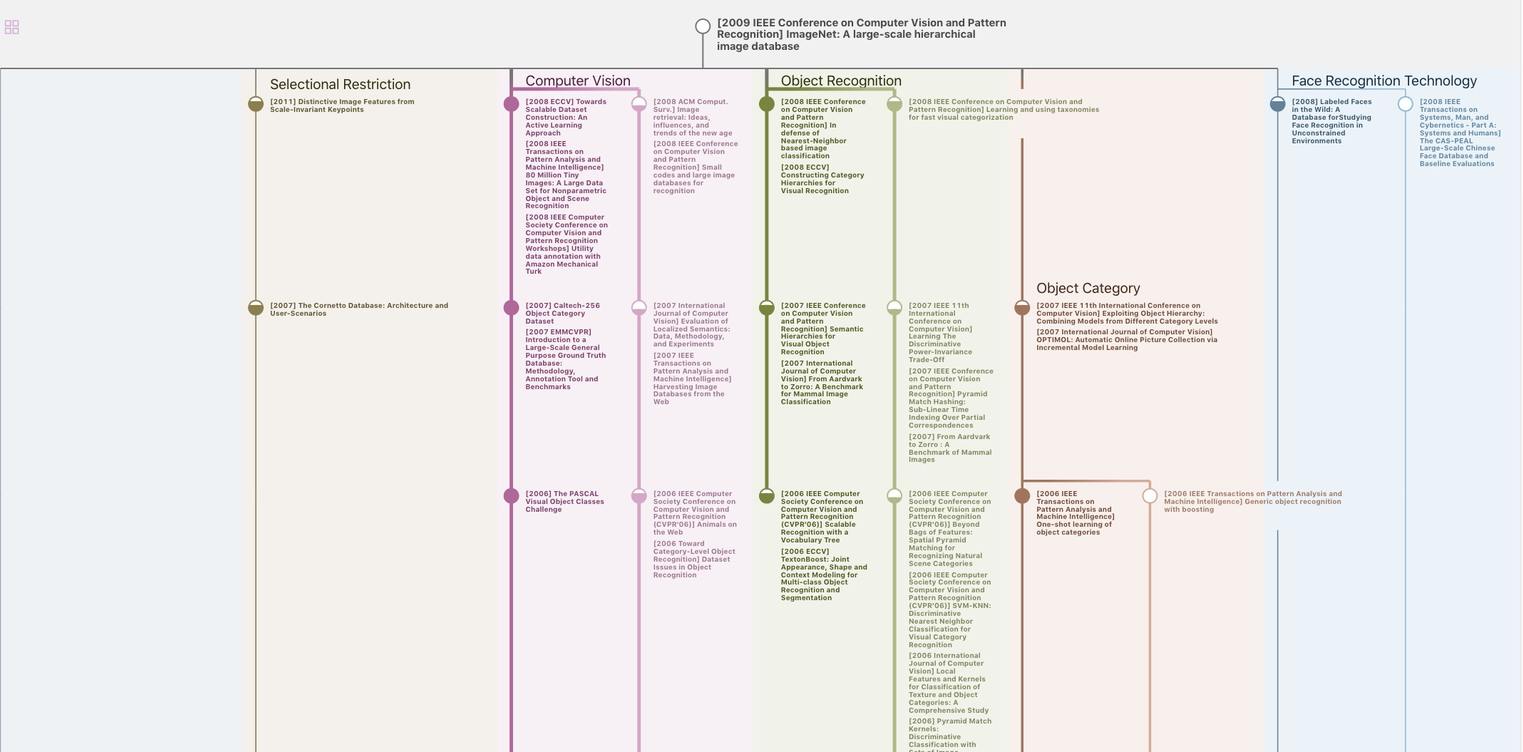
生成溯源树,研究论文发展脉络
Chat Paper
正在生成论文摘要