Classification-based self-adaptive differential evolution with fast and reliable convergence performance
Soft Computing(2011)
摘要
To avoid the problems of slow and premature convergence of the differential evolution (DE) algorithm, this paper presents a new DE variant named p-ADE. It improves the convergence performance by implementing a new mutation strategy “DE/rand-to-best/pbest”, together with a classification mechanism, and controlling the parameters in a dynamic adaptive manner, where the “DE/rand-to-best/pbest” utilizes the current best solution together with the best previous solution of each individual to guide the search direction. The classification mechanism helps to balance the exploration and exploitation of individuals with different fitness characteristics, thus improving the convergence rate. Dynamic self-adaptation is beneficial for controlling the extent of variation for each individual. Also, it avoids the requirement for prior knowledge about parameter settings. Experimental results confirm the superiority of p-ADE over several existing DE variants as well as other significant evolutionary optimizers.
更多查看译文
关键词
Differential evolution (DE),Evolutionary algorithms,Mutation strategy,Classification,Parameter adaptation
AI 理解论文
溯源树
样例
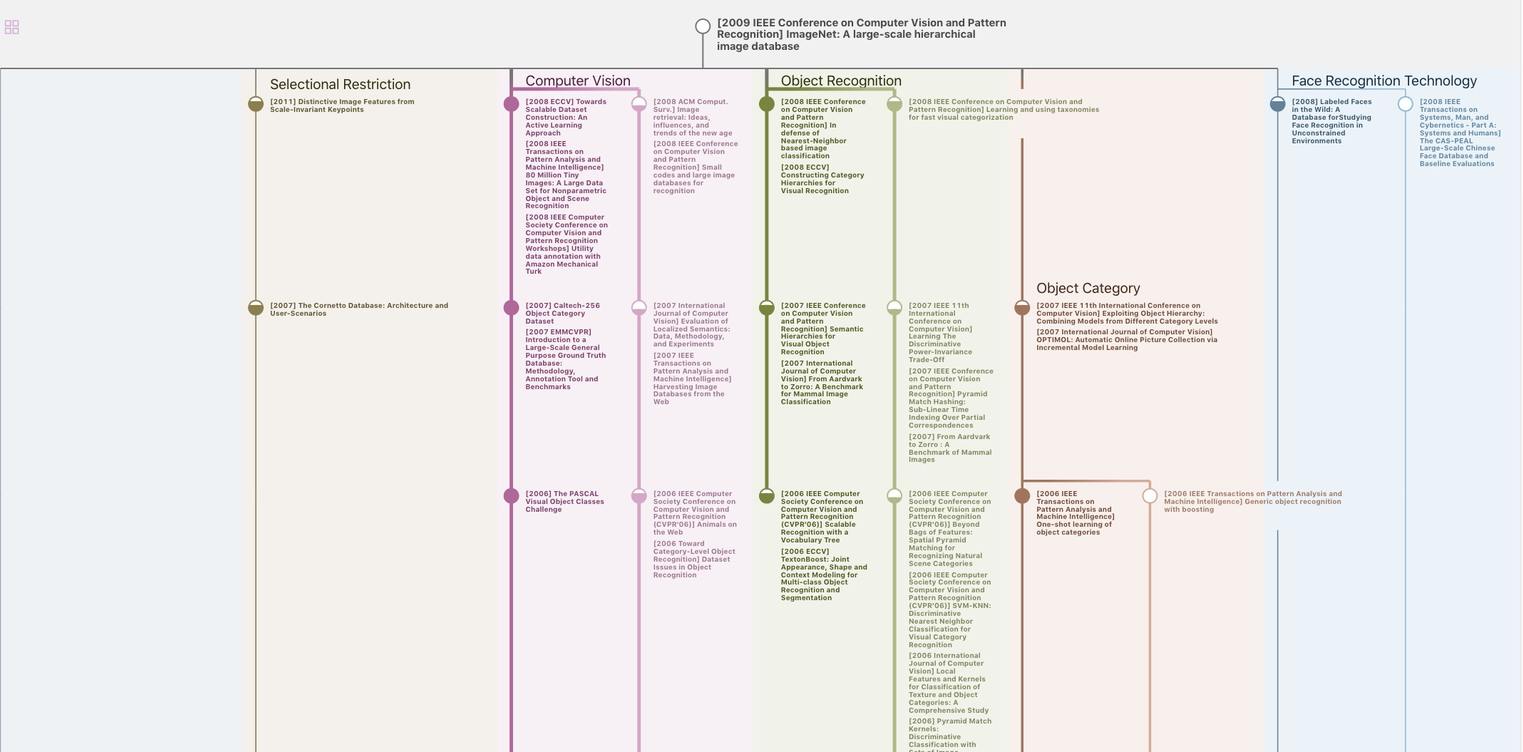
生成溯源树,研究论文发展脉络
Chat Paper
正在生成论文摘要