Anomaly detection in information streams without prior domain knowledge
IBM Journal of Research and Development(2011)
摘要
A key goal of information analytics is to identify patterns of anomalous behavior. Such identification of anomalies is required in a variety of applications such as systems management, sensor networks, and security. However, most of the current state of the art on anomaly detection relies on using a predefined knowledge base. This knowledge base may consist of a predefined set of policies and rules, a set of templates representing predefined patterns in the data, or a description of events that constitutes anomalous behavior. When used in practice, a significant limitation of information analytics is the effort that goes into defining and creating the predefined knowledge base and the need to have prior information about the domain. In this paper, we present an approach that can identify anomalies in the information stream without requiring any prior domain knowledge. The proposed approach simultaneously monitors and analyzes the data stream at multiple temporal scales and learns the evolution of normal behavior over time in each time scale. The proposed approach is not sensitive to the choice of the distance metric and hence is applicable in various domains and applications. We have studied the effectiveness of the approach using different data sets.
更多查看译文
关键词
anomaly detection,prior domain knowledge,information analytics,information stream,knowledge base,anomalous behavior,predefined pattern,predefined knowledge base,prior information,predefined set
AI 理解论文
溯源树
样例
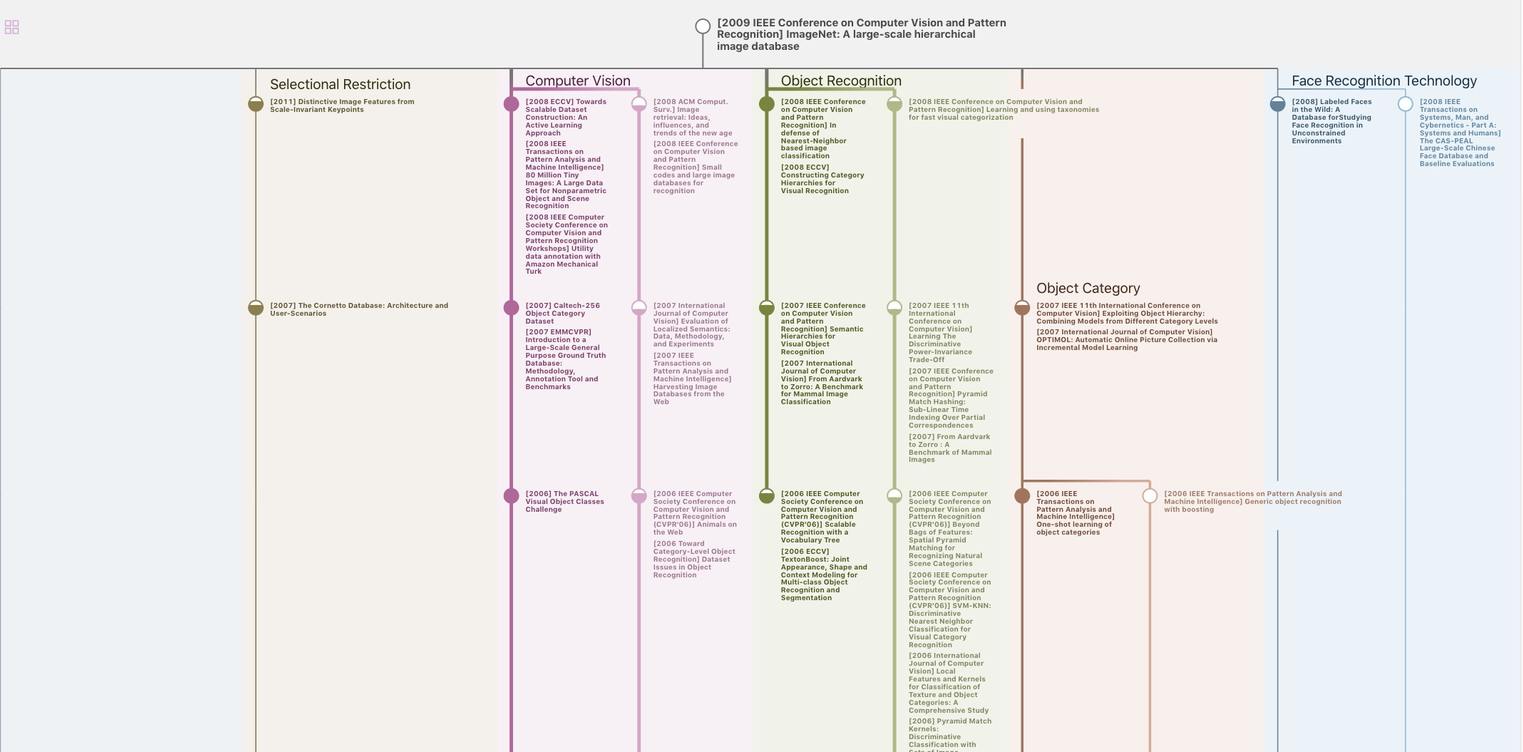
生成溯源树,研究论文发展脉络
Chat Paper
正在生成论文摘要