High-Dimensional Fuzzy Inference Systems
IEEE TRANSACTIONS ON SYSTEMS MAN CYBERNETICS-SYSTEMS(2024)
摘要
FIS have been developed for many years but the use of fuzzy inference systems (FISs) for high-dimensional problems is still a challenging task. The most frequently used T-norms for computing the firing strengths are product and minimum operators of which the former is often preferred because of its differentiability. However, for high-dimensional problems, the product T-norm suffers from the numeric underflow problem. Here, we primarily focus on addressing the problem that is associated with the use of the T-norms for designing high-dimensional FISs (HDFISs). For the product T-norm, we construct an HDFIS named HDFIS-prod, which easily escapes from the numeric underflow problem. The main novelty is that we propose an adaptive dimension-dependent membership function (DMF). For the minimum T-norm, an empirical observation led us to develop a mechanism that has the natural ability to deal with super high-dimensional problems, which results in another HDFIS named HDFIS-min. Both HDFIS-prod and HDFIS-min are tested on 18 datasets with feature dimensions varying from 1024 to 120 450. The simulation results demonstrate that both of them have competitive performance on handling high-dimensional datasets.
更多查看译文
关键词
Fuzzy inference systems (FISs),high-dimensional problems,numeric underflow,Takagi-Sugeno-Kang (TSK),T-norm
AI 理解论文
溯源树
样例
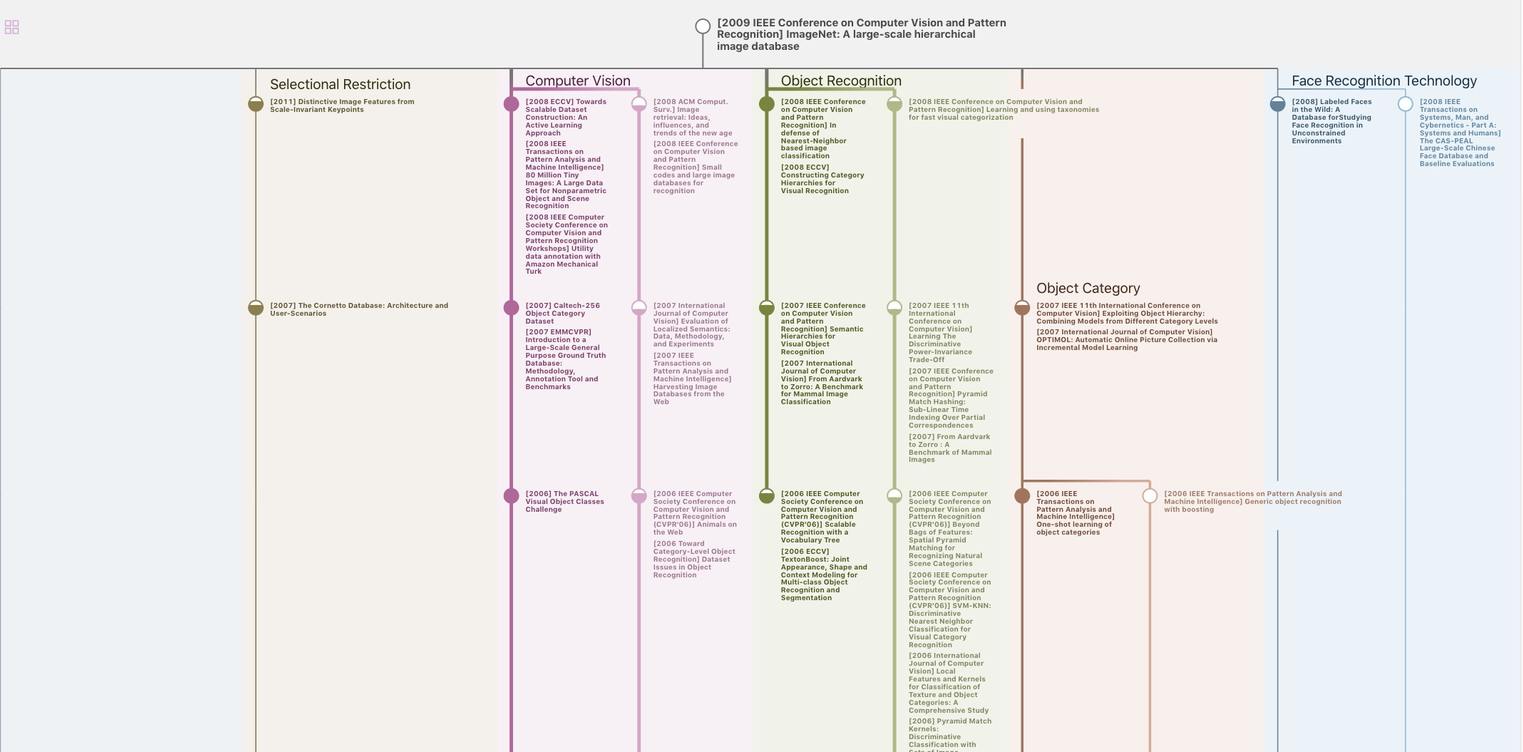
生成溯源树,研究论文发展脉络
Chat Paper
正在生成论文摘要