Epileptic Seizure Detection for Multichannel EEG Signals with Support Vector Machines
Bioinformatics and Bioengineering(2011)
摘要
Epilepsy is a common chronic neurological disorder characterized by recurrent unprovoked seizures. The electroencephalogram (EEG) signals play an important role in the diagnosis of epilepsy. In addition, multi-channel EEG signals have much more discrimination information than a single channel. However, traditional recognition algorithms of EEG signals are lack of multichannel EEG signals. In this paper, we propose a new method of epileptic seizure detection based on multichannel EEG signals. Both unipolar and bipolar EEG signals are considered in our approach. We make use of approximate entropy (ApEn) and statistic values to extract features. Furthermore, we tested the performance of four different Support Vector Machines (SVMs). The results reveal that the grid SVM achieves the highest totally classification accuracy (98.91%).
更多查看译文
关键词
multi-channel eeg signal,recognition algorithms,medical disorders,common chronic neurological disorder,medical signal detection,multichannel eeg signals,neurophysiology,eeg signal,bipolar eeg signal,grid computing,electroencephalogram,statistical analysis,electroencephalography,recurrent unprovoked seizures,multichannel eeg signal,medical signal processing,different support,grid method,chronic neurological disorder,electroencephalogram signals,epileptic seizure detection,feature extraction,vector machines,signal classification,statistic values,approximate entropy,classification accuracy,entropy,discrimination information,support vector machines,unipolar eeg signal,accuracy,bioinformatics,support vector machine
AI 理解论文
溯源树
样例
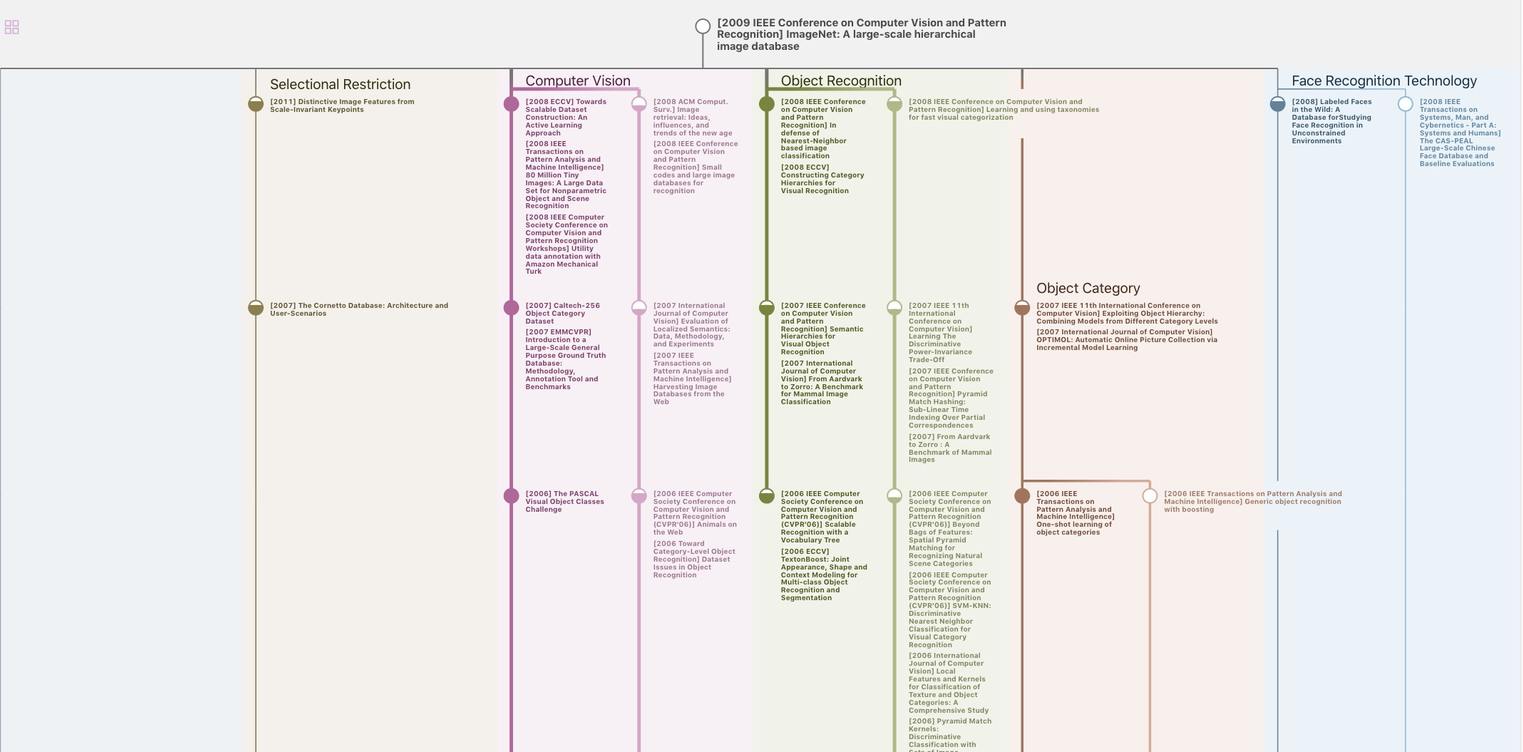
生成溯源树,研究论文发展脉络
Chat Paper
正在生成论文摘要